All published articles of this journal are available on ScienceDirect.
Application of a Decision-making Approach Based on Factor Analysis and DEMATEL for Evaluating Challenges of Adopting Electric Vehicles
Abstract
Background
Electric vehicles (EVs) are important as they decrease air pollution, lessen reliance on fossil fuels, and significantly contribute to cutting greenhouse gas emissions. The use of EVs in promoting a sustainable life and reducing the harmful effects of climate change is very beneficial to the environment and public health.
Objective
The purpose of this research is to evaluate the potential challenges in the adoption of electric vehicles in Iran. To this end, a set of challenges is categorized, and the interrelation and significance of these challenges are examined.
Methods
To categorize the challenges of adopting EVs, exploratory factor analysis is used, and to evaluate the relationship between them and rank them based on their importance, the DEMATEL (Decision Making Trial and Evaluation Laboratory) method, which is a Multi Criteria Decision Making (MCDM) technique, is employed.
Results
The results of the statistical analysis show that challenges can be divided into three categories: social, economic, and technological. Furthermore, the DEMATEL method results indicate that the three most important and impactful challenges are as follows: two social challenges, “Lack of government support to promote EVs” and “Lack of public willingness and public resistance to adopting new EV technologies,” and one technological challenge, “Immature technological advancement in EVs and battery technologies.”
Conclusion
This research provides insights into evaluating the challenges of adopting electric vehicles. The findings assist decision-makers in developing electric transportation and understanding the relationships between challenges and their significance. This study serves as a foundation for policymakers and industry stakeholders to navigate through the complex landscape of electric vehicle adoption.
1. INTRODUCTION
Utilizing electric vehicles (EVs) is becoming incre- asingly crucial in response to environmental concerns. Global societies are searching for answers to address these issues due to growing awareness of climate change and the detrimental effects that greenhouse gas emissions have had on the environment in recent decades. Advancing the transportation sector’s efforts towards achieving carbon reduction and neutrality is crucial for speeding up the green and low-carbon shift within the industry, as well as promoting the sector’s high-quality growth [1, 2]. EVs have the potential to significantly reduce these effects because they run on renewable energy rather than fossil fuels. Lowering air pollution is one of the main benefits of driving an electric car [3, 4]. Since these vehicles produce no emissions and run on electricity rather than fossil fuels, they do not contribute to the greenhouse gas problem. In cities and other urban areas, this greatly reduces air pollution and improves air quality. Furthermore, there is a greater chance to lessen the negative environmental effects of EVs as attention turns to the production of electricity from renewable sources [5, 6].
Using EVs also has the benefit of lessening dependency on fossil fuels. The demand for oil and other fossil fuels will decline as the number of electric vehicles increases, which might lead to lower costs and less reliance on energy imports by nations. Electric vehicles are not just a mode of transportation for the present time; they are also crucial for the future of mobility and energy transition [7]. The rise of the electric vehicle industry stimulates innovation and opens up new job prospects in manufacturing, technology, and related industries. More- over, electric vehicles operate more quietly compared to conventional vehicles, contributing to reduced noise pollution in city areas [8]. EVs also have financial advantages. While they may cost more to buy upfront than conventional cars, over time, maintenance and operating expenses are reduced. These cost savings are due to the absence of the need for replacing moving engine parts, oil changes, and filter repairs, which are necessary for fossil fuel vehicles [9, 10]. The advancement and encouragement of electric vehicle use can contribute to the creation of healthier and more sustainable societies. People's health improves when pollution emissions are decreased and air quality is raised. Additionally, communities can become more robust to changes in the energy markets by lowering their reliance on fossil fuels. As a result, promoting and supporting the use of EVs is crucial for creating a sustainable and livable society in addition to being a significant step in combating climate change [11-13].
The adoption of EVs has grown to be a significant issue for governments and communities in today's progressive world. This problem is critical for the environment and for reducing reliance on fossil fuels, but it is also critical for the green economy and sustainable development [14-19]. There is no denying the importance of applying multi-criteria decision-making techniques along with sophis- ticated statistical methodologies to gain a comprehensive understanding of the problems and obstacles related to EV adoption in society [20-22]. Researchers and decision-makers can precisely and scientifically analyze and evaluate data and information related to EVs using statistical methods. These methodologies can offer a clear insight into consumers' habits, attitudes, and impediments to obtaining and using EVs. Statistical analysis also helps analyze the possible consequences of government policies, infrastructure facilities, and other environmental factors on EV adoption. Multi-criteria decision-making (MCDM) techniques are effective tools for handling the uncer- tainties and complexities involved with EV adoption decisions [23-25]. With the use of these techniques, it is possible to assess multiple crucial aspects at once, including sociocultural parameters, environmental advantages, economic utility, and the accessibility of charging infrastructure [26-30]. In order to facilitate and encourage the use of EVs in society, decision-makers can better identify priorities and create sustainable, balanced strategies by utilizing MCDM [31-36].
In this study, the DEMATEL (Decision Making Trial and Evaluation Laboratory) technique and exploratory factor analysis (FA) are used to establish a decision-making process that is based on accurate data and information, effectively considering and evaluating all relevant features and factors. This comprehensive and scientific approach can help decision-makers create policies and programs that can hasten the adoption of these cutting-edge technologies, providing them with a better understanding of the problems associated with EV adoption in society.
1.1. Contributions of the Study
1.1.1. An Innovative Categorization Approach
This research introduces an innovative approach by categorizing the challenges of adopting electric vehicles into three main areas: social, economic, and technological, using exploratory FA. This structured categorization offers a new perspective on understanding and addressing the multifaceted barriers to EV adoption.
1.1.2. An Application of the DEMATEL Methodology
The study employs the DEMATEL method to evaluate and rank the interrelations and significance of the identified challenges. This application of the DEMATEL method in the context of EV adoption challenges provides a detailed hierarchical understanding, assisting stake- holders in prioritizing their efforts effectively.
1.1.3. Identification of Key Influential Challenges
The findings pinpoint the most critical and impactful challenges: “Lack of Government support to promote EVs,” “Lack of public willingness and public resistance to adopting new EV technologies,” and “Immature techno- logical advancement in EVs and battery technologies.” This prioritization of key challenges offers new insights and actionable guidance for policymakers and industry stakeholders, making it a significant contribution to the field.
The remainder of the paper is structured as follows: In Section 2, a summary of recent research on the appli- cation of decision-making processes and approaches to the field of electric vehicles is provided. Additionally, the methodology based on DEMATEL and FA techniques is described in the same section. Section 3 presents the findings and discussions, while Section 4 includes the conclusions.
2. MATERIALS AND METHODS
Given the importance of evaluating the various challenges in adopting electric vehicles, this section first reviews some of the recent studies that focus on the application of decision-making approaches in related evaluation processes. Then, it presents and defines the challenges evaluated in this research. Finally, it details the methodology used in the study.
2.1. Recent Studies
In recent years, there has been a growing body of research focusing on the application of decision-making processes and approaches in the electric vehicle field. This subsection reviews the latest studies that examine the utilization of various decision-making models and strate- gies within the realm of electric vehicles. Next, the challenges utilized in the evaluation process of this study are outlined and explained.
Khan et al. [37] identified the most sustainable hybrid electric vehicle option for Pakistan. They suggested a transition from traditional internal combustion engine vehicles to hybrid EVs as a more feasible approach to cleaner transportation and employed an MCDM technique known as fuzzy Technique for Order Performance by Similarity to Ideal Solution (TOPSIS). They assessed and ranked various Hybrid EV options based on ten criteria across economic, social, and environmental dimensions. The paper emphasized the importance of governmental support in facilitating the shift toward hybrid technology. Wang et al. [38] aimed to develop a framework for selecting optimal locations for Battery Swapping Stations (BSS). Recognizing the pivotal role of the site selection decision in the life cycle of BSS, the study addressed a gap in current literature that largely focuses on operational and management issues of BSS rather than on the crucial pre-construction phase of site selection. The study utilized the fuzzy DEMATEL method to determine the weight of each criterion and the fuzzy Multi-Objective Optimization by Ratio Analysis (MULTIMOORA) approach for evaluation of the site alternatives. Guler and Yomralioglu [39] developed a robust approach for selecting optimal locations for EV charging stations. They used Geographic Information System (GIS) techniques, Analytic Hierarchy Process (AHP), and TOPSIS for the evaluation process. Sonar and Kulkarni [40] tackled the challenge of selecting the best EV option from the various models available in the market. The study introduced an innovative integrated method combining AHP and the Multi-Attributive Border Approximation Area Comparison (MABAC) method. The AHP method was employed to determine the relative importance of various criteria, and the MABAC method was utilized to evaluate and rank the selected EV alternatives.
Alosaimi et al. [41] categorized electric vehicles into several types, including Battery Electric Vehicles (BEVs), Fuel Cell Electric Vehicles (FCEVs), Hybrid Electric Vehicles (HEVs), Plug-in Hybrid Electric Vehicles (PHEVs), and Range-Extended Electric Vehicles (REHEVs), highlighting the improvements in their capabilities over recent years. The study used an integrated MCDM approach for selecting the most suitable type of EV given various challenges and considerations. Tarei et al. [42] employed a two-phased, hybrid MCDM approach. The first phase involved quantitative analysis using the Best-Worst Method (BWM) to rank and prioritize the barriers and sub-barriers to EV adoption. In the second phase, these prioritized sub-barriers were further analyzed using interpretive structural modeling (ISM) to understand the mutual relationships and dependencies among them. Ecer [43] selected ten EVs for evaluation using MCDM techniques. Simultaneous Evaluation of Criteria and Alternatives (SECA), Measurement of Alternatives and Ranking according to Compromise Solution (MARCOS), Multi- Attribute Ideal-Real Comparative Analysis (MAIRCA), Combined Compromise Solution (CoCoSo), Additive Ratio Assessment (ARAS), and Complex Propor- tional Assessment (COPRAS) were used for a detailed comparison based on technical specifications such as acceleration, price, battery capacity, range, etc. Following the individual assessment with each method, the research aggregated the results using the Borda count and Copeland ranking methodologies to create an overall ranking of the EVs. Mall and Anbanandam [44] used fuzzy AHP to evaluate different criteria affecting the choice among alternative charging technologies. This involved considering a multitude of factors, including but not limited to efficiency, cost, and availability, in a structured and quantifiable manner. The study utilized Vise- Kriterijumska Optimizacija I Kompromisno Resenje (VIKOR) to identify the best charging technologies based on the theoretical criteria for effectiveness.
Murugan and Marisamynathan [45] identified the key barriers to the adoption of electric vehicles (EVs) in India, taking into account both expert opinions and customer perspectives. The study employed two methodological approaches to analyze the data: the fuzzy DEMATEL method and the Relative Importance Index (RII) method. The fuzzy DEMATEL method was used to identify potential barriers based on expert opinions, while the RII method prioritized the barriers from customers' perspectives. Pradhan et al. [46] demonstrated the benefits of EVs and provided a model that helps customers make informed decisions when choosing an EV by evaluating vehicles against multiple factors. They used a fuzzy MCDM model combined with the Quality Function Deployment (QFD) model to determine the importance of various criteria based on customer feedback. Yang et al. [47] aimed at improving the process of selecting third-party reverse logistics (3PRL) providers for the electric vehicle power battery recycling process. The main goal was to develop a method to help electric vehicle battery manufacturers choose the best 3PRL providers to handle the pre-treatment and transportation of used batteries efficiently and safely. To address this complexity, the study introduced a new decision-making framework based on a linguistic Pythagorean hesitant fuzzy MULTIMOORA method. Elghanam et al. [48] developed an effective method for determining the best locations to install wireless charging lanes (WCLs) for EVs to address range anxiety among EV drivers. In the first stage, the TOPSIS method was used to assess and rank potential locations for WCL implementation. The locations that received the highest rankings in the TOPSIS stage were then evaluated using a binary goal programming model.
Zhao et al. [49] aimed to address two main issues concerning electric vehicles: the environmental impact of their energy sources and the logistical challenges of finding suitable locations for EV charging stations. The study employed a fuzzy DEMATEL method to determine the relative importance of the various criteria and a fuzzy MULTIMOORA method to rank the potential sites. The proposed MCDM approach was enhanced with GIS technology. Ghose et al. [50] focused on the need to switch to sustainable technologies for a healthier environment. The primary aim of the research was to determine the most suitable material for manufacturing solar vehicles. To tackle this complex issue, the study introduced a systematic methodology for selecting the optimal material. It involved a hierarchical analysis of 14 different properties across 19 potential materials using a hybrid MCDM method, specifically, the Fuzzy COPRAS model. Wei and Zhou [51] developed and applied a compre- hensive decision-making framework for government agencies and public bodies when selecting EV suppliers. To achieve this objective, the study first identified 14 critical factors that influence EV supplier selection. These factors spanned economic, social, environmental, and technical dimensions and were determined through a combination of literature review and expert opinions. With these factors established, the research then introduced an integrated MCDM framework. The framework combined the BWM and the fuzzy VIKOR approach to systematically evaluate and rank EV suppliers based on the identified criteria.
Biswas et al. [52] proposed an MCDM framework for comparing 20 popular electric vehicles in India based on both technical attributes and user opinions. A q-rung ortho-pair fuzzy set was used to handle imprecise information. Technical performance was evaluated using 13 attributes and entropy-based weighted normalization. User opinions from experts were collected on 13 factors using a linguistic scale and aggregated with Einstein weighting. Both sets of results were combined using the alternative ranking order method accounting for two-step normalization (AROMAN). Dwivedi and Sharma [53] focused on the pressing need for environmentally friendly transportation options, specifically the shift from gasoline or diesel-powered vehicles to fully electric vehicles. The aim of the study was to evaluate and rank fifteen different brands and models of electric vehicles available globally. To analyze and rank the EVs, the study employed two distinct methods. Shannon’s entropy method was applied to determine the weight of each criterion, and TOPSIS was used for ranking EVs. Althaqafi [54] presented a methodology for evaluating and ranking green supply chain management (GSCM) practices using fuzzy TOPSIS. The study aimed to provide electric vehicle manufacturers with a framework for assessing GSCM practices to promote more sustainable supply chains. It identified criteria such as environmental impact, cost efficiency, and social responsibility and developed alternatives representing different GSCM strategies. By incorporating fuzzy logic with TOPSIS, the study offered a method for handling imprecise data, allowing for a more accurate and comprehensive evaluation of GSCM criteria. Barić et al. [55] reviewed previous studies that have evaluated the effectiveness and efficiency of lithium-ion batteries (LIBs) in EVs using cost-benefit analysis (CBA) and MCDM methods. They identified 40 relevant studies published between 1997 and 2023. These studies applied CBA and MCDM methods to evaluate LIBs in various areas, including optimal battery technology selection, energy storage systems, recycling processes, efficiency testing, electric vehicle charging station selection, risk assess- ment, and material supply problems. The review found that MCDM methods considered multiple evaluation criteria, while CBA focused only on cost.
Golui et al. [56] presented a study on selecting the most suitable EVs. They addressed the challenges faced by buyers in choosing an EV due to the technical complexities and lack of knowledge. They employed an MCDM approach and introduced an enhanced TOPSIS method within a Fermatean fuzzy context incorporating a novel correlation coefficient. Key evaluation factors highlighted the range and price of the EVs over factors like battery life, storage, and charging time. Gokasar et al. [57] focused on the implementation of EVs in developing countries where energy production predominantly relies on fossil fuels. The research evaluated the alternatives for introducing EVs to the market. A decision-making process involving a case study was explored using Type-2 neutrosophic numbers (T2NNs). The findings indicated that postponing the adoption of EVs was less favorable. Gönül et al. [58] studied the strategic siting of electric vehicle charging stations (EVCSs). Their study focused on developing a framework for locating EVCSs along highways, considering factors such as service level, traffic density, and proximity to connection roads. Utilizing expert opinions to weigh these criteria, a clustering-based approach was employed to identify optimal locations. Nath et al. [59] examined the development of EV charging infrastructure. The paper presented a combined ranking of key interventions through an MCDM process, identifying the priority of actions needed. The study concluded that a unified ranking of technical and policy interventions could facilitate the rapid and widespread deployment of EV charging infrastructure.
In one of the recent studies, Ramesan et al. [60] carried out research on overcoming the challenges of adopting electric vehicles. In their study, a set of 13 challenges was examined. The titles of these challenges and a brief description of each can be seen in Table 1. These challenges have been considered as a foundation for the analyses in the current study. The decision to utilize a set of challenges validated by previous researchers was made by the experts conducting evaluations in the current study. One of the main reasons for choosing the reference [60] is that its case study and the current research have significant cultural, social, and geographical similarities.
2.2. Methodology
In this research, a statistical analysis approach along with the DEMATEL technique is used to evaluate the challenges of using electric transportation systems. Initially, some existing challenges are extracted from the literature, and based on these challenges, questionnaires are designed. Then, exploratory factor analysis (EFA) is used to categorize the challenges and the DEMATEL approach is utilized to assess their importance. The flowchart of the methodology used in the research is shown in Fig. (1).
Finding the intricate and hidden structure within the data can be done statistically with the help of EFA. By reducing the number of variables and finding correlation patterns between them, EFA aims to preserve original information while reducing the number of variables. This is achieved by converting the observed data into a smaller collection of fundamental variables known as factors. Researchers can uncover underlying structures that might not be immediately obvious and develop a deeper knowledge of the relationships between variables by using this strategy. Early on in a study project, when the precise data structure is unknown, EFA can be very helpful in guiding future investigations and the creation of new measurements and hypotheses.
No. | Challenge | Description | Reference |
---|---|---|---|
1 | High cost of EVs and batteries (C1) | The cost of batteries, particularly those utilizing lithium in their production, can be attributed to the resources and raw materials needed. Electric vehicles primarily rely on lithium-ion batteries comprised of lithium, cobalt, and nickel Aaterials predominantly sourced from a few countries holding an advantageous position in the global lithium supply. This scenario results in supply chain bottlenecks for battery production, further complicated by various political and economic factors worldwide. Additionally, processes related to the extraction of lithium and other raw materials are costly and use a large amount of energy. | Ramesan et al. [60], Goel et al. [61], Shashank et al. [62] |
2 | Insufficient charging infrastructure (C2) | One of the key challenges hindering the widespread adoption and convenience of EVs is the scarcity or inadequacy of available recharging facilities. This shortfall leaves EV owners with limited options, particularly for long journeys, and poses specific difficulties in rural or underdeveloped areas. | Ramesan et al. [60], Patyal et al. [63] |
3 | Lack of Government support to promote EVs (C3) | One significant and pervasive obstacle in the electric vehicle market is the absence of government assistance for the adoption of EVs. Government incentives like financial aid and subsidies have a significant impact on how appealing electric vehicles are to customers. | Ramesan et al. [60], Patyal et al. [63] |
4 | Lack of recycling facilities for EV batteries (C4) | Electric vehicle batteries are made from valuable minerals such as lithium, nickel, cobalt, and manganese. These minerals are necessary for producing new batteries; therefore, recycling electric vehicle batteries is essential to conserve resources and reduce pollution. | Ramesan et al. [60], Capuder et al. [64], Goel et al. [61] |
5 | Unavailability of skilled workers for EV ecosystems (C5) | Compared to vehicles with conventional internal combustion engines, electric vehicles require a different set of skills for design, production, and maintenance. Sophisticated electrical systems, cutting edge battery technology, and new software to control the vehicle's performance are all found in electric vehicles. | Ramesan et al. [60] |
6 | High electricity tariff (C6) | The issue of expensive electricity rates poses a serious obstacle to the general use of electric vehicles. This has an impact on the construction of a larger infrastructure to enable EV charging as well as the operational affordability of EVs for their owners. | Ramesan et al. [60] |
7 | Lack of taxation support for adopting EVs (C7) | The availability or absence of tax support has a direct impact on the financial incentives that companies and consumers contemplating electric vehicles are offered. To lower the effective cost of electric vehicles and increase their competitiveness with conventional internal combustion engine vehicles (ICEVs), governments frequently employ tax breaks, rebates, and credits. | Ramesan et al. [60] |
8 | Lack of public awareness (C8) | One of the biggest challenges to the global switch to electric vehicles is a lack of public awareness. The advantages and applications of owning an electric car are not well known to many prospective customers, despite advancements in technology and a growing focus on sustainability in recent years. | Ramesan et al. [60], Goel et al. [61], Patyal et al. [63] |
9 | Lack of business model to overcome the challenges in the adoption of EVs (C9) | Compared to their internal combustion equivalents, EVs have a greater starting cost due to the ongoing high cost of EV technology, notably batteries. It is becoming harder for businesses to find models that can reduce these costs or provide enough value to justify the premium. Furthermore, in order to guarantee user comfort and reliability, the adoption of EVs requires a significant development of the infrastructure for charging. | Ramesan et al. [60] |
10 | Reluctance towards enhancing a clean and healthy environment (C10) | The adoption of electric vehicles is hampered in large part by a reluctance to promote a clean and healthy environment. This hesitation can be attributed to a number of issues that have an impact on how quickly people, businesses, and governments adopt electric vehicles. | Ramesan et al. [60] |
11 | Poor efforts to reduce the pollution from vehicles/transport (C11) | This complex issue has broad ramifications for both urban and rural settings and stems from the public, business, and governmental domains. Stricter laws intended to reduce vehicular pollution are lacking in many areas, especially laws that support the switch to greener forms of transportation like EVs. | Ramesan et al. [60] |
12 | Lack of public willingness and public resistance to adopting new EV technologies (C12) | This resistance may be caused by a number of issues. People frequently cling to their familiarity. The majority are knowledgeable about internal combustion engine vehicles, including their maintenance, fueling, and general operation. The shift to electric vehicles includes a change that many may find overwhelming or unneeded. | Ramesan et al. [60], Goel et al. [61], Patyal et al. [63] |
13 | Immature technological advancement in EVs and battery technologies (C13) | This obstacle raises the price of electric vehicles and restricts their usefulness, affecting their wider adoption. Energy density, or the quantity of energy that can be stored in a given mass of the battery, is one of the main issues with EV batteries. The energy density of modern lithium-ion batteries is still lower than that of fossil fuels despite tremendous advancements in this area. | Ramesan et al. [60], Shashank et al. [62] |
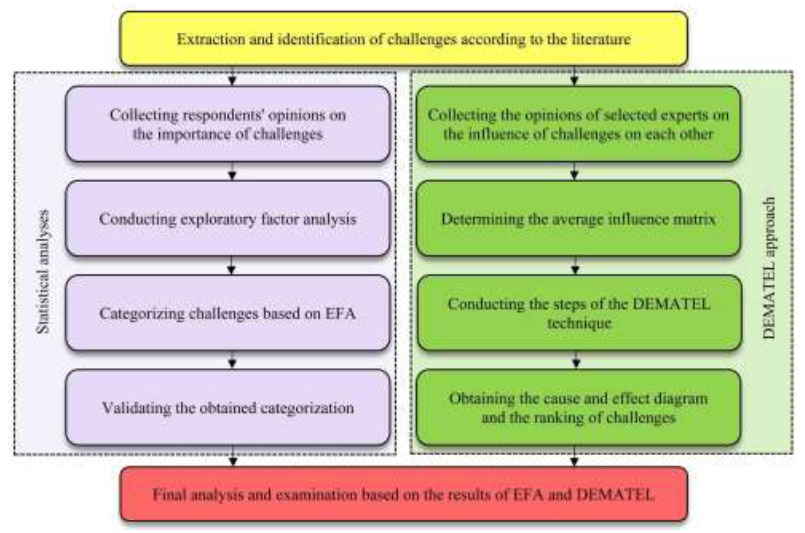
The flowchart of the methodology.
On the other hand, the DEMATEL method is a technique used for making decisions in complex systems, which is meant to help in cause and effect analysis. With the use of this technique, one can more easily recognize and evaluate the intricate connections among the various elements or components that go into a given situation. In order to uncover important aspects that influence or are affected by others, DEMATEL uses a systems approach to create communication maps that show underlying causes and effects. Using a questionnaire, experts assess each factor's influence on the others according to a predefined scale, which is the first step in the DEMATEL implementation process. Subsequently, the acquired data are examined mathematically in order to produce an interaction matrix. The matrix displays the degree of connection and effect among the variables. A network map that illustrates the cause-and-effect links between elements and aids in analysis and decision-making based on a more precise understanding of system dynamics is created using the data gathered from the matrix.
For statistical analysis, the opinions of 285 respon- dents (researchers and academics) in Iran were collected based on the importance of the identified challenges using a Likert scale ranging from 1 to 5. The existing challenges are categorized using the EFA approach with SPSS software (Version 25). After categorizing the challenges, the model obtained will be validated using LISREL software (Version 8.8).
After selecting ten experts out of the 285 respondents who have practical experience in the field of electric transportation systems, a DEMATEL questionnaire is sent to each of these experts. This questionnaire provides pairwise comparisons on the level of influence of each challenge (which is considered a criterion in this technique) compared to other challenges. In this research, the following spectrum is used to assess the level of influence:
0: No influence.
1: Very low influence.
2: Low influence.
3: Medium influence.
4: High influence.
5: Very high influence.
By collecting responses from the ten experts, the average matrix is obtained, and the steps of the DEMATEL technique are used for further analysis.
Suppose that aij denotes the average extent of influence of criterion i on criterion j, or the elements of the average matrix (A). If we have n criteria, the following steps are used in the DEMATEL technique.
Step 1. Calculate the initial direct influence matrix (D). By normalizing the average matrix A, where all elements on the main diagonal are set to zero, we can create the initial direct influence matrix. This matrix D illustrates the initial influence that one element has on another and vice versa. This matrix can be calculated using the following equations:
![]() |
(1) |
and s is calculated as follows.
![]() |
(2) |
Step 2. Determine the full direct/indirect influence matrix (T). This matrix is calculated as follows.
![]() |
(3) |
Step 3. Set the threshold value for the impact digraph-map. A threshold value for the influence level must be determined by the decision maker in order to produce an appropriate impact digraph map. Aspects that have influence levels in matrix T greater than the threshold value are the only ones that can be selected and transformed into an impact-digraph-map. In this paper, the threshold is considered as a specific percentage (α) more than the average of the elements in matrix T. For instance, if α is 20 percent, the threshold will be 1.2 times the average of the elements in T.
Step 4. Calculate the Prominence and Relation values. Ri + Cj is called the “Prominence” and Ri-Cj is called the “Relation”.
![]() |
(4) |
![]() |
(5) |
Step 5. Create the cause and effect diagram or impact-digraph-map based on the threshold value and by mapping the dataset (Ri + Cj:Ri-Cj) obtained from the previous step.
Step 6. Rank the criteria (challenges) according to their importance. The following formula can be used for the ranking.
![]() |
(6) |
There are several benefits to applying DEMATEL and EFA approaches for assessing the challenges associated with utilizing and implementing electric vehicles. This combination offers a thorough and varied method to comprehend these problems more fully. These two methods will enable us to have a precise and thorough understanding of the issues and challenges pertaining to the adoption of electric automobiles. This approach takes into account the significance and influence of all pertinent aspects, enabling us to precisely identify priorities for action and offer practical ways to overcome roadblocks. In summary, these two approaches effectively and precisely pinpoint forthcoming prospects and obstacles, furnishing significant insights to fortify approaches aimed at expediting the adoption and utilization of electric vehicles.
4. RESULTS AND DISCUSSION
In this section, we first present the results of the statistical analysis, followed by the use of the DEMATEL technique to examine the challenges of adopting Electric Vehicles (EVs).
4.1. Statistical Analysis
As mentioned in the previous section, the opinions of 285 individuals in Iran are utilized for statistical analysis. EFA is initiated using SPSS software. To obtain results in this analysis, the PCA approach is utilized, and the rotation method is Varimax. Additionally, the software parameters are set to display only loadings greater than 0.3. To demonstrate that the collected data meet the standard for conducting EFA, KMO (Kaiser-Meyer-Olkin) and Bartlett tests are employed.
Table 2 presents the descriptive statistics related to the collected data. It is worth mentioning that the collected data are provided as Supplementary material [65]. The descriptive statistics allow us to approximately understand the significance of each of the challenges. For instance, based on the Mean value, challenges C3 and C12 have relatively high importance. However, these values alone are insufficient for determining indicators' importance. Table 3 shows the results of the KMO and Bartlett tests. Considering that a KMO value close to 1 and a Sig value smaller than 0.05 are desirable for standard data, the obtained results confirm that the collected data meet the necessary standard for EFA analysis. Table 4 presents the output of FA using the PCA approach. This table indicates that after rotation, we will have three main factors that together account for more than 65% of the total variance of the data.
The loadings of these three factors, according to the challenges, are provided in Table 5. Based on the obtained results, the existing challenges can be distributed among these three factors. In this regard, challenges C3, C5, C8, C10, C11, and C12 fall under the first factor, challenges C1, C6, C7, and C9 under the second factor, and challenges C2, C4, and C13 under the third factor. By examining the definitions of the various challenges, it can be found that the challenges of these obtained factors have common characteristics. The characteristics of the challenges in the first factor are primarily social; therefore, this factor is named the Social (SOC) factor. On the other hand, the challenges of the second factor are mostly economic, and thus, naming this factor the Economic (ECO) factor would be appropriate. Moreover, the challenges of the third factor are technological, which is why this factor is named the Technological (TEC) factor.
Challenge | Mean | Std. Deviation | Analysis N |
---|---|---|---|
C1 | 4.54 | 0.714 | 285 |
C2 | 3.33 | 1.752 | 285 |
C3 | 4.58 | 0.960 | 285 |
C4 | 3.66 | 1.505 | 285 |
C5 | 4.17 | 0.905 | 285 |
C6 | 3.60 | 0.558 | 285 |
C7 | 3.53 | 0.597 | 285 |
C8 | 3.67 | 0.976 | 285 |
C9 | 4.34 | 0.836 | 285 |
C10 | 3.88 | 0.900 | 285 |
C11 | 4.31 | 1.181 | 285 |
C12 | 4.57 | 0.843 | 285 |
C13 | 4.11 | 1.366 | 285 |
Kaiser-Meyer-Olkin Measure of Sampling Adequacy. | 0.826 | |
---|---|---|
Bartlett's Test of Sphericity | Approx. Chi-Square | 1802.310 |
df | 78 | |
Sig. | 0.000 |
Factors | Extraction Sums of Squared Loadings | Rotation Sums of Squared Loadings | ||||
---|---|---|---|---|---|---|
Total | % of Variance | Cumulative % | Total | % of Variance | Cumulative % | |
1 | 4.892 | 37.628 | 37.628 | 3.045 | 23.423 | 23.423 |
2 | 2.305 | 17.734 | 55.362 | 3.029 | 23.299 | 46.722 |
3 | 1.284 | 9.877 | 65.239 | 2.407 | 18.517 | 65.239 |
- | Factors | ||
---|---|---|---|
1 | 2 | 3 | |
C1 | - | 0.752 | - |
C2 | - | - | 0.889 |
C3 | 0.680 | - | - |
C4 | - | - | 0.947 |
C5 | 0.752 | - | - |
C6 | - | 0.833 | - |
C7 | - | 0.831 | - |
C8 | 0.550 | 0.342 | - |
C9 | - | 0.715 | - |
C10 | 0.646 | 0.425 | - |
C11 | 0.761 | - | - |
C12 | 0.690 | 0.376 | - |
C13 | - | - | 0.824 |
To ensure the validity of this classification, the derived model is analyzed using the same data and the LISREL software. The results shown in Fig. (2) indicate that with the Chi-square to degrees of freedom ratio being less than 3 and the RMSEA value less than 0.08, the model is accepted to a satisfactory level. It is noteworthy that the LISREL outcome indicates values of NNFI=0.97, CFI=0.97, GFI=0.94, and AGFI=0.91, all exceeding 0.9, which confirms the validity of the performed classification.
4.2. Using the DEMATEL Approach
In this section, we explore the results derived from the DEMATEL technique based on the opinions collected from ten selected experts. Detailed data can be found in Reference [65]. The average matrix (A), used for conducting the DEMATEL steps, is displayed in Table 6, and the final outcomes of the DEMATEL process are presented in Table 7. In Table 7, the values of Prominence, Relation, and wj, as well as the ranking of each challenge based on wj values, are provided. As mentioned in the previous section, determining a threshold value is necessary for depicting the impact-digraph-map. In this part, three threshold values, α =0%, α =20%, and α =40%, are utilized to draw the impact-digraph-map, shown in Figs. (3 to 5). The interrelationships among challenges can be observed in these figures. It is clear that with higher α values, it becomes easier to identify the most significant challenges. Notably, at α=40%, challenges C3 and C12 still maintain their mutual relationships with other challenges. The importance of these challenges is also reflected in their rankings.
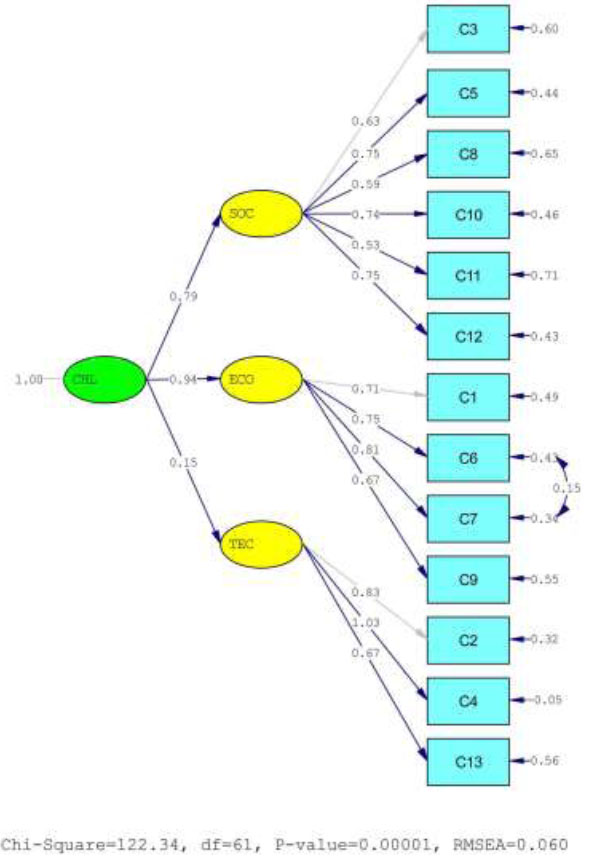
The results of the categorization validation using LISREL.
Challenges | C1 | C2 | C3 | C4 | C5 | C6 | C7 | C8 | C9 | C10 | C11 | C12 | C13 |
---|---|---|---|---|---|---|---|---|---|---|---|---|---|
C1 | 0 | 1.5 | 3.2 | 2.2 | 1.6 | 1.7 | 1.5 | 1.5 | 1.5 | 3 | 3.6 | 4.7 | 4.1 |
C2 | 1.9 | 0 | 1.4 | 1.6 | 1.3 | 1.5 | 1.6 | 1.4 | 1.5 | 2.8 | 1.3 | 2.6 | 1.4 |
C3 | 4.5 | 4.4 | 0 | 3.9 | 3.9 | 4.3 | 4.4 | 2.7 | 1.5 | 3 | 4 | 4.1 | 4.3 |
C4 | 2.6 | 1.4 | 1.5 | 0 | 1.7 | 1.4 | 1.6 | 1.8 | 1.7 | 1.5 | 1.2 | 2.5 | 1.6 |
C5 | 2.5 | 2.8 | 3 | 2.8 | 0 | 1.4 | 1.5 | 1.2 | 4.2 | 1.6 | 3.4 | 1.7 | 4.2 |
C6 | 3.5 | 3.5 | 3 | 1.6 | 1.6 | 0 | 1.5 | 1.6 | 1.5 | 4.4 | 3.7 | 3.7 | 4.1 |
C7 | 3.1 | 2.3 | 2.8 | 3.1 | 1.7 | 3.1 | 0 | 1.4 | 1.5 | 2.9 | 3.1 | 3.6 | 2.6 |
C8 | 1.4 | 1.3 | 3.6 | 1.5 | 4 | 1.4 | 1.4 | 0 | 2.7 | 2.7 | 3.9 | 4.3 | 2.3 |
C9 | 3.1 | 2.9 | 2.4 | 2.7 | 2.1 | 2.4 | 2.9 | 2.8 | 0 | 2.2 | 2.5 | 3.2 | 3.2 |
C10 | 1.8 | 1.5 | 3 | 2.3 | 2.3 | 1.6 | 2.4 | 2.8 | 2.4 | 0 | 2.5 | 2.6 | 2.7 |
C11 | 2.6 | 2.4 | 3.1 | 2.3 | 2.2 | 1.6 | 2.4 | 3.1 | 2.3 | 2.9 | 0 | 3.1 | 3.2 |
C12 | 2.4 | 2.7 | 4 | 2.4 | 3.9 | 1.4 | 3 | 4.3 | 3.3 | 3.8 | 3.7 | 0 | 3.3 |
C13 | 4.6 | 3.9 | 3.2 | 4.6 | 1.3 | 1.5 | 1.6 | 1.5 | 1.8 | 2.7 | 3 | 2.8 | 0 |
Challenges | Prominence | Relation | wj | Rank |
---|---|---|---|---|
C1 | 4.697 | -0.226 | 4.703 | 5 |
C2 | 3.722 | -0.727 | 3.792 | 13 |
C3 | 5.651 | 0.694 | 5.694 | 1 |
C4 | 3.795 | -0.754 | 3.869 | 12 |
C5 | 4.219 | 0.165 | 4.222 | 9 |
C6 | 4.144 | 0.741 | 4.210 | 10 |
C7 | 4.180 | 0.359 | 4.195 | 11 |
C8 | 4.224 | 0.334 | 4.237 | 8 |
C9 | 4.235 | 0.442 | 4.258 | 7 |
C10 | 4.478 | -0.360 | 4.492 | 6 |
C11 | 4.855 | -0.301 | 4.864 | 4 |
C12 | 5.532 | -0.017 | 5.532 | 2 |
C13 | 4.958 | -0.351 | 4.970 | 3 |
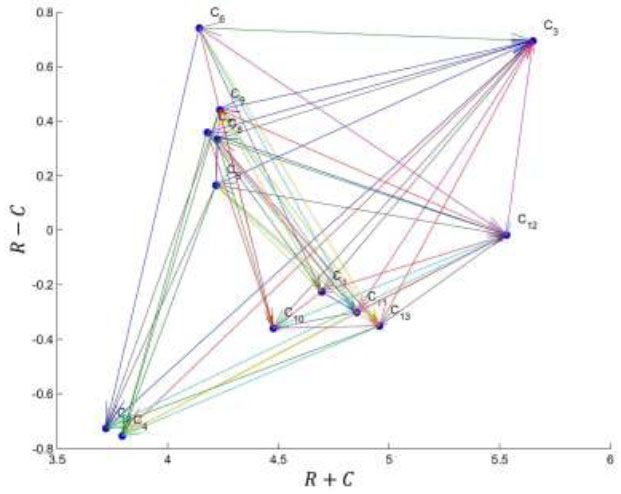
The impact-digraph-maps of the challenges obtained with α =0%.
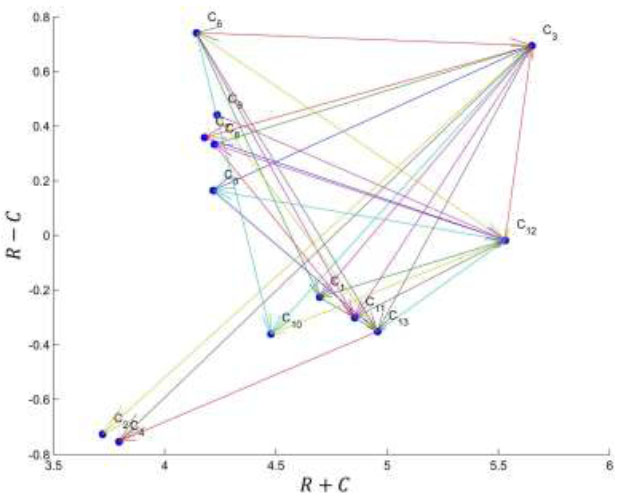
The impact-digraph-maps of the challenges obtained with α =20%.
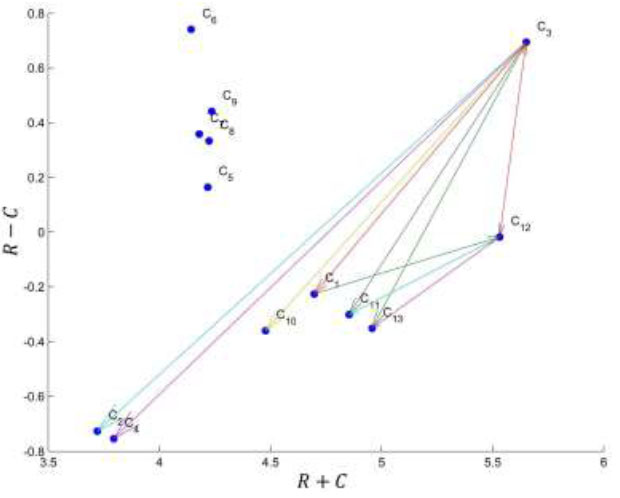
The impact-digraph-maps of the challenges obtained with α =40%.
Two social challenges, C3 and C12, and one techno- logical challenge, C13, are identified as the most significant according to the DEMATEL results. The placement of challenge C3 at the top rank indicates its high importance and the critical role of the government in facilitating the adoption of electric transportation modes. Given the positive Relation value, this challenge acts as a significant influencer on other challenges. On the other hand, challenge C12, with its Relation value below zero, is somewhat influenced by other challenges. However, it remains a significant factor in the adoption of electric vehicles. The same can be said for challenge C13, which, despite being influenced by other challenges, as indicated by a negative Relation value, still occupies a high position in the challenge ranking.
However, it's essential to recognize that economic, social, and technological factors overall play a crucial role in the adoption of electric transportation modes, and the rankings are based on a limited number of expert opinions. Particularly, the statistical model also reveals the overall importance of the economic factor, emphasizing the need to consider the effects of high electricity tariffs and fiscal policies on the adoption of EVs.
CONCLUSION
The importance of evaluating the challenges associated with the adoption of Electric Vehicles (EVs) cannot be overstated. This study underscores the multifaceted nature of these challenges, revealing that they span social, economic, and technological factors, each with its unique characteristics and implications for the widespread adoption of EVs. By categorizing the identified challenges into Social (SOC), Economic (ECO), and Technological (TEC) factors, this research provides a structured framework for stakeholders at all levels to understand and address the barriers to EV adoption comprehensively. The DEMATEL results further illuminate the intricate dynamics among these challenges, particularly highlighting the paramount importance of challenges C3, C12, and C13. The high ranks of social challenges C3 and C12, alongside technological challenge C13, underline the critical need for concerted efforts in these areas. Specifically, the government's role emerges as crucial in mitigating these challenges, as evidenced by the top ranking of challenge C3. The government's proactive engagement in fostering a conducive environ- ment for EV adoption through policy formulation and infrastructure development is essential. Moreover, the significance of the Economic (ECO) factor, as revealed by the statistical model, points to the necessity of addressing economic hurdles such as high electricity tariffs and adverse fiscal policies. These economic constraints can significantly deter potential EV adopters, thereby stalling progress toward sustainable transportation. This study, while based on a limited set of expert opinions, offers valuable insights into the complex interplay of factors affecting EV adoption. It highlights the need for a holistic approach that encompasses social, economic, and technological considerations to promote the widespread use of electric vehicles. Such an approach will not only facilitate the transition towards greener transportation options but also contribute to achieving broader sustainability goals. As the world continues to grapple with the urgent need for environmental sustainability, the findings of this research beckon stakeholders across the board to take informed and decisive steps toward overcoming the challenges of EV adoption, thereby paving the way for a cleaner, more sustainable future in transportation.
The study focused primarily on a specific country (Iran), which may not fully capture the varied challenges experienced globally. Different regions have unique socio-economic and technological environments that can significantly influence the adoption of electric vehicles. To ensure the replicability of this study in diverse contexts, the same exploratory factor analysis and DEMATEL method can be applied to other regions or countries with varying socio-economic and technological conditions. Moreover, the research relies on available data sources, which can introduce bias or inaccuracies. Data on emerging technologies such as electric vehicles can be limited or outdated, affecting the reliability of the findings. Additionally, the categorization and ranking of challenges are dependent on the quality and comprehensiveness of the collected data. The use of exploratory factor analysis and the DEMATEL method, while effective, has its inherent limitations. These methods require subjective judgments in factor extraction and interpreting relationships between challenges, potentially introducing bias. Further, the complexity of multi-criteria decision-making might oversimplify the intricate interplay between diverse factors affecting EV adoption.
Future research could incorporate fuzzy set-based approaches to account for the uncertainty inherent in the decision-making process. Additionally, new decision-making methods such as SECA, EDAS (Evaluation Based on Distance from Average Solution), MEREC (Method based on the Removal Effects of Criteria), and OPARA (Objective Pairwise Adjusted Ratio Analysis) can be utilized for assessing and prioritizing challenges.
AUTHORS’ CONTRIBUTION
The author confirms sole responsibility for the following: study conception and design, data collection, analysis and interpretation of results, and manuscript preparation.
LIST OF ABBREVIATIONS
EVs | = Electric Vehicles |
MCDM | = Multi-criteria Decision-making |
FA | = Factor Analysis |
BSS | = Battery Swapping Stations |
GIS | = Geographic Information System |
AHP | = Analytic Hierarchy Process |
QFD | = Quality Function Deployment |
AVAILABILITY OF DATA AND MATERIALS
The data and supportive information are available within the article.