All published articles of this journal are available on ScienceDirect.
Analysis of Influencing Factor for Rear-end Crash on Toll Road: An Examination using Self-reported Questionnaires
Abstract
Background
Rear-end crashes on toll roads have been a significant issue in Indonesia. This type of crash exists and needs a mitigation strategy. However, limited research exists examining the mechanism and contributing factors of these crashes in Indonesia. This research aimed to describe how rear-end crashes have occurred on toll roads in Indonesia, describe associated risk factors, develop a model for assessing the risk of rear-end crashes on Indonesian toll roads and identify potential mitigation strategies.
Materials and Methods
A questionnaire was developed and assessed for validity and reliability. It was distributed (online) to 220 drivers who had experienced a rear-end crash or near-crash in the past year.
Results
The findings from this study showed that contributing factors are driver (55%), environment (33%), and vehicle (12%). Subject vehicles usually consisted of private vehicles, while the lead (struck) vehicles included private vehicles (91.8%), trucks (7.27%), and buses (0.93%).
Discussion
This study also found two phenomena of local unique behavior in Indonesian toll roads that lead to rear-end crashes, such as “Lane Hogger” and driving under the speed limit (trucks) that lead other vehicles to rear-end crashes.
Conclusion
Factors contributing to rear-crash risk on Indonesian toll roads were driving experience (ownership driving license 15-20 years), breaking driving rules, driving in the right lane, and using an MPV. The findings from this study can improve potential mitigation strategies, including developing focused driving rules, traffic signs on toll roads, and crash avoidance technology.
1. INTRODUCTION
Rear-end crashes are the most frequent crash type on toll roads in several countries and the leading cause of death [1, 2]. A rear-end crash occurs when the subject vehicle strikes, from behind, a lead vehicle (or object) [3, 4]. The type of subject vehicle or leading vehicles can be a small vehicle (sedan, jeep, minibuses, sport-utility vehicles (SUVs), and vans) or a big vehicle (light truck vehicles (LTVs), heavy-duty trucks, and buses) [5, 6]. In the United States, rear-end crashes account for approximately 34% of the 40 million crashes that occur annually. Similarly, in China, 40% of all highway crashes are rear-end crashes [2]. In Indonesia, more than 67% of all crashes that occurred on toll roads were rear-end crashes that occurred in 2020 were rear-end [7].
Previous studies have identified three primary factors that are related to rear-end crash occurrence: driver, vehicle, and road environment [1, 8, 9, 10]. Driver factors include driver behavior, fatigue, and driving experience [6, 11-13]. Vehicle factors refer to the type of vehicle, transmission, speed, and production year [6, 11]. Road environment factors refer to road characteristics, weather, traffic conditions, and the visibility status of the road [11, 13]. Several unique local behaviors have been found in Indonesian drivers on toll roads that can lead to rear-end crashes. These unique local behaviors include speeding, low speed (relative to the posted speed limit), driving on the shoulder, overtaking without using turn signals, tailgating, driving in the fast lane constantly (called a “Lane Hogger”) and overtaking vehicles on the left side [7, 14, 15].
There are four rear-end crash configurations on Indonesia toll road; the first configuration was the small vehicle as the subject vehicle had condition following truck, which had low speed. The second configuration was the subject vehicle, and the leading vehicle was a small vehicle that drove on the heavy traffic toll roads during rush hours. The third configuration was the subject vehicle, and the leading vehicle was a small vehicle that drove at high speed on the toll roads [16]. The fourth configuration was the subject vehicle, and the lead vehicle was a small vehicle that failed to control its speed while facing the work zone on the toll road. Indonesian police reports suggest that speeding on toll roads and short headway (i.e., following distances) are primary factors in rear-end crashes [7].
The impact of rear-end crashes in Indonesia as economic losses up to Rp. 229.137.00 in 2018. Approximately 90% of rear-end crashes involving small vehicles affect the injury severity with higher fatality rate (disability, cause of death) and damaged toll facilities (beam guardrail, guardrail pole) [7, 14].
Previous research has studied rear-end crash risks in different countries but has never been conducted in developing countries such as Indonesia, with its high vehicle market and rapid toll road development in the past ten years [17-19]. Previous studies in the United States, Taiwan, and China have included surveys, modeling, laboratory experiments, and naturalistic driving studies. Often, the purpose of these international studies is to determine specific risk factors that may be unique to a specific country so that mitigation strategies can be deve- loped to fit into the country's condition [8, 20]. Examples include studies in the United States that evaluated the safety benefits of forward collision warning (FCW) systems in minimizing rear-end crash occurrence [21, 22]. In Taiwan, research has focused on developing warning systems to support safe following distances [23, 24]. In China, research has investigated various countermeasures that may reduce rear-end crashes in that country [2, 8, 25-27].
In addition, two predominant methodologies are often employed for developing rear-end crash risk models: self-reporting and utilization of accident data [28]. Both approaches aim to unravel the complexities of rear-end crashes. Self-reported data offer valuable insight into the human factors underlying rear-end crashes, with flexibility, adaptability, and cost-effective alternatives [29-31]. On the other hand, the differences in road geometry, traffic conditions, driver behavior, and incredibly unique local behavior would cause different risk factors, strongly influencing rear-end crashes.
The primary goal of this study is to investigate the impact of risk factors such as driver, vehicle, and road environment that contribute to rear-end crashes on Indonesian toll roads, based on the driver’s self-reporting. The study aims to elucidate the underlying rear-end crash mechanisms and contribute to the existing literature on rear-end crash risk on toll roads using Multiple Binary Logistic Regression (BLR). Our findings are expected to shed light on unique local driver behavior and offer insight into practical strategies for decreasing the rear-end crash risk on Indonesia toll roads. By identifying key patterns and correlations between several risk factors, this research aims to inform policymakers, practitioners, and stakeholders in toll roads and contribute to developing effective strategies or interventions that policymakers in Indonesia can utilize.
As such, the present study posited the following hypothesis based on previous research, observation of toll road conditions, and results of Indonesian police interviews:
1.1. Hypothesis 1: Driver Behaviors Factors
Unique local drivers’ behaviors in Indonesia will contribute to the rear-end crash risk on toll roads.
1.2. Hypothesis 2: Vehicle Factors
Types of vehicles in Indonesia will contribute to the rear-end crash risk on toll roads.
1.3. Hypothesis 3: Road Environment Factors
Road environment factors in Indonesia will contribute to the rear-end crash risk on toll roads.
Our study aims to investigate and understand more about the rear-end crash risk on toll roads, especially in Indonesia, focusing on driving behavior. In these research gaps, we developed models and comprehensively analyzed risk factors influenced by subjective questionnaire analysis based on several types of driving behavior questionnaires. After that, we employed the binary logistic regression model to estimate the probability of the rear-end crash risk.
2. MATERIALS AND METHODS
2.1. Participants and Data Collection
A purposive sampling method was used to perform an e-survey [32]. The requirements for participating in the e-survey were that the participants be male or female, 20-64 years old, have had a near-crash or rear-end crash on two major toll roads in Java Island, Indonesia, and have had a driving license type “A” for three years minimum [1].
The data were collected from a survey sample of n = 220 drivers, of which 30 (13.64%) participants had a rear-end crash experience and 190 (86.36%) participants had a near-crash experience. Of the participants, 131 (60%) were males, and 89 (40%) were females. The sample participants were between 20 and 64, with a mean M = 30.14 (SD = 6.46) years. More than half of the participants (55.25%) had an undergraduate degree, 27.9% had a postgraduate degree, 6.4% had a diploma, 10.5% had a high school, and 0.5% had junior high school education.
2.2. Description of the Questionnaire and Study Variables
The questionnaires were processed using a back translation process, validation, and reliability test with thirty participants. The final questionnaire, consisting of three sections for 220 participants, was distributed in Indonesian. The first section (12 questions) asked about demographics, gender, age, education, occupation, city region, cigarette consumption, caffeine consumption, driving experience, average driving miles, annual mileage, the way to get a driving license, and type of driving license. The second section (33 questions) asked several questions about driver behavior from the Driving Behavior Questionnaire (DBQ), Freight Driving Behavior Quest- ionnaire (F-DBQ), and Driving Attitude Questionnaire (DAQ) [9, 27, 33]. Drivers’ behavior questions consisted of risky driving behavior and fatigue with a frequency Likert-based questionnaire of 6 levels (1 = never - 6 = always). The third section (18 questions) covered rear-end crash conditions, including vehicle speed, type of vehicle, lane, road geometric, road traffic, weather, time, and visibility. The operational definition of the variable in this study is shown in Table 1. The variable consisted of rear-end crash risk as the dependent variable and twelve independent variables such as demographic, driving experience, risky driving behavior, fatigue, vehicle speed, type of vehicle, type of lane, road geometric, road traffic, weather, time, and visibility.
2.3. Ethics
During the design research, the methodology was conducted by referencing the Declaration of Helsinki in October 2000. The self-reported questionnaire contains informed consent for all participants. Informed consent included explaining the study aim, the number of questions, the average survey duration, the treatment of personal data, and the voluntary participants. All the information was always provided before participants answered the questionnaire. The personal or confidential data of participants were not used and formed anonymously [46].
Variable | Operationalization | Total Items of Question | References |
---|---|---|---|
Rear-end crash risk |
The condition while the driver faces a critical situation, such as a near crash or crash on a toll road, is measured by the distance between the subject vehicle and the leading vehicle. | 1 | [3, 8, 34] |
Demographic | Driver characteristics such as age, gender, smoking activity, consuming caffeine, educational level, and region of the city. | 7 | [1, 8, 35-37] |
Driving experience |
Driving experience includes driving license duration, average miles in the last three years, annual mileage, and the method of obtaining a driving license. | 6 | [1, 37-39] |
Risky driving behavior | Driver behavior includes using gadgets while driving, looking at the other side, interacting with passengers and overtaking other vehicles using the left side of the toll road. | 22 | [33, 40-42] |
Fatigue | Driver biological warning conditions for rest, such as drowsiness, sleepiness, and physical fatigue, can decrease the driver’s response. The other condition can cause response failure from the driver while driving on the toll road. | 4 | [43] |
Vehicle Speed | Vehicle speed on toll roads included acceleration and deceleration. | 5 | [1, 4, 8] |
Type of vehicle | Personal vehicles (Sedan, SUV, MVP, Minibus, bus, truck); Production year; Distance between vehicle (meter); Type of vehicle ownership (personal vehicle, public transportation); Vehicle brand; Vehicle transmission (Manual, Automatic). | 5 | [4, 36, 44] |
Type of lane | Types of lanes in toll roads include lanes 1,2,3,4, roadside, and interchange areas. | 2 | [3, 8, 44] |
Road Geometric |
Road geometrics on toll roads are straight flat, straight down, straight up, flat bend, bend down, and bend up. | 4 | [44, 45] |
Road Traffic | Road traffic on toll roads (very congested traffic, low vehicular flow, and regular travel velocity). | 2 | [9] |
Weather | Weather on the toll road (sunny, cloudy, rainy). | 1 | [1, 8, 44, 45] |
Time | Driving time (morning, afternoon, evening, weekdays, weekend). | 2 | [3] |
Visibility | Visibility level while driving on the toll road. | 2 | [1, 45] |
2.4. Data Analysis
Microsoft Excel transformed the collected responses into coding numbers before processing them with statistical analysis programs. The data obtained from the self-reported questionnaire in sections one and three were processed using statistical descriptions. The processed data is grouped based on the distribution of demographic data and the driver's condition when s/he had a near-crash or rear-end crash on the toll road. The second section of questionnaire data was processed by multiple binary logistic regression for statistical analysis using IBM SPSS Statistics V25 software(IBM, 2022) [47].
3. RESULTS
A total of 220 drivers completed the questionnaire, consisting of 30 drivers with a rear-end crash and 190 drivers with a near-crash. The sample of drivers noted risk factors, including driver, vehicle, and road environment. Based on the questionnaire results, the data processing used descriptive analysis and provided the model development of the rear-end crash risk factors model on toll roads.
3.1. Descriptive Analysis
The risk factors reported during the rear-end crash or near crash on a toll road are shown in Table 2. A driver’s factor includes driving experience. Vehicle factors consist of maximum vehicle speed, average vehicle speed on toll roads, the type of vehicle, the type of ownership of vehicles, the production year, and the type of vehicle transmission variable. The road environment factors consist of lane type, road geometric, road surface condition, road traffic, weather, time, days, visibility, and type of traffic sign.
The results show that 44.5% of the sample had 1-5 years of driving experience, of which 86% had near- and 14% had rear-end crashes. The maximum driver’s speed on toll roads was 80-100 km/hour (41.4%) of the total sample. The average driver’s vehicle speed was 80-100 km/hour on the toll road (60%) of the total sample. The type of vehicle (42%) was an SUV that had rear-end crashes on toll roads. Of the type of ownership vehicle, 99% of the sample is private vehicles. The production year of the vehicle shows 45% of the sample used vehicles with production year between 2015-2022. Drivers had incidents of Rear-end crashes in the right lane (90%), flat straight road geometric (77%), and the road surface condition was dry (82%). Besides that, the drivers who had rear-ended crashes in conditions of road traffic tend to flow smoothly (52.7%), bright weather (51.8%) with good visibility (48%) at 12.00-23.59 (37.3%), weekdays (52%) and the road fulfill with type of traffic sign 80 km/hour (39.1%).
Factors | Variable | Item | Near Crash (%) | Rear-end Crashes (%) | Total Sample (%) |
---|---|---|---|---|---|
Driver | Driving Experience (years) | 1-5 | 84 (86) | 14 (14) | 98 (44.5) |
5-10 | 73 (89) | 9 (11) | 82 (37.4) | ||
10-15 | 23 (82) | 5 (18) | 28 (12.7) | ||
15-20 | 4 (67) | 2 (33) | 6 (2.7) | ||
20-25 | 2 (100) | 0 (0) | 2 (0.9) | ||
>25 | 4 (100) | 0 (0) | 4 (1.8) | ||
Vehicle | Maximum vehicle speed on Toll Road (km/hour) | 40-60 | 0 (0) | 0 (0) | 0 (0) |
60-80 | 52 (88) | 7 (12) | 59 (26.8) | ||
80-100 | 78 (86) | 13 (14) | 91 (41.4) | ||
100-120 | 52 (85) | 9 (15) | 61 (27.7) | ||
>120 | 8 (89) | 1 (11) | 9 (4.1) | ||
Average vehicle speed on Toll Road (km/hour) | 40-60 | 3 (75) | 1 (25) | 4 (1.8) | |
60-80 | 60 (86) | 10 (14) | 70 (31.8) | ||
80-100 | 116 (88) | 16 (12) | 132 (60) | ||
100-120 | 11 (79) | 3 (21) | 14 (6.4) | ||
>120 | 0 (0) | 0 (0) | 0 (0) | ||
Type of vehicle | Sedan | 83 (91) | 8 (9) | 91 (41.4) | |
MPV | 66 (81) | 15 (19) | 81 (36.7) | ||
SUV | 35 (83) | 7 (17) | 42 (19.1) | ||
Minibus | 5 (100) | 0 (0) | 5 (2.3) | ||
Vehicle | Type of ownership vehicle | Truck | 1 (100) | 0 (0) | 1 (0.5) |
Private vehicle |
187 (86) | 30 (14) | 217 (99) | ||
Public vehicle |
3 (100) | 0 (0) | 3 (1) | ||
Vehicle | |||||
Production of vehicle | 1990-1995 | 3 (75) | 1 (25) | 4 (1.8) | |
1995-2000 | 0 (0) | 1 (100) | 1 (0.5) | ||
2000-2005 | 4 (57) | 3 (43) | 7 (3.2) | ||
2005-2010 | 15 (83) | 3 (17) | 18 (8.2) | ||
2010-2015 | 64 (91) | 6 (9) | 70 (31.8) | ||
2015-2020 | 88 (89) | 11 (11) | 99 (45) | ||
2020-2023 | 16 (76) | 5 (24) | 21 (9.5) | ||
Type of vehicle transmission |
Automatic | 108 (86) | 17 (14) | 125 (57) | |
Manual | 82 (86) | 13 (14) | 95 (43) | ||
Road Environment | Type of lane | Right Lane | 120 (90) | 14 (10) | 134 (61) |
Middle Lane | 36 (80) | 9 (20) | 45 (20) | ||
Left Lane | 25 (81) | 6 (19) | 31 (14) | ||
Road Geometric | Flat straight | 64 (77) | 19 (23) | 83 (37.7) | |
Bend down | 48 (94) | 3 (6) | 51 (23.2) | ||
Straight down | 44 (96) | 2 (4) | 46 (20.9) | ||
Bend flat | 17 (89) | 2 (11) | 19 (8.6) | ||
Bend up | 12 (92) | 1 (8) | 13 (5.9) | ||
Straight up | 5 (63) | 3 (38) | 8 (3.7) | ||
Road Environment | Road surface condition | Dry | 96 (82) | 21 (18) | 117 (53.2) |
Wet | 68 (93) | 5 (7) | 73 (33.1) | ||
Sandy | 12 (86) | 2 (14) | 14 (6.4) | ||
Water pooling |
11 (100) | 0 (0) | 11 (5) | ||
Melted asphalt |
2 (100) | 0 (0) | 2 (0.8) | ||
Fuel spill | 0 (0) | 1 (100) | 1 (0.5) | ||
Oily | 1 (100) | 0 (0) | 1 (0.5) | ||
Uneven surface |
0 (0) | 1 (100) | 1 (0.5) | ||
Road traffic | Tends to flow smoothly | 105 (91) | 11 (9) | 116 (52.7) | |
Congested | 51 (78) | 14 (22) | 65 (29.5) | ||
Very congested |
22 (92) | 2 (8) | 24 (11) | ||
Road traffic | Flowing freely | 12 (80) | 3 (20) | 15 (6.8) | |
Weather | Bright | 92 (81) | 22 (19) | 114 (51.8) | |
Rain | 58 (92) | 5 (8) | 63 (28.6) | ||
Foggy (Visibility <100m) |
40 (93) | 3 (7) | 43 (19.6) | ||
Time | 18.00-23.59 | 74 (90) | 8 (10) | 82 (37.3) | |
12.00-17.59 | 70 (85) | 12 (15) | 82 (37.3) | ||
Time | 06.00-11.59 | 26 (79) | 7 (21) | 33 (15) | |
00.00-05.59 | 20 (87) | 3 (13) | 23 (10.4) | ||
- | Days | Weekdays | 98 (85) | 17 (15) | 115 (52) |
Weekend | 92 (88) | 13 (12) | 105 (48) | ||
Visibility | Good (100-200 m) |
89 (85) | 16 (15) | 105 (48) | |
Poor (50-100 m) |
68 (94) | 4 (6) | 72 (33) | ||
Incredibly good (more than 200 m) | 21 (68) | 10 (32) | 31 (14) | ||
Extremely poor (less than 50m) | 12 (100) | 0 (0) | 12 (5) | ||
Type of traffic sign | 80 km/hour | 74 (86) | 12 (14) | 86 (39.1) | |
None | 72 (87) | 11 (13) | 83 (37.7) | ||
60 km/hour | 23 (88) | 3 (12) | 26 (11.8) | ||
Road Environment | 100 km/hour | 21 (84) | 4 (16) | 25 (11.4) |
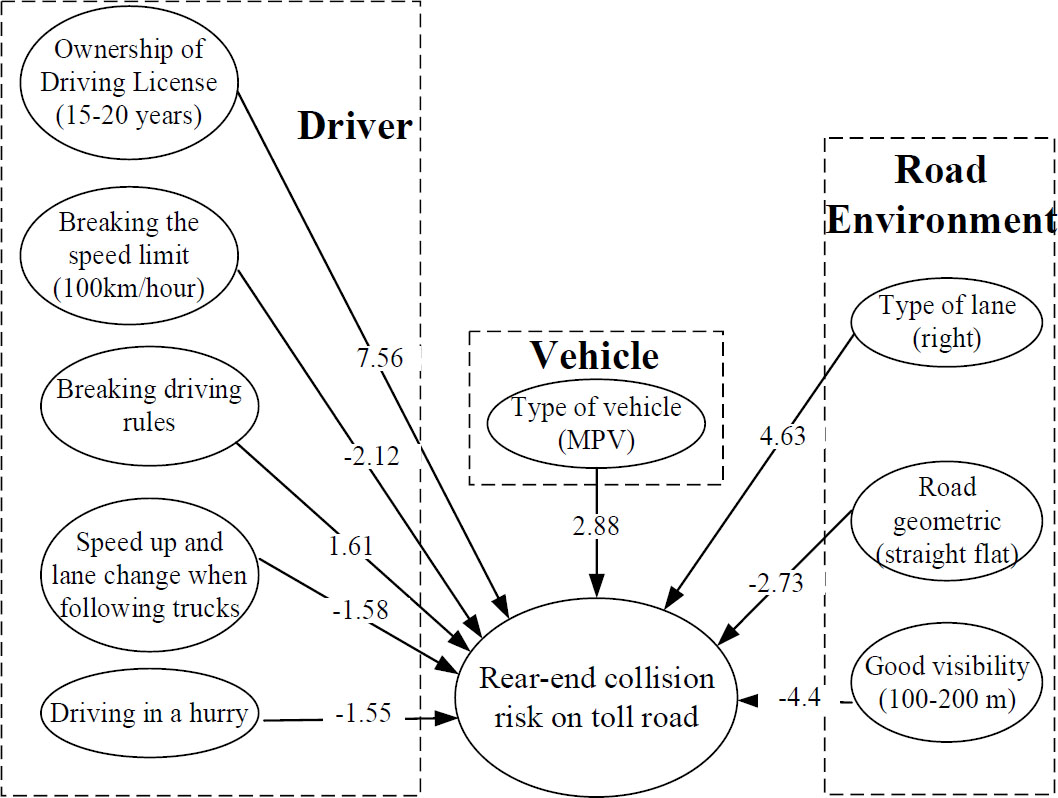
Rear-end crashes risk model only the significant variables.
3.2. Development of Rear-end Crash Risk Factors Model
Based on multiple binary logistic regression, Fig. (1) shows the rear-end crash risk model results with three main factors that can predict the rear-end crash risk on toll roads: driver, vehicle, and road environment. The driver factors had several variables that contributed to rear-end crashes. These factors included ownership of a driving license (15-20 years), breaking the speed limit (100km/hour), breaking driving rules, speeding up and lane change when following trucks, and driving in a hurry. For vehicle factors, only type vehicles (MPV) contributed as a potential variable to rear-end crash risk on toll roads. Environmental factors consisted of three variables: lane type (right), straight flat road geometric, and good visibility (100-200 m).
The rear-end crash risk model can estimate the probability of the rear-end crash using multiple binary logistic regression [1]. Before the regression, the Hosmer and Lem show Test, Nagelkerke R Square test, F test, and T-test were conducted. The P-value result of the Hosmer and Lemeshow Test was 0.651, implying that the model was fit. For the coefficient of determination used, the Nagelkerke R Square value was 0.667, interpreted as 667% of the variation in the rear-end crash risk on the toll road (dependent variable) predicted by the model [48]. The results of the F test using the Omnibus Test were p-value < 0.05, interpreted as non-collinearity between independent variables, and at least one of the independent variables was significant. The estimation results of the multiple binary logistic regression model are shown in Table 3. Nine variables among all varied variables were found to be significantly related to rear-end crash risk probability with a p-value < 0.05.
The results of the multiple binary logistic models in Table 3, the Odds Ratios (OR) or Exp(B), show the impact of the independent variable as a contributing factor on the probability of a rear-end crash on the toll road. The value of OR shows that the variable has an impact, increasing the chance of rear-end crashes. From the results, the predictors with the most significant estimates in magnitude corresponded to the driving experience with the ownership driving license (15-20) years, type of lane (right), type of vehicle (MPV), and breaking the driving rules.
Variable | Code | β | P-value |
(OR) Exp (B) |
95% C. I | ||
---|---|---|---|---|---|---|---|
Lower | Upper | ||||||
Driving experience | Ownership driving license (15-20) years | P11(4) | 7.56 | 0.018 | 1921.60 | 3.672 | 6.118 |
Risky driving behavior | Breaking the speed limit (100 km/hour) | P20 | -2.12 | 0.018 | 0.12 | 0.021 | 0.690 |
Breaking driving rules | P23 | 1.61 | 0.025 | 5.010 | 1.229 | 20.427 | |
Speed up and lane change when following trucks | P33 | -1.58 | 0.011 | 0.206 | 0.061 | 0.694 | |
Driving in a hurry | P46 | -1.55 | 0.014 | 0.212 | 0.061 | 0.732 | |
Vehicle type | Type of vehicle (MPV) | K7(2) | 2.88 | 0.04 | 17.82 | 1.143 | 278.02 |
Type of lane | Type of lane (right) | J1(1) | 4.63 | 0.002 | 102.9 | 5.799 | 1828.6 |
Road geometric | Road geometric (straight, flat) | J3(1) | -2.73 | 0.045 | 0.065 | 0.004 | 0.936 |
Visibility | Good visibility (100-200 m) | L4(1) | -4.4 | 0.024 | 0.012 | 0.00 | 0.561 |
4. DISCUSSION
The primary focus of this study was to determine the contributions of rear-end crash risk factors on Indonesian toll roads. Our study showed three key issues that significantly contribute to rear-end crash risk: the toll road environment, unique drivers’ behavior, especially “Lane-Hogger,” and trucks that exist on Indonesian toll roads.
4.1. Toll Roads
Indonesia has unique characteristics of toll roads compared to other countries, especially in terms of geometry, lane, and traffic conditions. Most tolls in Indonesia are paid, requiring drivers to pay a fee to use these roads. This condition contrasted with other countries, such as the United States, where the toll roads varied, but many highways provided free access for drivers. In other conditions, this different situation produces different drivers’ behavior.
Based on model results, the driver factor consisted of drivers with ownership of a driver's license for 15-20 years associated with rear-ending crash risk on Indonesia toll roads, especially the unique local behavior. Surprisingly, 30% of the drivers who had more experience driving on Indonesia toll roads were more likely to engage in a secondary task while driving, for example, calling. This result was in line with a previous study in the United States that adolescent drivers who did secondary tasks increased crash risk [49]. In another study in Spain, the driving experience factors could affect the driver’s perception of crash risk in risky driving behavior [50].
The risk factor that has negatively contributed to rear-end crashes is risky driving behavior, such as breaking the speed limit. The condition in Indonesian toll road shows that drivers who drive under the speed limit (<100 km/hour) would increase the probability of getting rear-end crashes. This phenomenon exists because 60% of the average maximum vehicle speed is 80-100 km/hour, and rear-end crashes mostly happen in road traffic and tend to be smooth. Those results were opposite results of a previous study, in which the drivers who drive at an excessive speed will have less time to react to surprise maneuvers of other vehicles (sudden brake or lane change) and get a crash [8, 51].
The vehicle type variable shows that the Multi-purpose Vehicle (MPV) has a positive impact on rear-end crash risk in Indonesia Toll Road. This condition occurs because the MPV market in Indonesia is quite high; up to 37% of respondents used MPV. This type of vehicle fulfills most of the Indonesian citizen's needs, such as a large capacity vehicle (family cars), economist prices, and reliability in various road areas. Nevertheless, the previous study shows that MPVs have safety concerns about fundamental structural incompatibility and suspension, whereas full-capacity vehicles are used [52].
In other conditions in Indonesia, there were no rules about the length of used vehicles, like 5 years maximum, so the vehicles that were manufactured before two thousand still existed on toll roads. However, a lack of safety technology could cause rear-end crashes [53]. Safety technology in newer and higher-end vehicles can help drivers avoid crashes when driving on toll roads. However, the mismatch between using old vehicle features and the toll road conditions can also increase the risk of crashes [54, 55].
Regarding the geometric variable of the road, drivers who drive on flat, straight roads have a negative contribution to rear-end crash risk compared to other road geometrics. This result was the opposite of the previous study, which found that road geometry with flat, straight roads can improve driving performance, and drivers can quickly drive at high speeds. This phenomenon occurs because of various geometric on Indonesian toll roads. The various geometric while travelling over long distances may lead to reduced levels of alertness and drowsiness [56]. The condition of a drowsy driver encounters another vehicle with a much lower speed; the driver can have difficulty responding to the situation and risk a rear-end crash [57].
However, drivers who drove when visibility conditions were good (100-200m) on Indonesian toll roads have a negative contribution to rear-end crash risk. This is because when the visibility conditions are bad, drivers find it difficult to estimate the distance between vehicles. They cannot see the road conditions in front of them. This phenomenon was in line with research in Malaysia that the rear-end crashes lead to bad visibility, especially for poor conspicuity and visibility of lightning of the vehicles [58]. Other conditions, such as fog conditions while driving, also increase the bad visibility through rear-end crash risk on toll roads [59].
4.2. Lane Hogger
Driving in the right lane was associated with a higher risk of rear-end crashes (note that Indonesia is a left-hand drive country). At times, slow-moving vehicles occupy the right lane, which should be reserved for passing/higher-speed vehicles. Based on toll road driving rules in Indonesia, the right lane is only used for vehicles that want to overtake other slower vehicles. There are conditions where the driver feels at home to keep driving in the fast lane (called a “Lane Hogger”). This condition often occurs on Indonesian toll roads for right-hand lane users. When the driver continues to drive in the right lane and encounters a slow car in front of him, the driver becomes frustrated, emotional, and disturbed, which can lead to poor driving decisions [60].
The following factor that contributed to a positive impact on rear-end crash risk was breaking the driving rules. More driver violations on toll roads would increase the probability of rear-end crashes. For example, the rules of Indonesian lane road function, the right lane itself must be used for a fast vehicle that wants to speed up another vehicle. The policy on toll roads was created from several technical considerations by the Police, the Department of Transportation, and the Indonesian Government to make their citizen drive safely, but the enforcement is still poor. The data shows that 50% of participants, most of the time, did driver's violations and speed violations. Moreover, effective and proper enforcement is crucial in preventing rear-end crashes, such as violation of traffic signs, vehicle lights used, and turn signals, especially to ensure a safe distance between the vehicles [61].
4.3. Trucks
The Indonesian condition shows that some trucks exist several times on toll roads, and most of the time drive under the speed limit. That condition affects the other vehicles that fail to speed up, and lane changes when following trucks will increase the rear-end crash risk. Meanwhile, the condition in Indonesia is in line with previous studies in China that show drivers who drive following another vehicle that drives less than the speed limit, for example, trucks or other types of vehicles, will increase the rear-end crash risk [9]. This condition occurred because of the speed difference, headway time distance and physiological conditions of drivers [62]. Other condition shows that driving with rushing behavior on Indonesian toll roads had negative effects on rear-end crashes. This scenario arises because of the monotone road; constant vehicle speed will increase the risk of crashes through fatigue levels if it occurs on a long trip [63].
There are some limitations of this study, such as limitations in using self-reporting approaches and the amount of sample of this study. Participants may provide incomplete or inaccurate information about their experience of rear-end crashes due to memory lapses, bias, or reluctance to disclose specific details. Additionally, the sample size of this study and demographic composition may not be representative of all drivers who experience rear-end crashes on Indonesian toll roads.
The implications of this research are manifold and carry significant contributing risk factors in enhancing road safety measures and rear-end crash prevention strategies. Firstly, a comprehensive understanding of the contributing factors to rear-end crashes serves as a foundation for the development of targeted intervention programs, and policy initiatives aimed at mitigating the prevalence of crashes. Insight gleaned from this study can inform the implementation of infrastructure improve- ments, such as warning systems, traffic management protocols, and lane design modifications. Moreover, the identification of key demographic, behavioral, and road environment predictors of rear-end crash risk underscores the importance of targeted increase drivers’ awareness campaigns directed towards vulnerable drivers with high-risk, unique local behaviors. This study shows influencing practical measures aimed at minimizing economic losses and preserving human lives within the realm of toll road safety.
CONCLUSION
Based on the self-reported questionnaire to 220 respondents who experienced near-crashes and rear-end crashes on Indonesian toll roads, they found that the contributing risk factors to rear-end crashes were driver, vehicles, and road environment factors. Then, based on multiple binary regression models, the influencing risk factors and the significant independent variable were established. The driver’s factors included driving experience and risky driving behavior. Vehicle variables included the type of vehicle that the driver drove. Environmental variables included lane type, road geometry, and visibility. Some conditions that make drivers at higher risk of rear-end crashes include the duration of license ownership (15-20 years), violating the speed limit (100 km/h), violating driving rules, using an MPV, driving in the right lane, and failed changing lanes when there is a truck ahead. Other conditions, speeding, driving on a straight, flat road, and driving with good visibility (100-200 m) also were significant factors with negative association.
Moreover, three unique local behaviors on Indonesia toll roads contributed to rear-end crash mechanisms: “Lane Hogger,” driving at speed, especially trucks on the toll roads, and doing the second tasks while driving. The subjective data is exciting and provides insight into an objective study that would follow. Five variable findings, such as risky behavior issues, driving at speed, driving in a bad road environment, and doing a second task while driving, were suggested for field study measured with the objective study. However, further research is needed to determine the condition of driver behavior when driving on Indonesian toll roads by grouping safety-critical event conditions in more genuine and accurate measures for driving situations that can cause rear-end crashes, hazardous driving behavior and road environment.
AUTHORS' CONTRIBUTION
F.S.D.: Writing the Paper; A.W., H.I.: Study Concept or Design; M.A.P., R.Z., V.T.: Data Collection; R.J.H.: Writing - Reviewing and Editing.
LIST OF ABBREVIATIONS
SUVs | = Sport-utility Vehicles |
LTVs | = Light Truck Vehicles |
FCW | = Forward Collision Warning |
ETHICS APPROVAL AND CONSENT TO PARTICIPATE
The study was approved by the The Research Ethics Committee of Bandung Institute of Technology, Indonesia KEP/II/2024/X/M170924FSD-TBDJ.
HUMAN AND ANIMAL RIGHTS
All human research procedures followed were in accordance with the ethical standards of the committee responsible for human experimentation (institutional and national), and with the Helsinki Declaration of 1975, as revised in 2013.
CONSENT FOR PUBLICATION
The self-reported questionnaire contains informed consent for all participants.
AVAILABILITY OF DATA AND MATERIALS
The data supporting the findings of the article is available in the Zenodo at https://zenodo.org/records- /14177537, reference number 14177537.