All published articles of this journal are available on ScienceDirect.
A Comprehensive Study of Single and Multiple Truck Crashes Using Violation and Crash Data
Abstract
Around 4,000 people died in crashes involving trucks in 2016 alone in the U.S., with 21 percent of these fatalities involving only single-unit trucks. Many studies have identified the underlying factors for truck crashes. However, few studies detected the factors unique to single and multiple crashes, and none have examined these underlying factors in conjunction with violation data. The current research assessed all of these factors using two approaches to improve truck safety. An injury/fatal crash was defined as a crash that results in an injury or fatality. The first approach investigated the contributory factors that increased the odds of injury/fatal single truck and multiple vehicle crashes with involvement of at least one truck. The literature has indicated that previous violations can be used to predict future violations and crashes. Therefore, the second approach used violations related to driver actions that could result in truck crashes. The analysis for the first approach indicated that driving on dry-roadway surfaces, driver distraction, and rollover/jackknife types of truck crashes, speed compliance failure, and higher posted speed limits are some of the factors that increased the odds of injury/fatal single and multiple vehicle crashes. With the second approach, the violations related to risky driver actions, which were underlying causes of truck crashes, were identified and analyses were run to identify the groups at increased risk of truck involved crashes. The results of violations indicated that being nonresident, driving off peak hours, and driving on weekends could increase the risk of truck involved crashes.
1. INTRODUCTION
Trucks are a crucial part of the United States economy. Trucks transport 80% of all freight in the U.S. annually, which accounts for over $700 billion worth of goods [1]. The trucking industry in the U.S. moves about 10.5 billion tons annually, which is expected to increase to 27 billion tons by 2040 [2]. Moreover, seven million people, including more than three million drivers, are employed through this industry. However, truck crashes place a huge burden on the nation in terms of death, injury, and lost productivity. According to the Federal Motor Carrier Safety Administration (FMCSA), there were 667 truck occupant deaths (driver and passenger), and of those 667 deaths, 398 deaths occurred in single-vehicle crashes [3].
Wyoming has the highest fatality rate (24.7 death per 100,000 population) in the nation [4]. Wyoming also has the highest truck crash rate in the United States [5]. These high truck crash and fatality rates result from the high amount of through truck traffic on Wyoming interstates, adverse weather conditions, and mountainous geometric conditions.
However, truck crashes can be mitigated by improving truck safety through policies and regulations, which enhance the performance of the trucking industry without compromising safety. Various countermeasures have been taken in the United States organized into 4 E’s of safety. The 4 E’s include enforcement, education, engineering, and emergency response. Enforcement is one of the 4 E’s that can improve traffic safety. The performance of Wyoming highway patrol (WHP), and consequently road safety, could be improved by identification of the factors that increase the odds of future violations, and consequently future crashes [6, 7]. Thus, this study incorporates violation data, in addition to crash data, to identify the contributory factors to the violations that are likely to increase the odds of future crashes. Identification of these factors can help the WHP to put more emphasis on the contributory factors of risky violations resulting in traffic safety.
Truck crashes are complex events. They can involve single vehicles or two or more vehicles. Out of 700 truck occupant deaths that occur every year in the U.S., about 60% occur in single-vehicle truck crashes [8]. For each type of event, different contributory factors may play roles. Previous research indicated that there are significant differences between single and multiple vehicle crashes [9, 10]. Therefore, this study analyzed single truck and multiple vehicle crashes, with truck involvement, separately. This study investigated factors impacting different types of truck crashes by including vehicle, driver, and environmental factors. In addition, this study included violation data to identify the groups at higher risk of truck crashes by including only the violations contributing to truck crashes in this state. For the purpose of this study, a truck is defined as a commercial vehicle with gross vehicle weight rating greater than 10,000 pounds.
2. BACKGROUND
Based on FMCSA, the critical reasons for large truck crashes can be assigned to driver (87%), non-performance (12%), recognition (28%), decision (38%), performance (9%), and vehicle (10%) [11]. Lemp et al. (2011) used the ordered probit model to investigate the impact of vehicle, occupant, driver, and environmental characteristics on crash severity for those involved in heavy-duty truck crashes [12]. The results indicated that the odds of fatalities increase with the number of trailers and fall as the truck gross vehicle weight rating decreases. Khattak et al. (2003) used crash data in North Carolina during 1996-1998 to investigate the impact of truck rollovers and occupant injuries in single-vehicle crashes [8]. The results indicated that higher risk factors in single-truck-crashes include risky driving, speeding, alcohol and drug use, traffic control violations, truck exposure to dangerous road geometry, and trucks that transport hazardous materials.
Moomen et al. (2018) investigated the influential factors of downgrade truck crashes in Wyoming using logistics regression. They found that driver gender, speed compliance, weather, lighting and road condition, shoulder and lane width, number of sag and crest curves, roadway grade and length are the contributory factors to truck related crashes [13]. Schneider et al. (2009) developed multinomial logit models to investigate driver injury severity resulting from single-vehicle crashes [14]. Different driver, vehicle, and environmental characteristics were found to increase injury severity. Being female, older, unbuckled, fatigued, and under the influence led to increase in the odds of injury. Zhu and Srinivasan (2011) investigated the factors impacting the injury severity in truck crashes [15]. Truck driver distraction, alcohol use, and emotional factors of car drivers were associated with higher severity crashes. Pahukula et al. (2015) investigated the contributory factors to injury severity of truck crashes using data from Texas during 2006 to 2010 [16]. The results indicated that different time periods in a day have different contributing impacts on truck crash severity.
However, in the majority of the studies, researchers mostly looked at the injury severity of both multiple-vehicle and single truck crashes as a whole. Thus, they did not identify the variables unique to single and multiple crashes. Zou et al. (2017) carried out a study in New York City to investigate the differences between single-vehicle and multiple-vehicle truck crashes [17]. The results indicated that there are substantial differences between factors affecting single and multiple truck crashes. Thus, this study examined truck crash severity separately for single-vehicle and multiple-vehicle truck crashes.
Many studies have identified correlations between previous violations and future crash risk. A previous study carried out by Li and Baker (1994) indicated that conviction records can be used to identify groups with greater odds of involvement in fatal crashes [18]. Similarly, Elliott (2001) investigated the ability of previous violations to predict future offenses and crashes [19]. The results indicated that the drivers with previous ticketed offenses are at greater risk for future crashes. Rezapour et al. (2017) used violation data, in addition to crash data, to assess unsafe driver actions to reduce crashes [6]. Chen et al. (1995) carried out a study by examining driver records to investigate the relationship between crashes and past records of crashes and convictions [20]. The authors found a positive correlation between pre-period crashes per driver and pre-period number of convictions. In this study, failure to yield and disobeying traffic signals were two violations that best predict crashes. Lantz and Loftus (2006) carried out a study to use an analytical model for predicting future crash involvement based on the history of driver information and also identifying effective enforcement actions that can predict driver behavior and future crash involvement [21]. The results indicated that reckless driving and improper turn violations are the violations that have the highest increase in likelihood of a future crash. Also, failure to keep proper lane was some of the convictions with the highest likelihood of a future crash. A study by Terrill et al. (2016) investigated the impact of traffic citations on the number of crashes on an interstate in Wyoming [22]. The results indicated that an increased number of citations issued is a preventive measure for the number of crashes.
However, none of the aforementioned studies used violation or conviction data to investigate groups of truck drivers with an increased risk of being involved in truck crashes. On the basis of the discussed studies, violations can be used as an indication of the groups that are at greater risk of being involved in future crashes.
This current study was set forward to fulfill two main objectives:
- Conduct crash analysis to determine the factors impacting injury single truck and multiple vehicle, truck involved, crashes. In order to determine these factors, two analyses were carried out:
1.1 Injury/fatal single truck crash analysis.
1.2 Injury/fatal multiple vehicle crash analysis with involvement of at least one truck. - Conduct violations analysis to identify the groups who are more likely to violate the laws that are the main causes of single and multiple truck crashes. Two analyses were carried out to fulfill this objective:
2.1 Analysis of those types of violations associated with single truck crashes.
2.2 Analysis of those types of violations associated with multiple vehicle crashes involving at least one truck.
A crash in this study is one that results in an injury or fatality. Due to the low number of fatality crashes, these crashes were aggregated with injury crashes.
3. METHODS
Logistic regression is used in many studies involving binary crash outcomes [23-27]. For the logistic regression model, the binary response variable Y is assumed to have a Bernoulli distribution with probability π [28].
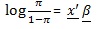
where x is a vector of explanatory variables and is a vector of unknown regression coefficients. Equation (1) can be solved for π which gives
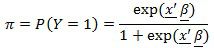
For modeling truck crash severity, the binary response variable was 1 for injury/fatal and 0 for a property damage only (PDO) truck involved crashes. The response is conditioned on a crash that has occurred, and then looking at its binary classification (fatal/injury (F+I) or PDO). Separate models were developed for single truck crashes and for multiple vehicle, truck involved, crashes. The probability (π) of either a single or multiple truck crash being injury was modeled using various risk factors as explanatory variables
.
Logistic regression was also used for analyzing violations. The purpose of these analyses was to identify drivers who are more at risk of committing particular traffic law violations, which can lead to truck involved crashes. Here, the response (Y) had the value 1 if a driver received a citation of a particular type and a 0 if a driver did not receive a citation of a particular type. The response is conditioned on drivers who had received a violation and then looking at its binary classification for the citation type (received or did not receive citation of particular type). Two different citation analyses were considered. The first analysis involved only those violations more commonly observed with single-truck crashes. The second analysis involved those violations most commonly observed in multiple-vehicle crashes involving at least one truck. The probability (π) of a driver receiving a citation of a particular type was examined in relation to the explanatory variables
involving driver characteristics such as gender and residency, and temporal characteristics such as time of day and day of the week.
Stepwise model selection was used to select explanatory variables for a final logistic regression model. A significance level of 0.10 was pre-specified for entering the model and a significance level of 0.05 was pre-specified for staying in the model. All analyses were performed using the Statistical Analysis System (SAS) [29].
4. DATA PREPARATION
The data was combined from the three interstates in Wyoming, I-80, I-25, and I-90, with the highest truck related crash rates. Crash data was obtained from the Wyoming Department of Transportation (WYDOT) using the Criticial Analysis Reporting Environment (CARE) from 2011 to 2014. This study used various variables, which can be categorized under driver, environmental, vehicle, temporal, crash, and driver behaviors. Driver characteristics included age, gender, residency, violation (conviction) record, and speed limit compliance at the time of crash. Environmental characteristics included weather and roadway-surface conditions. Weight of a truck was categorized as a vehicle characteristic. Day of week and time of crash were organized under temporal characteristics. Roadway characteristics included posted speed limit of a location where a crash occurred. Driver actions at the time of crash, number of vehicles, and pre-collision vehicle action were categorized under crash characteristics. Driver distraction, driver under influence (DUI) suspicion, fatigue, and the use of safety technology were categorized under driver behaviors. In this study, distraction is defined as any type of distraction such as TV, cell pager, or wireless communication inside the cabin at the time of crashes. Truck crash analyses were divided into two parts: Single truck and multiple vehicles, truck involved, crashes. Single truck crashes were investigated separately as more than 50% of all the truck crashes were single truck crashes.
The violation data was obtained from the Wyoming court reported violation database from 2011 to 2014. For single truck crash analysis, truck drivers were at fault in the crashes. Therefore, the violation data for this analysis was filtered to include just truck driver violations to identify groups that are more at risk of single truck crashes. There were 121,680 violations filtered to 17,239 truck violations. However, all violations were used for investigating the groups that were at higher risk of multiple vehicles crashes, involving at least one truck. This is due to the fact that both truck and no truck drivers could be at fault in these crashes. Only violation types: follow too closely, failure to drive within single lane, and speed too fast for conditions that resulted in truck crashes were included in this study (Table 1). Violations related to the main causes of truck crashes were identified among 800 types of violations and presented in Table 1. For instance, only one violation type: driving too fast for conditions was identified as a contributing factor to a crash type: “drove too fast for conditions”.
Driver Actions, Truck Crashes | Number of Identified Violation | Driver Actions, Related Violation |
---|---|---|
Failed to keep Proper Lane | 1 | Fail to drive within single lane |
Drove too fast for conditions | 1 | Speed too fast for condition |
Followed too close | 1 | Follow too closely |
5. RESULTS
5.1. Descriptive Analysis, Crash Data
Fig. (1) presents general characteristics of truck crashes on Wyoming interstates. As can be seen from Fig. (1a), most of the truck crashes (52%) involved just a single truck. Including both single (52%) and multiple vehicle, truck involved crashes (26%), about 78% of the truck drivers were at fault for truck crashes on Wyoming interstates. Driver actions with highest percentage, for both single and multiple truck related crashes, are included in Figs. (1b and c). As can be seen from Figs. (1b and c), the main causes of truck crashes, driver actions, include failing to keep proper lane, driving too fast for conditions, and following too close. Based on Figs. (1b and c), no improper driving, failure to keep proper lane, following too close account for 78% of multiple vehicle, truck involved, crashes and 65% of single truck crashes. That is the reason why the violations related to these driver actions are included in the violation analyses (Table 1). It should be noted that “no improper driving” action is a crash in which a driver had no improper driving, but was involved in a crash. Therefore, no violation was identified related to this driver action and this driver action was excluded from violation analyses.
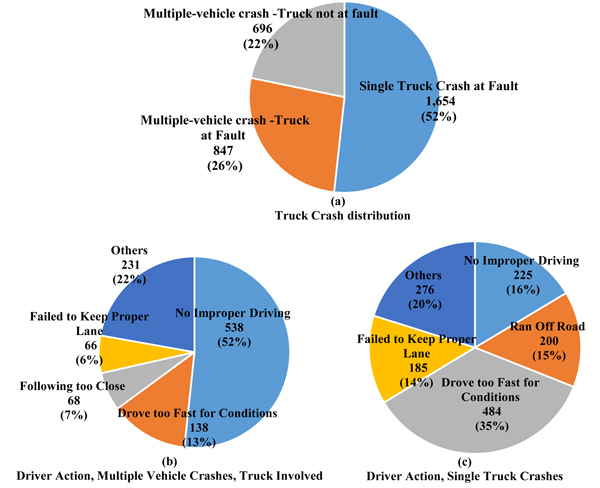
For the first analysis, data were filtered from the original file to include only single truck crashes. Summary statistics of significant variables that impacted the severity of single truck crashes are presented in Table 2. As can be seen from the table, most of the single truck crashes (85%) involved property damage only. Most of the drivers in single truck crashes were male (94%) compared with only 6% involving female drivers. Speed limit of 65 mi/hr was chosen as a threshold for the speed limit variable. This is because most speed limits on the included highways in Wyoming are greater than 70 mi/hr and 65 mi/hr was found to be the best threshold that can divide the crashes into similar categories. Although the majority of single truck crashes (71%) occurred at a posted speed limit of greater than 65 mi/hr, a rather large proportion (29%) occurred at a lower speed limit. Most of the single truck crashes (67%) occurred on not-dry-road conditions. Most single truck crashes (72%) were rollover or jackknife. In 16% of all the single truck crash cases, truck drivers had some type of distraction in the cabin. Distraction was defined as any distraction in a cabin such as wireless communication or TV.
Truck crashes | |||
---|---|---|---|
Variable Name | Number | % | |
Single Truck Crashes | |||
Injury single truck crash | Injury truck crash | 204 | 15 |
Property damage only | 1166 | 85 | |
Gender | Male | 1290 | 94 |
Female | 75 | 6 | |
Posted speed limit | Less than or Equal 65 MI/HR | 396 | 29 |
Greater than 65 MI/HR | 972 | 71 | |
Road condition | Dry | 450 | 33 |
Not dry | 920 | 67 | |
Rollover/Jackknife | Rollover/Jackknife | 993 | 72 |
Other types | 377 | 28 | |
Driver distraction | Has some Types of Distraction in Cabin | 217 | 16 |
There was no distraction | 1139 | 84 | |
Multiple Vehicle Crashes, Truck Involved | |||
Injury multiple vehicle crash, truck involved | Injury truck crash | 85 | 7 |
Property damage only | 1,203 | 93 | |
Speed compliance | Speed limit was followed | 1,203 | 93 |
Speed limit was not followed | 85 | 7 | |
Speed Limit was not followed | Dry-road condition | 32 | 38 |
Not-dry-road condition | 53 | 62 | |
Posted Speed Limit | Less than or equal 65 mi/hr | 365 | 29 |
Greater than 65 | 895 | 71 | |
Lower posted speed Limit | Dry-road condition | 161 | 44 |
Not-dry-road condition | 259 | 56 | |
Crash characteristic | Truck was at fault of crash | 749 | 58 |
Non-truck driver was at fault of the crash | 539 | 42 | |
Temporal characteristics | Business day | 808 | 63 |
Weekend | 480 | 37 |
For the second analysis, the data was filtered from the original file to include crashes involving at least one truck. Due to the involvement of at least two vehicles, only the summary statistics of a vehicle at fault is presented in Table 2. Seven percent of truck crashes occurred while the driver at fault did not follow the posted speed limit. To provide more insight about this variable, Table 2 also includes more statistics on the circumstance in which speed limit compliance was not fulfilled. In these crashes, 62% of the at-fault vehicles did not follow the posted speed limit while driving on not-dry-road conditions. Not-dry-road conditions include the road conditions other than dry, such as rainy/snowy. About 29% of truck crashes occurred at the locations with a speed limit of less than 65 mi/hr. More detailed summary is also provided for this variable in Table 2. The results indicated that most of the lower speed crashes occurred when the road was not dry (56%), which might be an indication that the locations were equipped with Variable Speed Limits (VSL). Forty four percent of the lower speed limits were also related to dry-road condition, which might be due to driving through work zones.
5.2. Statistical Modeling, Crash Data
5.2.1. Factors Associated with Higher Risk of Injury Single Truck Crashes
This first modeling approach investigated the variables that increase the odds of fatal/injury truck crashes compared to PDO truck crashes. Table 3 shows the variables included in the full model and the estimates for those variables remaining in the reduced model at the pre-specified significance levels. Being a male driver decreased the odds of being involved in injury single truck crashes; females are not more likely to be involved in a crash. However, when they are involved in a crash, they are more likely to be injured/killed. The results disagreed with the research carried out by Kim et al. (2013) indicating that being a male truck driver increases the odds of F+I single truck crashes [30]. It was found that dry-road conditions increase the odds of being involved in injury single truck crashes. This result is in contrast with the results obtained by Kim et al. (2013) indicating that wet or snowy/icy surfaces increased the odds of injury in single-vehicle crashes [31]. However, the difference between single vehicle and single truck crashes should be noted.
The lower odds of injury single truck crashes may lie in the fact that truck drivers drive more cautiously, with lower speed, on not-dry-road conditions. Speed limit is another variable that impacts the severity of single truck crashes on Wyoming interstates. The results indicated that by increasing speed limit, single truck crashes were more likely to be injury/fatality. The results confirmed the research carried out by Abdel-Aty (2000), which indicated that when drivers speed, the odds of being involved in an injury crash increase [32]. Rollover/jackknife was another important variable. The odds of an injury/fatal truck crash were estimated to be nearly 4 times higher if that truck crash involved a rollover/jackknife than if that truck crash did not involve a rollover/jackknife. The result was in accordance with the result found by Krull et al. (2000) [33]. It should be noted that this research included only rollover in all types of single vehicle crashes. Driver distraction was found to increase the odds of getting involved in injury single truck crashes. The result confirmed the research carried out by Bunn (2005), which indicated distraction/inattention increased the odds of a fatal motor vehicle collision [34].
Collision Characteristic | Predictors | Odds Ratio | 95% CL |
---|---|---|---|
Driver characteristics | Gender: Male truck driver (1 if true; 0 otherwise) | 0.35* | 0.19-0.62 |
Age group1:Younger driver: age <45 years (1 if true; 0 otherwise) | - | - | |
violation record1 : Traffic record of one ticket or less (0 if true; 1 otherwise) | - | - | |
Residency1: State of Wyoming (0 if true; 1 otherwise) | - | - | |
Speed limit compliance1: Truck Driver followed Speed Limit(0 if rue, 1 otherwise) | - | - | |
Environmental characteristics | Weather condition1: Clear (0 if true; 1 otherwise) | - | - |
Roadway-surface condition: Dry (0 if true; 1 otherwise) | 0.19* | 0.14-0.27 | |
Light condition1: daylight (0 if true; 1 otherwise) | - | - | |
Vehicle characteristics | CMW weight1: Truck greater than 26000 pound (1 if true; 0 otherwise) | - | - |
Temporal characteristics | Day of week1 : Weekends(1 if true; 0 otherwise) | - | - |
Time1: Peak hours 6< <22: (0 if true; 1otherwise) | - | - | |
Posted speed limit : Speed limit less than 65 mi/hr(0 if true; 1 otherwise) | 1.63* | 1.11-2.47 | |
Roadway characteristics | Traffic1 | - | - |
Crash characteristics | No of vehicle 1: 1(0 if true; 1 otherwise) | - | - |
Pre-collision vehicle actions1: Straight-ahead(0 if true; 1 otherwise) | - | - | |
Manner of collision1 | - | - | |
Rollover/Jackknife(1 if true; 0 otherwise) | 3.62* | 2.38-5.52 | |
Occupant ejection1: Driver is partially or totally ejected(1 if true; 0 otherwise) | - | - | |
No improper driving1 (1 if true; 0 otherwise) | - | - | |
Drove too fast for conditions1: (1 if true; 0 otherwise) | - | - | |
Fail to keep proper lane1: (1 if true; 0 otherwise) | - | - | |
Driver behaviors | Driver distraction: No distraction in truck(0 if true; 1 otherwise) | 1.72* | 1.16-2.60 |
DUI suspicion1 : Driver was suspected of driving under the influence condition(1 if true; 0 otherwise) | - | - | |
Sign of fatigue1: Driver was fatigued(1 if true; 0 otherwise) | - | - | |
CMV driver safety technology in use1: Safety equipment was not used (1 if true; 0 otherwise) | - | - |
*indicates p<0.05
1 indicates insignificant variable included in the initial model
Table 4 provides possible explanations behind the variables identified in the logistic model for severity of single truck crashes. For instance, the increased odds of being involved in injury/fatal single truck crashes, with distraction in the cabin, may be related to drivers being less attentive to road hazards. Also, a female driver may have higher odds of being involved in injury/fatal single truck crashes since females are less able to sustain different types of physical trauma [35].
Variable | Relationship | Possible Reason |
---|---|---|
Female truck driver | It increases the odds of injury/fatal single truck crashes. | Women are less able to sustain physical trauma. |
Wet roadway surface condition | It decreases the odds of injury/fatal single truck crashes. | Drive more cautiously, with less speed, on not-dry-road conditions. |
Increased posted speed limit | It increases the odds of injury/fatal single truck crashes. | Drive faster in higher posted speed limit locations. |
Rollover/Jackknife | It increases the odds of injury/fatal single truck crashes. | Increased the contact of driver with road and vehicle. |
Driver distraction | It increases the odds of injury/fatal single truck crashes. | Drivers are less cautious about the hazard associated with Wyoming mountainous area. |
5.2.2. Factors Associated with Higher Risk of Injury Multiple Vehicle Crashes, Truck Involved
After learning about contributory factors to injury single truck crashes, an analysis was run to identify contributory factors to injury in multiple vehicle crashes, with involvement of at least one truck. Out of 25 included variables, 3 variables were found to be important at the pre-specified significance level. As can be seen from Table 5, lack of speed limit compliance had the highest estimated impact among the variables in this crash analysis. The odds of this crash were estimated to be 3.34 times higher when the truck driver did not follow the speed limit. Although not much research has been done on the importance of speed compliance in preventing injury truck crashes, many studies showed that increased vehicle speed significantly increased the odds of being involved in fatal/injury crashes [36, 37]. Posted speed limit is another speed variable that increased the odds of being involved in fatal/injury crashes. An increase in injury/fatal crashes was identified during weekends. This impact can be due to a variety of reasons such as less congestion traffic, higher travel speeds, and a desire to reach a destination in a timely manner. The possible reasons for other important variables are presented in Table 6.
As can be noticed from the results presented in Tables 3 and 5, the only common predictor between crash severity for single truck and multiple vehicle, truck involved, crashes related to posted speed limit. Increased posted speed limit increased the crash severity for single truck crashes (1.63) and multiple vehicle crashes (1.85).
Collision Characteristic | Predictors | Odds Ratio | 95% CL |
---|---|---|---|
Driver characteristics | Gender1: Male truck driver (1 if true; 0 otherwise) | - | - |
Age group1:Younger driver: age <45 years (1 if true; 0 otherwise) | - | - | |
violation record1 : Traffic record of one ticket or less (0 if true; 1 otherwise) | - | - | |
Residency1: State of Wyoming (0 if true; 1 otherwise) | - | - | |
Speed Limit compliance1: Truck Driver followed Speed Limit(0 if rue, 1 otherwise) | 3.34* | 1.75-6.35 | |
Environmental characteristics | Weather condition1: Clear (0 if true; 1 otherwise) | - | - |
Roadway-surface condition1: Dry (0 if true; 1 otherwise) | - | - | |
Light condition1: daylight (0 if true; 1 otherwise) | - | - | |
Vehicle characteristics | CMW weight1: Truck greater than 26000 pound (1 if true; 0 otherwise) | - | - |
Temporal characteristics | Day of week : Weekends(1 if true; 0 otherwise) | 1.70* | 1.22-2.36 |
Time1: Peak hours 6< <22: (0 if true; 1otherwise) | |||
Posted speed limit : Speed limit less than 65(0 if true; 1 otherwise) | 1.85* | 1.25-2.74 | |
Roadway characteristics | Traffic1 | - | - |
Crash characteristics | No of vehicle 1: 1(0 if true; 1 otherwise) | - | - |
Pre-collision vehicle actions1: Straight-ahead(0 if true; 1 otherwise) | - | - | |
Manner of collision1 | - | - | |
Rollover/Jackknife1 (1 if true; 0 otherwise) | - | - | |
Occupant ejection1: Driver is partially or totally ejected(1 if true; 0 otherwise) | - | - | |
No improper driving1 (1 if true; 0 otherwise) | - | - | |
Drove too fast for conditions1: (1 if true; 0 otherwise) | - | - | |
Fail to keep proper lane1: (1 if true; 0 otherwise) | - | - | |
Driver behaviors | Driver distraction1: No distraction in truck(0 if true; 1 otherwise) | - | - |
DUI suspicion1 : Driver was suspected of driving under the influence condition(1 if true; 0 otherwise) | - | - | |
Sign of fatigue1: Driver was fatigued(1 if true; 0 otherwise) | - | - | |
CMV driver safety technology in use1: Safety equipment was not used (1 if true; 0 otherwise) | - | - |
Variable | Relationship | Possible Reason |
---|---|---|
No speed compliance | It increases the odds of getting involved in injury multiple vehicle crashes | Drive faster than recommended speed limit. |
Weekend driving | It increases the odds of getting involved in injury multiple vehicle crashes. | Less traffic, higher speeds, or rush to reach a destination. |
Increased posted speed limit | It increases the odds of getting involved in injury multiple vehicle crashes | Drive faster on increased posted speed limit locations. |
5.3. Descriptive Analysis, Violation Data
The literature indicated that previous violations can be used to predict future crashes. Therefore, for a better understanding of the enforcement efficiency, this section aimed to include the most common types of driver violations resulting in truck crashes. The objective of this section was to identify the groups that are more at risk of violating the laws that can result in truck related crashes. Figs. (1b and c) showed the highest proportions of driver actions in truck involved crashes. The figures revealed that driving too fast for conditions (13% for multiple vehicle crashes compared to 35% for single truck crashes), no improper driving (16% for single truck crashes compared to 52% for multiple vehicle crashes), failure to keep proper lane (6% for multiple vehicle crashes compared to 14% for single truck crashes), and following too close (7% of multiple vehicle crashes) accounted for 65% of all the single truck crashes and 78% of multiple vehicle crashes. Therefore, violations related to aforementioned driver actions were included in the violation analyses. No violation was identified for “no improper driving” action as drivers had no improper driving, but were involved in this type of crashes. Therefore, this type of driver action was excluded from the violation analyses.
The summary statistics of significant variables and the summary statistics of the responses are included in Table (7). Violation analyses were divided into two sections: truck related violations and all types of violations. For single truck crashes, only trucks were at fault in the crashes, so only truck violation data were used to identify truck drivers being at higher risk of future crashes. For multiple vehicle crashes, with an involvement of at least one truck, truck and no truck vehicles/drivers could be at fault in the crashes. Therefore, all types of violations were used for investigating the drivers at higher risk of involvement in multiple vehicle crashes, with involvement of at least one truck. Only 12% of all the citations were assigned to trucks. Other types of violation, “others”, included violations such as seat belt, driving under the influence (DUI), and Hour Of Service (HOS) violations. Nonresidents of Wyoming accounted for 70% of all the truck violators and 33% of all the violators. The WHP allocated only 11% of all their resources during off peak hours and most of the violators were male (97%).
Violation | Number | % |
---|---|---|
Truck Violation | 17,239 | 12 |
Non-truck Violation | 121,680 | 88 |
Truck Related Violations | ||
Speed too fast for condition | 226 | 1.38 |
Fail to drive within single lane | 755 | 4.62 |
Speeding | 3,179 | 19.5 |
Others | 11,800 | 70.3 |
Wyoming Resident | 2,010 | 12 |
Non Wyoming Resident | 14,327 | 88 |
Weekend | 4,423 | 27 |
Business days | 11,914 | 73 |
Peak Hours | 14,584 | 89 |
Off Peak Hours | 1,753 | 11 |
Male | 15,814 | 97 |
Female | 482 | 3 |
All Violations | ||
Speed too fast for condition | 1,239 | 0.9 |
Fail to dive within single lane | 2,930 | 2.1 |
Speeding | 86,984 | 62.6 |
Following too Close | 1,144 | 0.8 |
Others | 45,855 | 33 |
Weekend | 39,568 | 30 |
Business days | 93,266 | 70 |
Peak Hours | 124,621 | 94 |
Off Peak Hours | 8,113 | 6.1 |
5.4. Statistical Modeling, Violation Data
5.4.1. Factors Associated with Higher Risk of single Truck Crashes, Risky Truck-Related Violations
This section identified the risk of different truck drivers in violating particular traffic laws, with different driver and temporal characteristics. Only truck related violations were considered in this section as the risk of involvement in single-truck crashes were evaluated. Also, only traffic violation types that are associated with single truck crashes were included in this analysis (Fig. 1b). Therefore, the truck related violations were filtered to include only violations titled: Speed too fast for conditions and failure to drive within single lane. The aforementioned violations, driver actions, accounted for 49% of all single truck crashes, driving too fast for conditions (35%), and failure to drive within single lane (14%). Results of this analysis are presented in Table 8. As can be seen from this table, nonresidents of Wyoming, driving on weekend, and driving in off peak hours, all increased the odds of driving trucks too fast for conditions. These variables, based on the literature, could consequently increase the risk of being involved in single truck crashes. In particular, the odds of violating driving too fast for conditions were estimated to be 2.21 times higher for a nonresident than for a resident of Wyoming.
Except for failure to drive within single lane, all the variables included in the model were found to affect the odds of violating this law. Being female, a nonresident of Wyoming, driving on the weekend, and driving off peak hours all increase the odds of a violation related to failure to keep the proper lane. Being nonresident (2.00) and driving during weekends (2.31) have the highest estimated odds of such a violation.
– |
Speed Too Fast for Condition | Failure to Drive within Single Lane | |
---|---|---|---|
Driver characteristics | Driver gender Male truck driver (1 if true; 0 otherwise) | - | 0.65* |
Residence group: Non residence(1 if true; 0 otherwise) | 2.21* | 2.00* | |
Temporal characteristics | Date: weekends(1 if true; 0 otherwise) | 1.97* | 2.31* |
Time: peak 6< <22(0 if true; 1 otherwise) | 1.55* | 1.25* |
-indicates insignificant variable
Variable | Relationship | Possible Reason | Possible Reason |
---|---|---|---|
Female | Increase the risk of not keeping proper lane. | Have higher score for errors and violations. | Have higher score for errors and violations. |
Non residence | Increase the risk of driving too fast for the condition and failing to keep proper lane. | Lack of familiarity with mountainous areas in Wyoming. | Lack of familiarity with mountainous areas in Wyoming. |
Weekend | Increase the risk of driving too fast and failing to keep proper lane. | Less traffic and higher speeds. | Less traffic and higher speeds. |
Off peak hours | Increase the risks of all these violations. | Possible fatigue. | Possible fatigue. |
5.4.2. Factors associated with higher risk of multiple vehicle, truck involved crashes, all types of risky violations
As Fig. (1a) indicated, in 22% of the multiple vehicle crashes, truck involved, non-truck drivers could also be at fault. As a result, all types of violations, truck and no truck violations, were included in this analysis to identify the risk of different drivers, with different temporal and driver characteristics, in violating particular traffic laws. Only traffic violations that are the main causes of multiple vehicle truck crashes were included in this analysis. As descriptive analysis in Fig. (1b) shows, 26% of multiple vehicle crashes were related to driving too fast for conditions (13%), following too close (7%), and failure to keep proper lane (6%). Therefore, only violations related to aforementioned driver actions were included in the violation analysis.
As can be seen from Table 10, being a truck driver increased the odds of violating all of these laws. This is consistent with the results in Fig. (1a) showing that truck drivers are at fault in 78% of all truck related crashes. Contrary to the results obtained from single truck violation analysis, driving on weekends decreased the odds of driving too fast for conditions and failing to drive within single lane violations. The possible reason for the difference between the impact of this variable for this analysis and single truck violation analysis could be related to the fact that in this analysis about 90% of violators are non- truck drivers. On the other hand, driving off peak hours, similar to the previous analysis, was an indication of increased odds for driving too fast for conditions and failing to keep proper lane.
Groups | Drive too Fast for Condition | Failure to Drive within Single Lane | Following too Close | |
---|---|---|---|---|
Driver characteristics | Driver gender Male truck driver (0 if true; 1 otherwise) | - | - | - |
Residence group: Non residence(1 if true; 0 otherwise) | - | - | - | |
Type of vehicle | No truck(0 if true; 1 otherwise) | 2.22* | 2.85* | 2.26* |
Temporal characteristics | Date: weekends(1 if true; 0 otherwise) | 0.27* | 0.60* | - |
Time: peak 6< <22(0 if true; 1 otherwise) | 1.67* | 3.00* | - |
-indicates insignificant variable.
6. CONCLUSION AND DISCUSSION
Crash data from WYDOT was used to study the impacts of various variables on injury/fatal single truck and multiple vehicle, truck involved crashes. While much research has been done on the contributory factors to both multiple-vehicle and single truck crashes as a whole, this work investigated multiple-vehicle and single truck crashes separately. One of the reasons that single truck crashes were analyzed separately is the importance of this type of crash on Wyoming interstates. As it was shown in this study, more than 50% of all the truck related crashes were related to single truck crashes. This study also just focused on mountainous interstates in Wyoming that have the highest truck crash rates in the state. Moreover, this study included violation data in addition to truck related crash data.
The results indicated that various variables impact single truck and multiple vehicle crashes, with involvement of at least one truck. Being female, driving on dry-road conditions, driving with increased posted speed limits, rollover/jackknife type of truck crashes, and having distraction in the cabin were factors that increased the odds of injury/fatal single truck crashes. As for multiple vehicle crashes, truck involved, different variables impacted this type of crash. Non-speed limit compliance, driving on segments with higher speed limit, and driving during weekends were the factors that increased the odds of getting involved in multiple vehicle crashes leading to fatality or injury. Posted speed limit was a variable that increased the odds of injury/fatal crashes for both analyses.
The literature has indicated that previous violations can be used to predict future offenses and crashes. Therefore, the current study used violation data, in addition to crash data, to examine the groups that are more likely to violate the laws, which account for the majority of driver actions in single truck and multiple vehicle crashes. The violations leading to truck crashes were identified from more than 800 violations and the risk was examined. The results indicated that nonresidents of Wyoming were at higher risk of driving too fast for conditions and failure to drive within single lane. Driving during off peak hours and on weekends also increased the odds of violating these laws.
About 50% of all the multiple vehicle crashes were caused by non-truck drivers. Therefore, both truck and non-truck violations were included in this analysis. Violations related to dominant causes of multiple vehicle crashes were identified and the violation analysis was run only on those violations. The variables included in this analysis were similar to single truck crashes, with the difference that following too close was also added to the contributory factors. Overall, results indicated that being a truck driver plays a dominant role in these types of violations. Truck drivers were more at risk of violating the laws that lead to about 26% of all the causes of multiple vehicle crashes. Driving off peak hours also increased the odds of being involved in violations such as driving too fast for conditions and failing to keep proper lane.
7. RECOMMENDATIONS
Soole et al. (2013) reported that enforcement was effective in changing driver behavior resulting in reduced crash rates [39]. Studies also showed that 90% improvement in law compliance can be achieved by enforcement approaches [40]. Therefore, WHP can play an important role in reducing the high truck crash rate in Wyoming by allocating resources in the correct areas.
The following recommendations can be given based on this study:
- Nonresidents of Wyoming were more likely to be involved in risky violations that can result in truck related crashes. Therefore, the WHP should educate the nonresidents about the hazards associated with driving in mountainous areas in Wyoming.
- Distraction in the cabin was another variable that increased the odds of getting involved in injury/fatal single truck crashes. Distracted driving laws need to be toughened.
- Weather and lighting conditions increased the odds of being involved in multiple vehicle crashes. The WYDOT should educate the drivers about the risk of driving on not clear weather and night time conditions by displaying speed related messages on dynamic message signs (DMS).
- Truck drivers were more likely to be involved in driving too fast for condition, failing to keep proper lane, and following too close. Stricter measures should be taken for truck drivers who violate these laws.
- Weekend driving increased the odds of injury crashes for both single and multiple truck crashes. Weekend driving also increased the odds of being involved in risky violations. Only 30% of the WHP resources were dedicated to law enforcement on these days. More activity should take place on these days.
It is recommended for the future studies to incorporate the impact of different active safety systems such as adoptive cruise control, forward collision warning and braking, road sign detection, and night vision in their studies.
CONSENT FOR PUBLICATION
Not applicable.
CONFLICT OF INTEREST
The authors declare no conflict of interest, financial or otherwise.
ACKNOWLEDGEMENTS
The Wyoming Technology Transfer (WYT2/LTAP) center at the University of Wyoming provided extensive resources to assist in the compilation of the data sets used. WYDOT provided the funding of this study. Special acknowledgement goes to WHP and WYDOT for providing the data used in this research.