All published articles of this journal are available on ScienceDirect.
From Intention to Actual Behavior to Adopt Battery Electric Vehicles: A Systematic Literature Review
Abstract
Background:
Climate change and global warming are severely impacting countries worldwide. Governments are being urged to promote Battery Electric Vehicles (BEVs) as an alternative to conventional vehicles, which are one of the main sources of emissions and pollution. Despite their environmental benefits, BEV adoption remains at a low level. Therefore, identifying the determinants of BEV adoption is necessary to promote greener transportation.
Objective:
This study aims at identifying and synthesizing factors affecting BEV adoption intention and actual behavior. A comparison and analysis will be made to draw meaningful results and suggest future research areas.
Methods:
A systematic literature review was conducted. Data were collected from research articles in peer-reviewed journals of well-known publishers. After a strict qualification process, 45 studies were selected and their findings are critically discussed.
Results:
Most studies are quantitative in nature and conducted in BEV developed markets. Various determinants of BEV adoption intention were identified and classified into 5 categories: psychological, behavioral, product attributes, contextual, and demographic. Meanwhile, only a few studies examine BEV's actual adoption behavior. Antecedents of adoption behavior were categorized into 4 groups, namely, psychological, behavioral, contextual, and demographic.
Conclusion:
BEV adoption can be influenced by both internal and external factors. There is a gap in research between intention and behavior in previous studies related to BEV adoption. While determinants of intention are the topic of many studies, actual behavior is more important but limitedly explored. Future research can focus on adoption behavior in newly developed markets with real data rather than utilizing self-reported responses to better analyze actual behavior.
1. INTRODUCTION
Despite the fact that transportation plays an indispensable role in the social and economic development of every nation, the growth of the transportation sector has raised a great concern due to its impact on the environment. The global number of vehicles in use was estimated at nearly 1.3 billion [1], which is a major source of greenhouse gas (GHG) emissions. According to International Energy Agency - IEA [2], transportation accounts for about one-fourth of the global carbon emissions, which is responsible for global warming and climate change. Additionally, the great number of vehicles directly undermines public health by increasing the risk of respiratory and cardiovascular diseases under the effect of poor air quality [3].
To alleviate these issues, many governments have focused on replacing internal combustion engine vehicles (ICEVs) with electric vehicles (EVs) as they offer many environmental advantages. EVs are alternative fuel vehicles that operate solely or partially by electricity [4]. EVs can be classified into 3 categories [5]. Battery Electric Vehicles (BEVs) are fueled only by electricity through a rechargeable battery. Hybrid Electric Vehicles (HEVs) combine an internal combustion engine and an electric motor. Plug-in Hybrid Electric Vehicles (PHEVs) make use of an internal combustion engine and an electric motor powered by a rechargeable electric battery. The development of EVs is expected to reduce the amount of emissions, oil dependency, and noise pollution and increases energy efficiency [6-8]
However, the adoption rate of EVs around the world is relatively low. EVs are mainly popular in China, EU, and the United States. It was reported that the total number of EVs worldwide reached 10 million units, making up only 3% of the global car market share in 2020 [9]. Promoting the shift from traditional vehicles to EVs requires deep understanding of the factors involved in the customer’s decision making. There have been many research papers exploring the determinants of EVs adoption. Based on different methods and approaches, various factors have been recognized, such as demographic characteristics, technical features, psychological factors, policies, and incentives [10-12]. Therefore, it is necessary to systematically review and summarize the diverse determinants of EV’s adoption in a single literature review to lay a solid foundation for future research.
Several literature reviews have related to this topic, but most of them pay little attention to the differences among EV types. Particularly, the review of Kumar & Alok [13] and Singh et al. [14] focused on factors affecting EV adoption in general (BEVs, PHEVs, and HEVs). The review of Rezvani et al. [15] and Coffman et al. [16] focused on BEVs and PHEVs. As BEVs depend solely on electric batteries, they are remarkably different from hybrid vehicles (HEVS and PHEVs). Regarding BEVs' characteristics, they are pure electric vehicles and do not release tailpipe emissions. Although a BEV's emission in the manufacturing stage is higher than an ICEV, a BEV is still environmentally friendly as its emission amount is 18% less than an ICEV during its entire life cycle [17]. The diffusion of BEVs also reduces oil dependency in transportation as electricity can be generated from various alternative sources such as solar, wind, and biomass [18]. In addition, the energy efficiency of BEVs is about 70%, while the figure for ICEVs is only 18% [19]. However, there are several technological disadvantages of BEVs reported in previous research. Depending solely on battery limits the operating range of BEVs and requires charging stations as well as a long charging time [20]. Additionally, high battery cost, which contributes 36-50% to the production cost of a BEV, is also a barrier to customer purchase decisions [21]. Another drawback of BEV is the disposal of used batteries, which could pose environmental risks due to metal toxicity; however, this problem is being dealt with by recent advancements in battery technologies [19].
In general, as compared to ICEVs, BEVs are greener in terms of tailpipe emissions, more advanced in technology, and require significant customer behavioral changes [22]. Previous research articles often ignored the differences between EV categories, but customers’ views may differ among EV types [22]. Therefore, it is necessary to treat the determinants of BEV adoption independently instead of grouping factors affecting EV adoption in general. Additionally, previous reviews rarely separated between adoption intention and actual behavior. Thus, this research focuses on identifying factors affecting the adoption intention and actual behavior of consumers toward BEVs.
The rest of this study is as follows. The next section introduces the research methodology, which specifies the identification strategy and content analysis. The results of this review are presented in Section 3, which includes descriptive statistics and determinants of Bevs' adoption intention and behavior. Section 4 discusses the determinants of BEV adoption in detail. Finally, the conclusion section provides comparisons and recommendations of opportunities for future research.
2. RESEARCH METHODS
This review followed the selection process proposed by Tripathi & Singh [23]. The first step of this systematic review is identifying keywords related to the topic. Specifically, the keyword string includes (“battery electric vehicle” OR “full electric vehicle” OR “pure electric vehicle”) AND (“purchase” OR “adoption” OR “buy” OR “acceptance”) AND (“intention” OR “behavior”). Credible databases chosen for this review were SCOPUS, Science Direct, Emerald, Sage, Wiley, Taylor & Francis, and Springer. In total, the number of studies that resulted from the keyword string was 3511.
Inclusion criteria were developed to select articles relevant to the research purpose. First of all, the content of qualified research papers must focus on exploring factors that influence the adoption intention or behavior of customers. Secondly, this review only considers empirical work instead of conceptual and theoretical research to ensure the objectivity of findings. Thirdly, only research articles written in English in peer-reviewed academic journals are examined. Finally, the timeframe was set within the last 5 years (from 2017 to 2021) to acquire up-to-date data.
After applying filters related to article type, language, and timeframe, 1525 out of 3511 articles remained. By scanning titles and abstracts, 1438 studies were removed due to irrelevance. In the final step, full-text reading was performed on the remaining 87 papers. 2 reviewers worked independently to exclude studies that do not focus mainly on influencing factors of BEV adoption, and disagreements were settled by consensus. This step further excluded 42 articles and 45 studies were selected.
Regarding the content analysis of the selected papers, the descriptive statistics of the chosen articles were analyzed to form a general view of the publishing period, regional location, and research methodology. After that, antecedents of BEV adoption intention and behavior were identified, categorized, and discussed in detail. The search strategy and results are presented in Fig. (1).
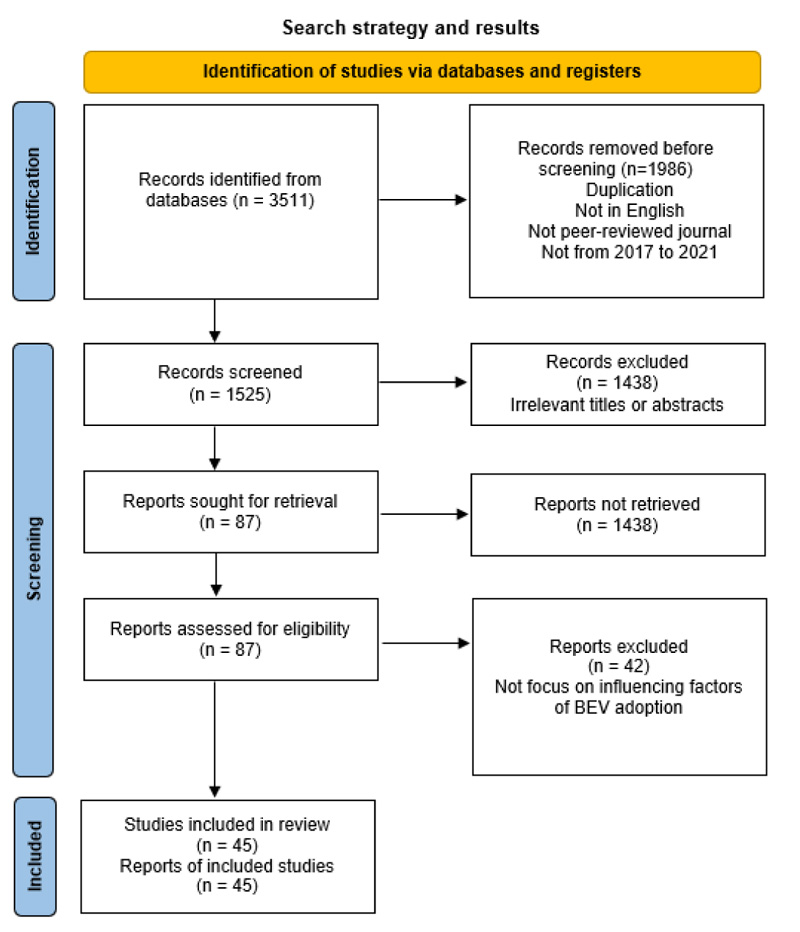
3. RESULTS
3.1. Descriptive Statistics
As presented in Fig. (2) below, there was an outstanding number of articles relevant to this study purpose published in 2021. Particularly, 18 out of 45 papers were published in 2021, accounting for 40% of the sample. It suggests that investigating factors affecting BEV adoption has become a major research interest recently.
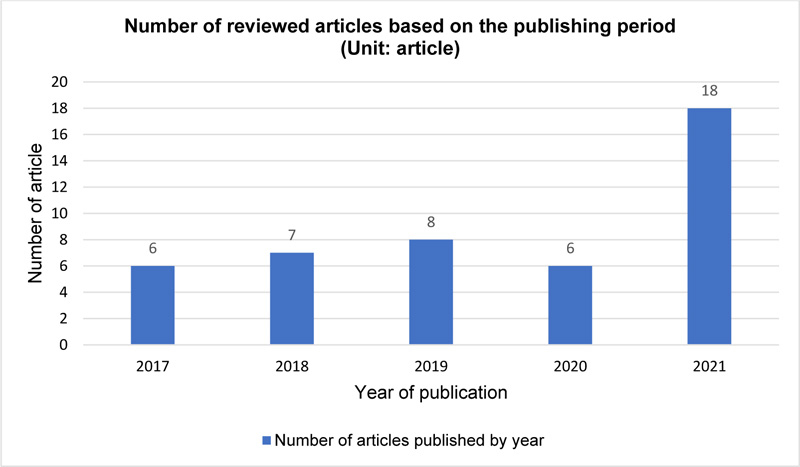
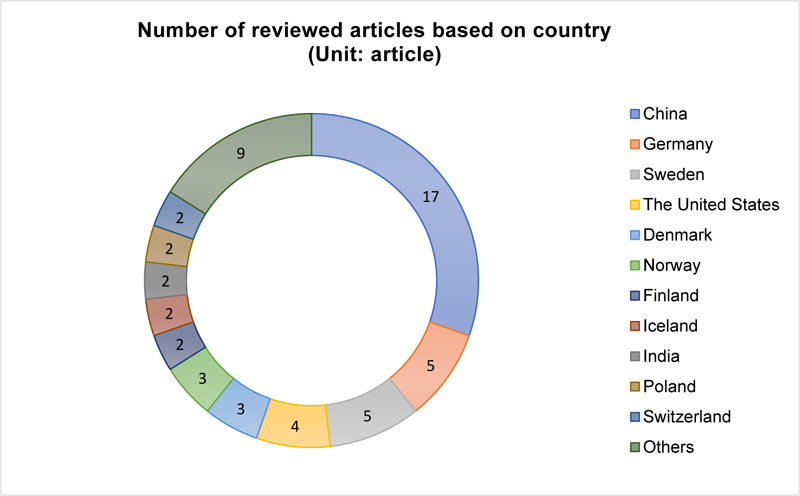
*The number of articles exceeds 45 as some studies are conducted across nations
As illustrated in Fig. (3), most research focused on BEV adoption in China, which is the world's largest market in terms of population. Additionally, many articles examined BEV adoption in European countries, especially in Germany and Nordic countries. In general, most studies are conducted in markets with a high BEV adoption rate, such as China, EU, and the US, as reported by IEA [9]. The authors also identified 4 cross-national research papers investigating and comparing BEV adoption among countries. It shows that the impact of cultural differences on BEV adoption is a potential research topic with a limited number of articles.
Regarding previous research methods presented in Table (1), most studies are quantitative in nature (n=41). A common quantitative research method is to conduct choice experiments. In this approach, researchers pre-design a series of hypothetical scenarios or choice sets, including several vehicle options. Respondents are required to choose a vehicle option that they prefer. Each vehicle option is described in detail with some product attributes based on which decisions are made by respondents. Every response is recorded by a stated preference survey and analyzed using choice models. This method enables researchers to analyze the impact of different selected attributes on customer decision [24,25]. Another popular approach is to extract participants’ responses through a survey and analyze data using structural equation modelling (SEM) or multiple linear regression. In this approach, a self-reported questionnaire is sent to a sample of the target population (for example, BEV owners, potential BEV buyer, ICEV drivers, etc.) to gather responses. Based on the collected data, researchers could use statistical models to measure and analyze the relationship between variables. Paticularly, researchers usually use this method to validate hypotheses related to the relationship between BEV adoption and its determinants [26-28]. The dominance of quantitative research in this topic could be explained due to its ability to verify the impact of factors on a large sample and generalize the results to a broad population.
On the contrary, only 2 qualitative studies were identified in this review. They all utilized semistructure interviews with EV drivers and experts to identify and explain the underlying motivations for BEV adoption [29, 30]. In the interview, the interviewers only ask a few predetermined questions and then have a follow-up conversation to further examine the interviewees’ opinions. Therefore, this qualitative method provides flexibility and deeper insights into the subject of concern [30]
Mixed method was also identified in 2 articles. In these studies, data collection through interviews was used as an addition to the literature review to form hypotheses that were later validated by statistical models [31, 32].
Method | Quantitative | Qualitative | Mixed |
---|---|---|---|
Number of studies | 41 | 2 | 2 |
Categories | Explanatory factors | Article |
---|---|---|
Psychological | Intention | [51] |
Satisfaction with incentive policies | [51] | |
Technology affinity | [64] | |
Green party preference | [64] | |
Environmental awareness | [64] | |
Behavioral | Usage of Carsharing | [64] |
Contextual | Charging infrastructure | [32, 57, 65-67] |
Monetary incentive policies | [65, 67] | |
Non-monetary policies | [65, 66] | |
Demographic | Age | [32, 57] |
Gender | [57] | |
Education | [32, 57] | |
Accommodation ownership | [32, 57] | |
Income | [57, 64] | |
Vehicle ownership | [64] | |
Household size | [57] | |
Commuting time | [32] |
Categories | Explanatory Factors | Article |
---|---|---|
Psychological | Attitude | [26, 28, 29, 31, 33-39] |
Perceived Behavioral control | [26, 28, 29, 34, 36, 39-42] | |
Subjective norm/Social influence | [26, 29, 34-36, 39-47] | |
EV experience | [11, 30, 36, 40, 44, 47-50] | |
Environmental value | [11] | |
Technology affinity/consciousness | [43] | |
Performance expectancy | [51] | |
Hedonic Motivation | [27, 42, 46, 51] | |
Price value | [51] | |
BEV knowledge | [30, 48] | |
Gain motivation | [27] | |
Normative motivation | [27, 39, 42] | |
Perceived technology | [28] | |
Perceived return | [49] | |
Perceived risk | [49, 52] | |
Innovativeness | [45, 47] | |
Interest in new technologies | [37] | |
Face consciousness | [38] | |
Deontological evaluation | [53] | |
Risk aversion | [53] | |
Environmental concern | [38, 46, 54] | |
Brand recognition | [37, 52] | |
Status symbol | [55] | |
Environmental symbol | [55] | |
Innovation symbol | [55] | |
Collective efficacy | [47] | |
Perceived information | [47] | |
Brand image | [52] | |
Brand identity | [52] | |
Behavioral | Travel distance | [11, 56, 57] |
Solar panel adoption | [11, 58] | |
Recycling | [11] | |
Changing diets | [11] | |
Usage of carsharing | [58, 59] | |
BEV driving habit | [51] | |
Eco-friendly behaviors | [37] | |
Driving frequency | [58] | |
Product attributes | Environmental attributes/performance | [26, 35, 41, 47] |
Range | [25, 30, 46, 56, 57, 60-62] | |
Price and cost | [11, 25, 43, 54, 57, 60, 62, 63] | |
Charging time | [11, 25, 62] | |
Vehicle-to-grid capability | [11] | |
Ease of operation | [11] | |
Technical reliability | [11] | |
Fuel Economy | [11] | |
Energy efficiency | [11] | |
BEV benefits/advantages | [43, 44] | |
Instrumental attribute | [28, 58] | |
Emissions | [25] | |
Performance barriers | [35, 43, 63] | |
Contextual | Charging infrastructure | [25, 30, 37, 44, 46, 56-58, 62, 63] |
Monetary incentive policies | [24-26, 38, 39, 48, 51, 56, 57] | |
Non-monetary policies | [48, 56, 62] | |
Marketing, distribution and aftersales service | [43] | |
Facilitating conditions | [46, 51] | |
Demographic | Age | [11, 48, 56, 57] |
Education | [48, 56-58] | |
Vehicle demand | [56, 57] | |
Vehicle ownership | [56, 58, 60] | |
Budget | [56] | |
Gender | [11, 37, 48, 57, 58] | |
Income | [11, 56, 47, 57, 58] | |
Number of children | [11] | |
Ethnicity | [58] | |
Household size | [28] |
3.2. Determinants of BEV Adoption Intention and Behavior
This systematic literature review identified 38 articles examining determinants of BEV adoption intention (Table 2) and 5 articles related to adoption behavior (Table 3). There are 2 research papers concerning both BEVs’ adoption intention and behavior.
All determinants were presented in “Explanatory factors” column and they are divided into 5 categories: (1) Psychological category includes internal subjective factors such as attitude, perception, subjective norms, motivation and symbols; (2) Behavioral category covers human behaviors that affect their BEV adoption; (3) Product attributes refer to BEV technical specifications such as price, range and charging time; (4) Contextual category deals with external supporting conditions such as policy and infrastructure; (5) Demographic category includes individual demographic characteristics such as age, gender, education, and vehicle ownership.The “Article” column presents the number in the reference list.
4. DISCUSSION
4.1. Determinants of BEV Adoption Intention
4.1.1. Psychological Factors
There are various psychological factors identified in (Table 2). For simplicity, only popular themes are reported as below.
Attitude: Many previous works considered attitude as a major factor affecting BEV adoption intention. However, there is a slight difference among researchers in terms of attitude objects. Particularly, some researchers addressed attitude toward buying BEVs, whereas some stressed the attitude toward BEVs themselves. Xu et al. and Ackaah et al. referred to attitude as a positive or negative evaluation toward a certain behavior and found a strong positive relationship between the attitude of buying a BEV and purchase intention [26, 34]. Müller further pointed out that this relationship is weaker in China as compared to Europe and North America, which might be explained by cultural differences [33]. In addition, attitude towards BEVs and their attributes also positively impact intention [28, 29, 31]. The research of Liu et al. showed that favorable evaluation of BEVs, emission and noise result in higher adoption intention [36].
Perceived behavioral control (PBC): Schmalfuß et al. defined PBC as an individual’s perceived ability to perform a behavior based on his/her internal and external resources [40]. In other words, PBC represents the extent to which a person believes that he/she can do a task. Xu et al. pointed out that PBC is the strongest predictor of adoption intention instead of attitude [26]. The research of Pradeep et al. (2021), Liu et al. (2020), and Du et al. (2018) also confirmed that customers tend to buy BEVs if they assert high affordability and decision-making ability [28, 36, 39].
Subjective norm/social influence: Ackaah et al. described subjective norm as an individual’s perceived social pressure of whether to perform a behavior or not [34]. Krishnan & Koshy posited that a person can be influenced by social views and others’ interests in peer groups [43]. Thus, to promote BEVs adoption, it is necessary to make BEVs familiar to public groups, maybe through public exhibitions and free test drives. Du et al. indicated that the subjective norm positively impacts purchase intention, therefore, people are more likely to buy BEVs if they receive recommendations from others in their social network [39]. The authors argued that BEV purchase intention is based on herd mentality or peer pressure, which is socially influenced.
EV experience: EV experience could be understood as previous exposure to EVs. Chen et al. suggested that BEV adoption is a “learning by driving” process in which users learn and form attitudes while driving [11]. More exposure results in higher familiarity and stronger affinity, increasing the chance that they will adopt a new BEV in the future. The study of Kim et al. showed similar results and recommended the test drive as a useful promotion method to strengthen adoption intention in the South Korean market [48]. As BEVs are a new form of transportation, most people are unaware and have limited knowledge. Schmalfuß et al. proved that people having direct experience (using experience) with BEVs evaluate them more favorably and express a stronger willingness to purchase [40]. Similarly, Hinnüber et al. conducted a test drive experiment with 114 German participants and concluded that the first-time BEV driving experience enhances participants’ perception of the product and increases the adoption intention [50].
Motivations: Motivations are the forces that drive people’s behaviors. The study of Rezvani et al. pointed out 3 types of motivations [27]. Firstly, gain motivation is the rational trade-off between cost and benefits when the customer adopts BEVs. If the perceived comparisons between benefits and cost are positive, customers are more likely to adopt BEVs. Secondly, norm motivation or personal norm refers to individual moral obligations to perform a behavior. The awareness of environmental problems and perceived individual responsibility encourage customers to engage in sustainable behaviors such as buying an environmentally-friendly car. This argument is also supported by the research findings of Dong et al. [42] and Du et al. [39]. Thirdly, hedonic motivation, which includes emotions and feelings, was found to be an important predictor of BEV adoption. It is posited that customers aim at positive emotions and feelings during consumption and avoid negative ones. Therefore, the adoption intention will be strengthened if BEVs bring a pleasant and enjoyable driving experience [46, 51].
Environmental concern: Environmental concern refers to people’s consciousness towards environmental problems. As BEVs help reduce CO2 emissions, air pollution, and climate change impact, BEV adoption is considered an environmentally friendly act. Therefore, it is influenced not only by self-interest gains but also by benefits to the environment [54]. As the awareness of environmental issues is rising, intending to adopt BEVs is a way to decrease perceived concern and show social responsibility [46].
Symbol: Products usually carry symbolic meanings that express individual identity. The study of Liu et al. demonstrated the impact of 3 types of symbols on BEVs’ adoption intention [55]. Particularly, the status symbol stands for the perceived social position granted by owning a car, which is considered as a luxury product. Meanwhile, environmentalism symbol creates a positive image of environmental protection for the owners. Finally, the innovation symbol is associated with being innovative and advancing to the times.
4.1.2. Behavioral Factors
Travel distance and frequency: Lu et al. concluded that potential adopters of BEVs prefer short distance travelling (less than 40 km) because of BEVs’ limitation in range and lack of charging infrastructure [56]. Similarly, Chen et al. pointed out that long driving distance discourages people from purchasing a BEV [11]. Nazari et al. also reported that drivers who travel more frequently exhibit less interest in BEVs [58].
Environmentally friendly habits: Habit describes the extent to which individuals tend to conduct an act automatically based on previous behavior. In other words, past behaviors could predict how people behave in the future. In the case of BEVs, customers who have driven a BEV before show more interest in buying and using them [51]. Additionally, sustainable habits such as solar panel adoption, recycling, changing diets, carsharing, and other eco-friendly behaviors also have a spill-over effect on BEV adoption intentions [11, 37, 58, 59].
4.1.3. Product Attribute Factors
According to Table 2, environmental performance, range, charging time, price and cost are the most common factors in previous studies.
Environmental performance: Environmental performance of BEVs refers to their benefits towards the environment. Xu et al. stated that BEV usage can save natural resources and reduce gas emissions [26]. As more people are concerned about environmental issues, it is obvious that BEVs’ environmental performance is a positive predictor of adoption intention. According to Haustein & Jensen, although facing some technical drawbacks, the environmental performance of BEVs attracts purchase intention of customers as they could reduce noise pollution and local emissions and promote renewable energy sources [35]. In a study investigating the purchase intention of conventional car drivers, it was reported that environmental and economic attributes of BEVs are the determinants of purchase decisions, meanwhile, instrumental attributes play an insignificant role. It was explained that conventional car drivers who intend to buy a BEV as their next vehicle weigh instrumental attributes less critical than environmental performance [41]. However, in another research conducted by Berneiser et al., environmental performance was found to have a minor positive impact on purchase intention. This result was explained by the fact that some environmental benefits of BEVs are still questioned, for example, the production and disposal of batteries, recycle issues, and fossil fuel electricity sources [47]. In general, BEV adoption intention depends much on the public’s perception of its environmental performance.
Range: The range of BEVs refers to the distance of travelling after a full charge. In comparison to ICEVs, BEVs are limited by battery capacity, thus, they have a shorter cruising range. Range concern has been an inhibitor preventing the customer from purchasing BEVs. A recent survey reported that the BEV choice rate would improve from 46% to 81% if the cruising range were increased to the same as ICEVs [ 56 ]. Ščasný et al. found that Polish customers are willing to pay an extra EUR 1000 for each additional 100 km in the BEV range [ 62 ]. In a qualitative research interviewing 227 experts in transportation about barriers to BEV diffusion, range was the most mentioned factor [ 30 ]. Many interviewees stated the fact that BEVs are incapable of long trips. Although the current range of BEVs is sufficient for daily travel, it is difficult for customers to accept a vehicle that cannot serve all trip purposes.
Charging time : Charging time refers to the time needed to fully charge a BEV. Chen et al. surveyed 4885 respondents in 5 Nordic countries and found that 87% of participants considered charging time an important attribute of BEV [ 11 ]. The study also showed a positive relationship between shorter charging time and adoption intention. Li et al. pointed out that an ICEV only takes 5 minutes to refuel, the normal charging time of a BEV is about 8 hours. The availability of a quick charge option reduces the charging time to 1 hour, but it is still a barrier to adoption intention [ 25 ].
Price and cost: Customers are less likely to adopt BEVs if they do not have the ability to pay. The research of Jreige et al. demonstrated a negative relationship between adoption intention and purchase price and driving cost [ 60 ]. Li et al. stated that as the cost per kilometre of electricity is cheaper than gasoline, BEVs offer lower fuel costs as compared to ICEVs, which is a positive feature attracting customer intention [ 25 ]. Krishnan & Koshy posited that high purchase prices and battery costs are preventing customer’s intention to adopt BEVs; however, the authors also noted that low fuel and maintenance costs are advantages of BEVs [ 43 ].
4.1.4. Contextual Factors
Charging infrastructure: Since BEVs depend solely on electricity, charging infrastructure plays an important role in adoption intention. Jia & Chen proved that the availability of charging infrastructure (including charging stations along highways, at the workplace, and in local areas) can positively affect adoption intention [57]. Compared to conventional vehicles, BEVs take a longer time to refuel/recharge, which is inconvenient while driving. Thus fast charging technology and fast charging station are necessary to increase the adoption intention. The research of Ščasný et al. demonstrated that each additional hour of charging time decreases the willingness to pay by EUR 300 per vehicle; meanwhile, fast charging station availability increases the willingness to pay by EUR 2000 per vehicle [62].
Monetary incentive policies: The term refers to financial incentive policies. Xu et al. suggested that these policies could include purchase subsidies, tax exemptions, preferential insurance policies, and allowable loan amounts for BEVs [26]. Lu et al. recommended tax reduction, purchase subsidy, and vehicle use subsidy as measures to improve adoption intention in the context of China [56]. Similar effect of monetary incentive policies is found in the US [57] and South Korea [48]. Additionally, personal carbon trading (PCT) influences adoption intention positively [25]. PCT is a policy that imposes a fixed credit on personal carbon emissions and allows individuals to trade their unused credits. Since BEV adopters have fewer carbon emissions, they spend fewer emission credits. As a result, they can sell the remaining credits to people with excessive needs in exchange for financial benefits. Li et al. showed that PCT is more effective than other monetary policies such as carbon tax, tax exemption, and charging discount [24].
Non-monetary incentive policies: Lu et al. examined the effect of restriction policies on BEV adoption intention in China and confirmed the negative impact of license plate restrictions and driving restrictions [56]. Currently, these policies are only applied to conventional vehicles, but if they are applied to BEVs, the choice rate will drop from about 46% to less than 25%. Additionally, bus line driving could be a good alternative for financial subsidies as it offers BEV owners more convenience during peak hours [56]. Meanwhile, public parking benefits such as priority lots and free parking fees could also enhance adoption intention [48].
Marketing, distribution and after-sales service: Few studies explored the impact of marketing, distribution, and after-sales services on the acceptability of BEVs. Krishnan & Koshy concluded that manufacturers and distributors should develop more showrooms and service centers, reinforce a strong brand image through advertising and other marketing activities, and improve postsales services to increase the adoption intention [43].
4.1.5. Demographic Factors
Age: Most studies suggested that young people tend to express a higher intention to adopt BEVs. For example, the research of Jia & Chen indicated that people under 35 are more interested in BEVs as they have a favorable attitude towards new technologies such as BEVs [57]. Lu et al. pointed out that adoption intention decreases with age [56]. However, Kim et al. found that people in the old age group have a higher intention to adopt BEVs in South Korea [48]. Therefore, the impact of age might vary among countries.
Education: All selected papers agreed on the positive impact of high education on BEVs adoption intention [48, 56-58]. Lu et al. found that people with a graduate degree show more interest in BEVs than others [56]. Compared to conventional vehicle owners, BEV owners have higher educational attainment (bachelor’s degree or higher). Kim et al. reported that people holding a high school degree or below are less likely to adopt BEVs [48]. It seems that people with high education are better aware of environmental issues, thus, they are more likely to take action to protect the environment.
Vehicle ownership: The study of Lu et al. in China revealed that people who have owned a vehicle tend to purchase BEVs [56]. The authors explained that as most vehicles are conventional and subjected to driving restriction policies, people adopt BEV as a supplement for travelling. In addition, Nazari et al. concluded that people who have already owned a BEV or HEV are more likely to purchase another BEV as they have more experience and knowledge [58].
Gender: Most researchers show that BEVs are products for men except for the study of Kim et al. [48]. However, most papers do not clearly explain the association between gender and BEV adoption intention.
Income: In Nordic countries, Chen et al. found that high income is positively associated with adoption intention as BEV purchase price is quite high [11]. In Germany, Berneiser et al. also reported similar results, this is due to the expensive purchase price of BEVs compared to conventional vehicles that only people with medium to high income can afford [47]. However, Lu et al. found that people with low income (less than USD 28.000 per year) are more likely to adopt BEVs in China [56]. The underlying reason might be due to the great BEV subsidies in China that make it more popular and affordable for low-income people.
4.2. Determinants of BEV Adoption Behavior
4.2.1. Psychological Factors
Zhou et al. identified 2 psychological predictors of BEVs adoption behavior, including intention and satisfaction with incentive policies [51]. Intention refers to people’s commitment to action, thus as intention becomes stronger, the actual behavior is more likely to occur. Intention is one of the most important predictors of behavior, which was previously verified by many studies. Satisfaction with incentive policies is the extent to which drivers compare the perceived to the expected value of incentive policies. The authors argued that it is satisfaction that increases the adoption rate, not the policies themselves.
The study of Brückmann et al. found 3 psychological factors affecting the adoption behavior of BEVs, namely, technology affinity, green party preference, and environmental awareness [64]. Technology affinity stands for human technological enthusiasm, as BEVs are innovative products tech-enthusiasts are more likely to adopt. Regarding green party preferences, voters who prefer pro-environment parties have a higher chance of being adopters of BEVs. Finally, people who exhibit a strong sense of environmental consciousness tend to adopt BEVs in reality.
4.2.2. Behavioral Factors
Car sharing: The study of Brückmann et al. investigated the impact of some pro-environment travel behaviors on BEVs actual adoption [64]. The results showed that the act of carsharing significantly improves BEVs adoption behavior. However, the same effect could not be confirmed in the case of public transport subscription as it is statistically insignificant. The study called for further research on particular behavioral factors that might lead to BEVs actual adoption.
4.2.3. Contextual Factors
Charging infrastructure: Similar to intention, charging infrastructure plays a major role in determining adoption behavior. Based on data at the county level in Virginia (US), Jia & Chen documented that the density of charging points is highly correlated with the number of BEV registration numbers [57]. The same result was found in China [65] and Sweden [66], in which the availability of public charging increases the actual adoption of BEVs. However, Mukherjee & Ryan reported that a long distance to the nearest public charging stations positively influences BEV adoption in Ireland, although the effect is quite small [32]. According to the authors, public charging stations are likely located in nonresidential areas while actual adopters live in the suburbs. Additionally, as most BEV drivers charge at home, they do not need nearby public charging stations.
Monetary incentive policies: Kalthaus & Sun reported that a 1% raise in monetary subsidies could lead to a 0.5% increase in newly registered BEVs [65]. In addition, Narassimhan & Johnson reported that a 1% raise in tax incentives results in a 1.15% increase in BEV purchases [67]. In general, subsidies and tax incentives are useful tools to boost BEV adoption in reality.
Non-monetary incentive policies: The research of Kalthaus & Sun examined the effect of 2 particular nonmonetary policies in favor of EV drivers, which are exemptions from license-plate lotteries and auctions [65]. The result showed that the aforementioned policies could promote the diffusion of BEVs with substantial effect. Egnér & Trosvik suggested that public procurement policy should be taken into consideration [66]. Particularly, the authors proved that municipalities owning more BEVs also witness a higher number of personal-owned BEVs. Therefore, BEVs public procurement policy should be set for municipalities so that they become lead users to spread the adoption of BEVs among community members.
4.2.4. Demographic Factors
Age: Jia & Chen found a discrepancy in the impact of age on stated intention and actual adoption behavior [57]. In particular, according to the vehicle choice experiment, young respondents (below 35 years old) are more likely to express the intention to adopt BEVs. However, according to the real-world EV registration data, old individuals (above 55 years old) are more likely to own BEVs. Mukherjee & Ryan reported similar results in which young age from 19 to 34 negatively influences adoption behavior [32]. A possible explanation is that young individuals might have low incomes; thus, they are less likely to own BEVs in reality.
Gender: Gender shows a consistent effect on both adoption intentions and behavior. Men state a greater interest in BEVs [57], while women show less preference [64].
Education: High education is a positive predictor of BEVs adoption behavior. People holding bachelor’s degrees or higher tend to own BEVs [57]. Mukherjee & Ryan implied that these individuals can afford BEVs as they achieve higher income and social class [32].
Accommodation ownership: Brückmann et al. stated that moving from rented accommodation to an owner-occupied flat or an owned house can increase the adoption chance by 10% and 12%, respectively [64]. Mukherjee & Ryan explained that rented accommodations usually do not offer secure parking space for charging as well as indoor charging infrastructure, thus, it is less likely for people living in a rented house to own a BEV [32].
CONCLUSION
This review has selected and reviewed 45 out of 3511 articles that are relevant to the topic of BEV adoption determinants. In general, most studies are conducted in global EV leading markets such as China, Europe, and the US. The number of articles has been rising recently, which indicates greater interest in exploring BEV adoption. However, for future research, more studies are needed to promote the adoption of BEVs in less developed markets where environmental issues are causing great concern. Additionally, a few cross-border studies were identified that might suggest a potential research topic related to the impact of culture on BEV adoption.
This study has identified various determinants of BEV adoption and categorized them into 5 groups, namely, psychological, behavioral, product attribute, contextual, and demographic factors. Furthermore, as a gap exists between intention and behavior, this study has separated factors affecting adoption intention and actual behaviors.
Overall, most research papers focused on investigating adoption intention by extracting self-reported data in surveys. Many respondents are not actual owners or drivers of BEVs, thus, their product knowledge is limited and their stated intention does not necessarily lead to adoption behavior in reality. Among the predictors of adoption intention, the psychological category is dominant with popular factors such as attitude, social influence, perceived behavioral control, motivation, experience, and symbols. In terms of behavioral factors, travel distance and frequency negatively affect interest in BEVs, while other environmentally friendly habits are positive predictors. In addition, the intention to adopt BEVs is strengthened if some drawbacks related to product attributes are improved, such as range, charging time, price, and cost. BEVs adoption intention is also dependent on contextual factors such as charging infrastructure, supporting policies, marketing, distribution, and after-sales services. Regarding demographic factors, potential adopters of BEVs are likely to be young males with high education and income.
In contrast, BEVs actual adoption is a potential topic for future research as it is limitedly explored by previous research. Many studies investigating actual adoption determinants utilize real-world data or surveying actual BEV drivers. There are only 4 groups of determinants, including psychological, behavioral, contextual, and demographic factors. Perhaps the product attribute category only serves as input information, which is necessary for product evaluation to form an intention, therefore, they do not directly influence the actual behavior. The most important psychological factor is intention, as it directly guides actual behavior. The impact of behavioral factors on adoption behavior is not fully explored as it is only mentioned in 1 article. Compared to adoption intention, similar contextual and demographic factors are identified except for age. Based on real-world data, it is reported that young people could express higher intentions, but old individuals are more likely to be actual adopters of BEVs since they possibly have higher incomes.
Finally, the authors also acknowledge some limitations of this review. The study only considers English articles from a limited number of databases such as SCOPUS, Science Direct, Emerald, Sage, Wiley, Taylor & Francis and Springer. Therefore, articles from other databases or written in other languages were not analysed. Additionally, in order to gather up-to-date data, only articles published from 2017 to 2021 were reviewed; future research can extend the timeframe to collect more robust data.
LIST OF ABBREVIATIONS
BEVs | = Battery Electric Vehicles |
GHG | = Greenhouse gas |
ICEVs | = Internal Combustion Engine Vehicles |
EVs | = Electric Vehicles |
HEVs | = Hybrid Electric Vehicles |
SEM | = Structural Equation Modelling |
CONSENT FOR PUBLICATION
Not applicable.
STANDARD OF REPORTING
PRISMA Guideline were followed.
AVAILABILITY OF DATA AND MATERIALS
The data supporting the findings of the article is available in the Zenodo at https://doi.org/10.5281/zenodo.7066477, reference number 7066477.
FUNDING
None.
CONFLICT OF INTEREST
The author declares no conflict of interest, financial or otherwise.
ACKNOWLEDGEMENTS
Declared none.
SUPPLEMENTARY MATERIAL
PRISMA checklist is available as supplementary material on the publisher’s website along with the published article.
APPENDIX.
Source | Intention | Behavior | Research method | Positive variables | Negative variables |
Xu et al. (2019) [26] | X | - Quantitative - SEM - 382 customers in Zhejiang Province, China |
-Attitude -Perceived behavioral control -Subjective norm -Environmental performance - Monetary incentive policy |
||
Lu et al. (2020) [56] | X | - Quantitative - Binary Logit Model - 900 participants plan to buy cars in Beijing, China |
- Charging convenience - Cruising Range - Purchase subsidy - Vehicle use subsidy - Bus line driving permit - Age (Young) - Education (high) - Travel distance (short) - Vehicle demand (urgent) - Vehicle Ownership (already own vehicles) - Income (low) |
- License plate restriction - Driving restrictions - Purchase tax - Income (high) |
|
Jia & Chen (2021) [57] | X | X | - Quantitative - Mixed logit model - 837 drivers in Virginia, US & data from Department of Motor Vehicles |
Intention: - Age (Young) - Gender (male) - Education (high) - Charging infrastructure - Purchase incentive (tax credit & State rebates) - Range - Income (high) - Vehicle demand (subcompact/compact car) Behaviour: - Age (old) - Gender (male) - Education (high) - Charging infrastructure - Household size |
Intention: - Purchase price - Cost - Travel distance (long) Behavior: - Income (high) |
Chen et al. (2020) [11] | X | - Quantitative - Hierarchical regression - 4885 adults living in Demark, Finland, Iceland, Norway and Sweden |
- Demographics (young, men, higher-income, higher number of children) - Financial attributes (expectation of car cost, intention to buy a new car) - Mobility practices (Short driving distance, EV experience) - Electric mobility attributes (shorter charging time, V2G capability) - Conventional vehicle attributes (ease of operation, technical reliability, fuel economy) - Sustainability values (energy efficiency, solar panel adoption, recycling, changing diets, high environmental values) |
||
Brückmann et al. (2021) [64] | X | - Quantitative - Generalized linear mixed-effects logistic model - 5325 owners in Switzerland |
- Income (high) - Cars per household (high) - Usage of carsharing - House ownership - Technology affinity - Green party preference - Environmental awareness |
- Gender (female) - Age (old) |
|
Hoerler et al. (2021) [59] | X | - Quantitative - Binary logistic model - 823 Swiss respondents |
- Car Sharing experience | ||
Zhou et al. (2021) [51] | X | X | - Quantitative - SEM - 725 Chinese drivers |
Intention: - Incentive Policies - Performance Expectancy - Effort Expectancy - Facilitating Conditions - Hedonic Motivation - Price Value - BEV driving habit Behaviour: - Incentive Policies - Facilitating Conditions - Intention |
|
Müller (2019) [33] | X | - Quantitative - PLS-SEM - 1177 respondents from EU, North America and China |
- Attitude | ||
Kim et al. (2019) [48] | X | - Quantitative - Binary choice model - 924 South Korean drivers |
- Prior experience - Age (old) - Gender (female) - BEV Knowledge - Public Parking - Incentive |
- Education (low) | |
Rezvani et al. (2018) [27] | X | - Quantitative - SEM - 573 car owners in Sweden |
- Gain motivation - Normative motivation - Hedonic motivation |
||
Jreige et al. (2021) [60] | X | - Quantitative - Mixed logit model - 400 household in Lebanon |
- Range - Car Ownership (2 or more) |
- Purchase Price - Driving Cost |
|
Degirmenci (2017) [31] | X | - Qualitative & Quantitative - Interview & SEM - 40 end user for interview 167 surveys with test drive participants in Germany |
- Attitude | ||
Kalthaus & Sun (2021) [65] | X | - Quantitative - Generalized Method of Moments (GMM) dynamic panel estimations - 31 regions in China |
- Financial incentive - Regulatory incentive - Availability of charging infrastructure |
||
Schmalfuß et al. (2017) [40] | X | - Quantitative - Path analysis - 286 German participants with driving license |
- Experience - Subjective norm - Perceived behavioral control |
||
Pradeep et al. (2021) [28] | X | - Quantitative - Multiple regression - 385 respondents in India |
- Attitude - Perceived technology - Perceived behavioral control |
- Instrumental attribute | |
Franke et al. (2017) [61] | X | - Quantitative - Regression - 72 respondents in Germany |
-Range satisfaction | ||
Li et al. (2018) [25] | X | - Quantitative - Random parameter logit model - 928 responses in China |
- Personal Carbon Trading policy - Range (long) - Charging station - Financial incentive policies |
- Fuel cost - Emissions - Charging time - Purchase price |
|
Egnér & Trosvik (2018) [66] | X | - Quantitative - Regression - Panel data 2010-2016 from Swedish participants |
- Charging points - Public procurement policy |
||
Ščasný et al. (2018) [62] | X | - Quantitative - Mixed logit model - 2156 respondents who intend to buy a car |
- Range - Fast charge station - Free parking - Free public transport policy |
- Charging time - Operating cost - Purchase price |
|
Krishnan & Koshy (2021) [43] | X | - Quantitative - SEM - 1440 Indians owning conventional vehicle |
- Perceived benefits - Social Influence - Technological consciousness - Price - Marketing, distribution and aftersales service |
- Perceived performance barriers - Policy implications |
|
Carley et al. (2019) [44] | X | - Quantitative - OLS Regression - Longitudinal survey data (2302 individuals in 2011 and 2119 individuals in 2017) |
- Relative advantage - EV experience - Social influence - Charging station |
||
van Heuveln et al. (2021) [29] | X | - Qualitative - Semi-structure interview - 20 EV drivers in Netherland |
- Attitude - Subjective norm - Perceived behavior control |
||
Ackaah et al. (2021) [34] | X | - Quantitative - SEM - 404 experienced drivers in Ghana |
- Attitude - Subjective norm - Perceived behavioral control |
||
Mukherjee & Ryan (2020) [32] | X | - Qualitative &Quantitative - Focus group Interview & Count data econometric models - 18 participants for interview & 1680 BEV owners in Ireland |
- Education (High) - Distance to nearest charging point - Commuting time (long) |
- Accommodations (rented) - Age (Young) |
|
Haustein & Jensen (2018) [35] | X | - Quantitative - Linear regression - 673 BEV owners and 1794 CV owners in Demark and Sweden |
- Attitude - Subjective norm - Satisfaction with environmental performance |
- Perceived functional barriers | |
Li et al. (2017) [49] | X | - Quantitative - SEM - 940 consumers in Jiangsu Province, China |
- Perceived return - Past experience |
- Perceived risk | |
Yang & Chen (2021) [45] | X | - Quantitative - Mixed logit models - 934 respondents in China |
- Social influence - Innovativeness |
||
Liu et al. (2020) [36] | X | - Quantitative - Path analysis - 347 Chinese drivers |
- Subjective norm - Driving experience - Perceived behavioral control - Attitude towards cruising range - Attitude towards battery life - Attitude towards low emission - Attitude towards low noise |
||
Kowalska-Pyzalska et al. (2021) [37] | X | - Quantitative - Ordinal logit regression model - 983 Polish respondents |
- Interest in new technologies - Eco-friendly Attitude and behaviors - Charging infrastructure - Brand recognition - Gender (male) |
||
Qian & Yin (2017) [53] | X | - Quantitative - SEM - 948 respondents in China |
- Deontological evaluation | - Risk aversion | |
Khazaei & Tareq (2021) [46] | X | - Quantitative - SEM - 322 people know about BEVs in Malaysia |
- Social influence - Facilitating conditions - Perceived Enjoyment - Environmental Concern |
- Range anxiety | |
Simsekoglu & Nayum (2019) [41] | X | - Quantitative - Hierarchical regression analysis - 205 respondents in Norway |
- Environmental-economic attributes - Subjective norm - Perceived behavioral control |
- Gender (male) | |
Brinkmann & Bhatiasevi (2021) [54] | X | - Quantitative - OLS Regression - 195 young adults In Thailand |
- Environmental consciousness | - Price | |
Nazari et al. (2019) [58] | X | - Quantitative - Binary Probit Model & Log-linear regression - 1249 people intend to buy an additional vehicle in the US |
- Gender (Male) - Education (high) - Ethnicity (White and Asian) - Income (High) - Home charging - Solar panels purchase - BEV ownership (already have BEVs) - Ridesharing users - Important vehicle attributes |
- Studentship - Driving frequency - BEV concern attitude |
|
Hinnüber et al. (2019) [50] | X | - Quantitative - Wilcoxon test - 114 German test drive participants |
- First time drive experience | ||
Wang et al. (2021) [38] | X | - Quantitative - SEM - 261 potential buyers in China |
- Attitude - Financial incentive policies - Environmental concern - Face consciousness |
- Information provision policies - Convenience policies - Social influence |
|
Liu et al. (2021) [55] | X | - Quantitative - SEM - 347 experienced drivers in China |
- Status symbol - Environmental symbol - Innovation symbol |
||
Narassimhan & Johnson (2018) [67] | X | - Quantitative - Regression - EV sales from 2008 – 2016 in the US |
- Tax incentives - Charging infrastructure |
||
Berneiser et al. (2021) [47] | X | - Quantitative - Hierarchical regression - 1922 participants in Germany |
- Personal experience - Subjective norms - Collective efficacy - Technological risk attitude - Perceived information - Environmental benefits of EV - Income |
- Age (Young) | |
Noel et al. (2020) [30] | X | - Qualitative - Semi-structured expert interview - 227 participants from Denmark, Finland, Iceland, Norway and Sweden |
- Knowledge and experience - Charging infrastructure - Range |
- Price | |
Dong et al. (2020) [42] | X | - Quantitative - SEM - 1021 households in China |
- Feelings and emotion - Subjective norms - Perceived Behavioral Control - Personal norms |
||
She et al. (2017) [63] | X | - Quantitative - SEM - 162 Chinese trainees in driving schools |
- Vehicle performance barrier - Infrastructure barrier - Financial barrier |
||
Jiang et al. (2021) [52] | X | - Quantitative - SEM - 332 respondents in China |
- Brand image - Brand awareness - Brand identity |
- Perceived risk | |
Du et al. (2018) [39] | X | - Quantitative - Hierarchical Regression - 811 residents of Tianjin, China |
- Attitude - Subjective norms - Perceived behavioral control - Personal norms - Government policies |
||
Li et al. (2019) [24] | X | - Quantitative - Discrete choice model - 458 Chinese respondents |
- Carbon tax - Carbon trading |