All published articles of this journal are available on ScienceDirect.
The Method of Multi-criteria Evaluation of the Effectiveness of the Integrated Control System of a Highly Automated Vehicle
Abstract
Objective
A multi-criteria approach was developed to assess the effectiveness of the implementation of on-board information and control systems that reflect the impact of the implemented equipment in the control system of a highly automated vehicle, taking into account the establishment of their indicators.
Methods
A multi-criteria approach is used to determine the rational composition of the on-board information and control system, taking into account the weight coefficients and limitations of transport systems.
Results
Based on the model of integration of the intelligent on-board information and control system of the vehicle into the transport infrastructure, the criterion of controllability is determined - the coefficient of efficiency of vehicle management.
Conclusion
The results of research shows that the controllability of the vehicle increases by 14.3% when the automatic function of connecting manual control is introduced into the on-board information and control system, on the basis of which the dependences of calculating the critical distance and time when driving an unmanned vehicle are clarified.
1. INTRODUCTION
The transition to a new level of autonomy will significantly improve the safety and efficiency of vehicle use. Assigning a number of tasks to the onboard information and control system (ICS) requires an increased level of reliability of automotive control systems, which requires the installation of uniform requirements for the design and operation of electronic systems responsible for the unmanned vehicle [1, 2]. The ICS provides information on the interaction between the systems inside the vehicle and elements of the road infrastructure. The full-fledged implementation of the ICS into the vehicle is a complex task, focused primarily on ensuring control over each individual system. The technology will allow for the controllability of the vehicle, as well as monitoring its functioning through embedded sensors [3-5]. The creation of unmanned logistics corridors has great transport potential, especially for cargo vehicles. This will allow cargo vehicles to receive complete information about road traffic - speed, distance, obstacles on the way, etc.; therefore, it is more accurate to determine the required actions when driving [6]. However, for the mass introduction of unmanned transportation, it is necessary that not only should the vehicle be equipped with on-board information and control systems, but also the entire road infrastructure should be equipped with sensors for information exchange with the vehicle [7]. The degree of interaction between the vehicle and the transport infrastructure determines the level of connection of the vehicle (Fig. 1).
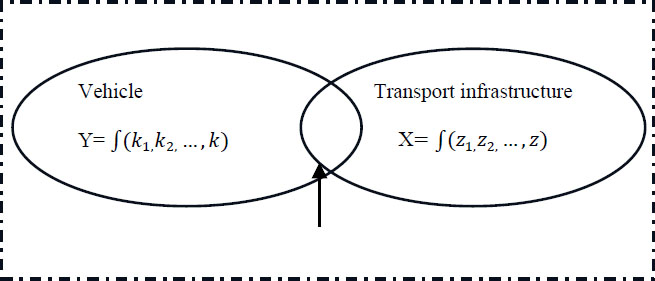
The degree of connection of the vehicle to the transport infrastructure, where K1, K2, …, Kn – factors affecting the connected vehicle, Z1, Z2, …, Zn – factors affecting the connected transport infrastructure.
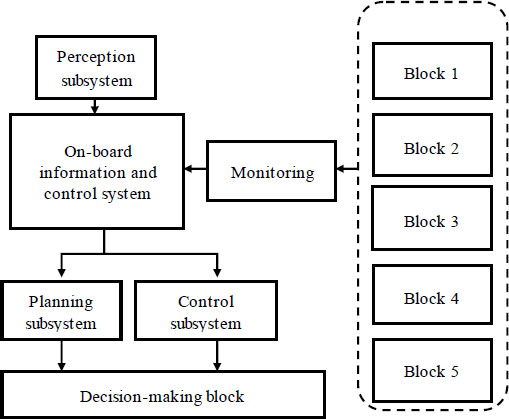
The principle of interconnection of subsystems of the on-board information and control system.
The interaction and management of the systems are carried out using on-board information and control systems. At the moment, there remains an undeveloped methodology for selecting the rational composition of the ICS to achieve maximum efficiency in the use of vehicles. It also requires the creation of tools to assess the potential impact of implemented systems on vehicle properties. One of the possible options for evaluating the effectiveness of the implemented electronic systems is the use of a multi-criteria approach when choosing the composition of the ICS [8-10].
The development of unmanned technologies should be accompanied by the possibility of automated authenti- cation of each vehicle, big data privacy technologies, and the transfer of big data at the required speed. These types of researches can be studied in more detail in the articles [11-14].
The on-board information and control system consists of a perception subsystem, a planning subsystem, and a control subsystem. Through the subsystem of continuous monitoring, the on-board information control system receives information about the technical condition of each vehicle system, transmits it to a computing mechanism, and verifies the received vehicle parameters with nominal values. The system can adjust the operation of individual components and assemblies, as well as transmit all necessary information to the driver in real-time (Fig. 2).
For the efficient operation of the transport system, each vehicle must be able to fully interact with the controls of the transport system. However, at the moment, such interaction of vehicles and transport infrastructure is not functioning at the proper level [15-17]. Therefore, it is proposed that the efficiency of vehicle use should be evaluated based on a multi-criteria approach that takes into account many factors. The application of a multi-criteria approach will make it possible to reasonably select on-board information and control systems and organize a system of interacting vehicles connected to the transport infrastructure. Additionally, the introduction of real-time monitoring of the condition of transport systems, automatic creation of workflow diagrams, and the possi- bility of remote workflow management will significantly reduce vehicle downtime by preventing malfunctions [18, 19].
2. MATERIALS AND METHODS
The introduction of onboard information and control systems into the vehicle equipment is aimed at increasing their efficiency. The use of new technologies to improve vehicle autonomy requires the creation of tools to assess the potential impact of various systems on vehicle performance. Furthermore, to select suitable onboard information and control systems, a multi-criteria approach is used, which determines the parameters that affect the efficiency of the vehicle (Fig. 3) based on a literary analysis. For each criterion, parameters affecting the technical condition of the vehicle are determined.
Moreover, to make a reasonable choice of the composition of onboard information and control systems, it is necessary to conduct a comparative analysis of the influence of the systems on the characteristics of the vehicle and select a complex in which each characteristic will be maximum. Determining the characteristics of each criterion and the most optimal composition of on-board information and control systems will improve the functionality of a vehicle.
Correctly defined ways of influencing each of the selected criteria will allow for the analysis and selection of the most optimal composition of onboard information and control systems to enhance the functionality of the vehicle in accordance with its specific operation. This takes into account how each system affects certain characteristics of the vehicle and what advantages it brings in different situations.
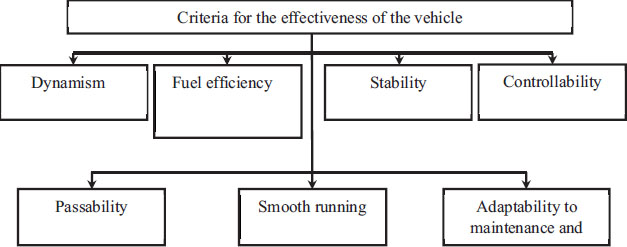
Parameters that affect the efficiency of the vehicle.
The integration of the onboard information and control system into the vehicle allows to collect, process, store, and transmit information about the status of vehicle systems in real-time to the control system. The elements of an intelligent transport and logistics system exchange data between themselves and external information receivers, which contributes to increasing the safety and efficiency of using vehicles.
Making the optimal decision is reduced to the deterministic task of forming the ICS software and hardware complex [20-22]:
![]() |
![]() |
(1) |
Where D is the set of acceptable values within which direct, functional and criteria constraints are fulfilled, represented as a general system of inequalities and equalities.
The proposed approach takes into account the weighted average of the selected criteria:
![]() |
(2) |
The weight coefficients determine the relative importance of individual criteria. They also characterize the sensitivity of the objective function to changes in certain particular criteria:
![]() |
(3) |
An additive multi-criteria approach is preferable when a decrease in the score for one criterion is compensated by an increase in the score for some other criterion (or several criteria) [23-25]. Furthermore, due to the heterogeneity of the selected criteria and the inability to bring them to a single formula due to different scales and units, it is necessary to bring the criteria to a single scale.
The method implies transformations that equalize the limits of changing criteria [26-29]. The most common transformation of this type is to replace the original criterion with a transformed criterion of the form:
![]() |
(4) |
where are the maximum and minimum values of the function on the set X, assuming, of course, that they exist.
However, since we will never go beyond the Pareto set when maximizing linear convolution with positive coefficients [2, 30], it makes sense to clarify the denominator of the normalized criterion as follows:
![]() |
(5) |
where - nadir is a vector that defines the coordinate boundaries from below the Pareto set, the degree of proximity to it and implements a certain distance from the “extreme” Pareto optimal points characterized by a maximum value according to one of the criteria.
Each of these transformations is strictly increasing, therefore, the Pareto set does not change with such a transformation, and normalization is applicable to the Edgeworth-Pareto principle and the subsequent application of linear convolution of the transformed criteria [31, 32].
The application of these principles makes it possible to equate the criteria to a single scale within a single numerical range, which simplifies the assessment of the effectiveness of automating the functions of connecting manual control of a vehicle [33].
The creation of tools for assessing the potential impact of implemented systems on vehicle properties by integrating an on-board information and control system into a vehicle collects, processes, stores and transmits information to the control system about the status of vehicle systems in real time [34-37]. The elements of an intelligent transport and logistics system evenly exchange information between transport and external information receivers, which contributes to improving transport safety and efficiency of vehicle use (Fig. 4) [38-41].
In addition, to have a complete understanding of which criteria are important for optimal vehicle performance and which systems affect these criteria, the user, can choose the most effective set of onboard information and control systems. This will improve the performance, safety, economy, and handling of the vehicle, depending on its specific use. The complexity of the task lies in the fact that, at the moment, there are a huge number of systems and developments that are not regulated in any way. That is why the study should begin with a comprehensive analysis of the possible impact of onboard information and control systems on various transport systems and the determination of the minimum composition of electronic control units.
The introduction of intelligent transport systems allows to influence and monitor the operation of individual vehicle systems. A highly automated vehicle must meet the level of intelligence required in accordance with the categories of automation (Table 1). The automatic steering connection function (ASCF) is a set of functions in the vehicle's electronic control system, in which the activation of the manual control system can occur as a result of automatic evaluation of signals initiated on board the vehicle, in combination with passive infrastructure elements, to ensure control in order to assist the driver [42, 43]. The UNECE has agreed on the classification of manageability into categories depending on the degree of automation. The ASCF of category E, in which the system performs maneuvers during the movement of the vehicle without the support of the driver, is closest to the required level of unmanned operation. However, currently, requirements have been developed only for category C ASCF.
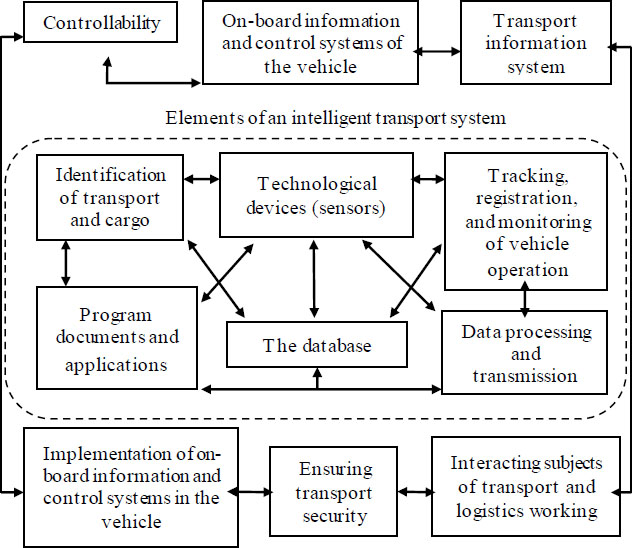
Model of the integrated intelligent onboard information and control system of the vehicle.
Category | Function | Efficiency Coefficient |
---|---|---|
A | It is triggered at a speed of no more than 10 km/h to assist the driver at his command when performing maneuvers at low speed or parking | Кef1 |
В1 | Helps the driver to keep the vehicle within the selected lane by affecting the lateral movement of the vehicle | Кef2 |
B2 | It is activated by the driver and keeps the vehicle within its lane, affecting the lateral movement of the vehicle for extended periods of time without further commands/confirmation from the driver | Кef3 |
C | It is activated by the driver and can provide one side maneuver (for example, lane change) at the driver's command | Кef4 |
D | It is activated by the driver and is able to indicate the possibility of performing one lateral maneuver (for example, changing lanes), but performs this function only after confirmation by the driver | Кef5 |
E | It is activated by the driver and continuously detects the possibility of maneuvering (for example, by changing lanes) and performs these maneuvers for extended periods of time for further commands/confirmation from the driver | Кef6 |
Therefore, to assess the controllability of vehicles of various categories, an efficiency coefficient has been determined, which should strive for the maximum value. Each coefficient is determined from the optimization conditions and exceeds the nominal value of the efficiency coefficient:
![]() |
(6) |
Address correspondence to this author at the Department of Transport and Technological Processes and Machines, Saint-Petersburg Mining University, Saint-Petersburg, Russia; E-mail: safravi@mail.ru
3. EXPERIMENTAL
The introduction of onboard information and control systems allows the user to control the operation of the manual control of the vehicle [44, 45]. The intelligent system recognizes in advance the movement of connected vehicles within the range of radar and lidar visibility. The principle of lidar operation is based on sensor technology. Radar sensors emit infrared light and determine the time of passage of light from an object to the sensor, creating a three-dimensional map from a cloud of points with geometry around an unmanned vehicle. The radar uses radio waves in the range of 77-79 GHz to record the distance to a transport infrastructure facility. The average radar visibility distance is 300-500 meters [45, 46]. Moreover, receiving information from radar, lidar, and cameras, the onboard information management system makes cartography of the area, including information about the surrounding infrastructure and other objects (Fig. 5) [47, 48].
The minimum distance required to overtake a vehicle is determined by the dependence:
![]() |
(7) |
where td is the reaction time of the driver (calculations usually take from 1.5 to 3 seconds), ln is the length of the vehicle, ls is the safety distance between them after stopping, V is the instantaneous speed of the car at the moment of braking, a is the deceleration (acceleration) of the car.
The average reaction time of unmanned transport systems due to radars and lidars is up to 0.14 seconds. Thus, depending on the reaction time of the driver, td is equated to 0 and takes the form:
![]() |
(8) |
where tc is the reaction time of the vehicle systems.
The deceleration scheme of vehicles is shown in the Fig. (6):
The efficiency increase was obtained based on changes in road capacity. With the introduction of on-board information and control systems, the time required for the driver's reaction (for example, when starting traffic at intersections or when performing a maneuver) is reduced from 2 seconds to 0.14 seconds, which are necessary for sensors to read the road situation and make a decision.
![]() |
(9) |
Therefore, the efficiency coefficient of the on-board information and control system (Fig. 7) is taken as the ratio.
Кef = 14,3%.
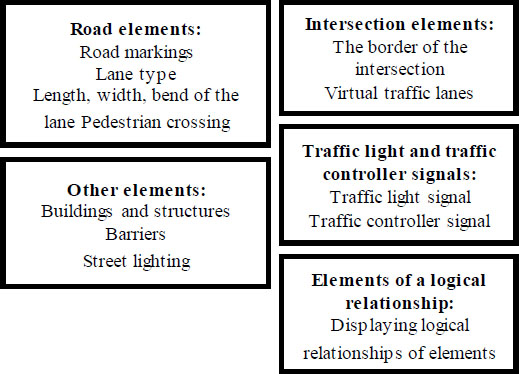
Transport infrastructure facilities and other elements required by the vehicle.
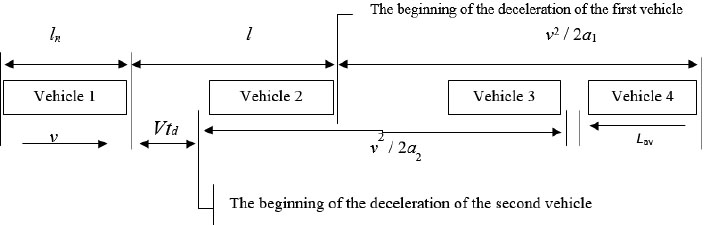
Braking scheme of vehicles during overtaking manoeuvre.
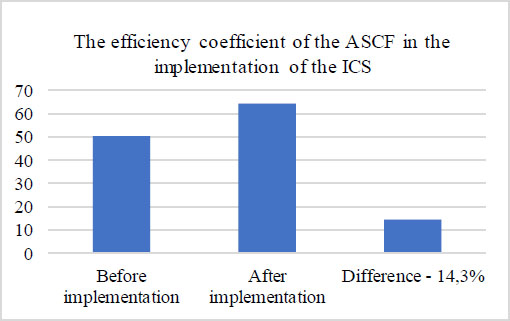
Comparative assessment of the efficiency coefficient of the ASCF in the implementation of the ICS.
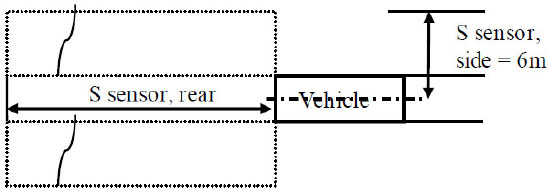
The detection area of the vehicle’s ICS.
4. RESULTS AND DISCUSSION
Furthermore, to be able to perform maneuvers automatically, the perception subsystem of the onboard information and control system must scan the environment and detect approaching and distancing vehicles in real-time. The detection area of the system should be at least 55 meters (Fig. 8).
A built-in servo system is used to automate the steering of the car. During normal operation, the servo system receives a voltage signal proportional to the measured torque applied by the driver. Driver assistance systems operate under the control of the driver and provide him with various features and functions for more comfortable and safe driving.
The situation is considered critical if, at the beginning of the maneuver, a vehicle approaching along the adjacent lane brakes with a deceleration of more than 3 m/s2 0.4 seconds after the start of the lane change maneuver in order to ensure such a distance between two vehicles that would not be less than the distance that the vehicle moving to another lane, it takes 1 s. The critical distance during the maneuver is calculated:
![]() |
(10) |
where: vrear = the actual speed of the approaching vehicle or 130 km/h, whichever is lower; vACSF = the actual speed of the vehicle with ASCF; a = 3 m/s2 (deceleration of the approaching vehicle); tB = 0.4 s (the time after the start of the lane change maneuver at which the deceleration of the approaching vehicle begins); tG = 1 s (the distance remaining between the vehicles after the deceleration of the approaching vehicle).
The minimum operating speed to which the vehicle performs the maneuver is calculated using the formula:
![]() |
(11) |
where Srear is the minimum distance specified by the manufacturer, in [m]; Vapp = 36.1 m/s (the speed of the approaching vehicle is 130 km/h, i.e. 36.1 m/s); Vsmin in [m/s] = the resulting minimum speed for activating the ASCF of category C.
Additionally, to increase the efficiency of cargo transportation, it is necessary to use intelligent transport systems that will automate the interaction of vehicles with infrastructure. Every aspect of driving a vehicle should be autonomous, but at the moment, this practice is just starting to gain momentum [49]. Similarly, for optimal selection of information and control systems on board the vehicle, it is recommended to use a multi-criteria approach. This contributes to a better interaction between vehicles and the overall infrastructure. Continuous monitoring of vehicle systems with the possibility of remote control helps to prevent downtime and malfunctions. The integration of onboard information and control systems will require the creation of tools to quantify their impact on vehicle performance. Based on the conducted research, further work is planned to specify criteria that can be used to unify requirements for vehicle on-board equipment.
The creation of autonomous logistics routes represents significant potential for the transportation industry, especially for freight vehicles. This will enable trucks to receive up-to-date information about road conditions - speed, distance to obstacles, etc., which will allow them to make more accurate decisions while driving. However, for the widespread introduction of autonomous transpor- tation, it is necessary that not only vehicles are equipped with onboard information and control systems, but also the entire road infrastructure is equipped with sensors for data exchange with the vehicle.
At the moment, one of the unresolved problems on public roads remains the lack of a unified information base reflecting the general condition of the selected territory and the analysis of the operation of each individual vehicle. The comprehensive implementation of the on-board information management system will allow the collection of information into a single system and influencing management systems, which are divided into 3 main blocks (Fig. 9):
1. Configuration of control scenarios, which displays the operation of the systems, forecasting the movement and technical condition of the vehicle, as well as the situational control mode.
2. Digital Environmental System - Environmental assessment report.
3. An automated traffic management system that includes information about the state of the road network.
This approach has great potential to improve the control of the situation on the road [50-52].
Similar developments are also expected in the VANET system. The VANET (vehicle ad-hoc network) system is a wireless network technology that allows vehicles to exchange information with each other and with the traffic infrastructure. VANETE allows cars to share information with each other and with the traffic infrastructure. It uses wireless communication standards to establish communication and exchange data on position, speed, and traffic conditions. VANETTE contributes to improving safety and driving efficiency and can be used to create driver assistance systems and reduce environmental impact. VANET uses wireless communication standards such as IEEE 802.11p (wave-wireless access in vehicular environments) to provide communication between cars and other nodes on the network. The main characteristics and advantages of VANET technology:
4.1. Data Exchange
Vehicles can exchange information about the current road position, speed, obstacles, or emergency situations, which contributes to improving road safety and efficiency.
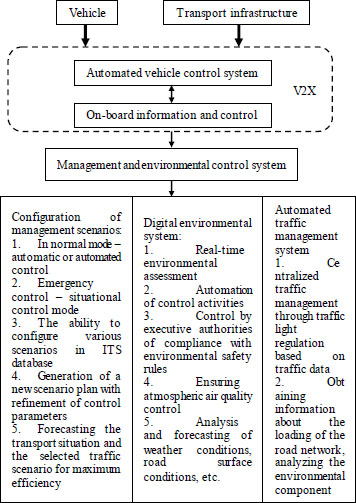
The scheme of data collection and transmission by means of an on-board information management system.
4.2. Traffic Improvement
Due to the possibility of obtaining data on the traffic situation and other traffic parameters, vehicles can choose the best routes and avoid congestion.
4.3. Driver Assistance Systems
VANET can be used to implement various driver assistance systems, such as emergency warnings, collision detection systems, and others.
4.4. Environmental Benefits
The use of VANET technology improves traffic flow management, which in turn can reduce emissions and improve the environmental situation.
In general, VANET technology plays an important role in the development of intelligent transport management systems and in improving road safety, but it also needs to be improved to create a unified information base that interacts with the transport infrastructure [53-55].
CONCLUSION
A multi-criteria method for evaluating the effectiveness of an integrated onboard information and control system for a vehicle has been developed, which allows to establish technical and operational indicators for the introduction of intelligent technologies in an automated vehicle control system. A model of integration of an onboard information and control system is presented, and a criterion for the effectiveness of controllability, time, and distances necessary for the curvilinear movement of a highly automated vehicle are determined. Based on the developed method, the calculation of increasing the efficiency coefficient of the automated steering function is presented. According to the calculations carried out, an increase in the automatic steering connection function on a highly automated vehicle was obtained - 14.3%.
AUTHORS' CONTRIBUTION
It is hereby acknowledged that all authors have accepted responsibility for the manuscript's content and consented to its submission. They have meticulously reviewed all results and unanimously approved the final version of the manuscript.
LIST OF ABBREVIATIONS
ICS | = Information and control system |
ASCF | = Automatic steering connection function |
AVAILABILITY OF DATA AND MATERIALS
The data and supportive information are available within the article.