All published articles of this journal are available on ScienceDirect.
A Review of Traffic Accidents and Related Practices Worldwide
Abstract
A traffic accident, a traffic collision or crash occurs when a vehicle collides with another vehicle, pedestrian, animal, road barrier, or any stationary obstruction such as a tree or a utility pole. Traffic collisions may result in injury, death, vehicle damage and possession damage. Motor vehicle collisions cause death and disability as well as financial burden. Traffic accidents cause many losses especially of human life, property damages, and loss of resources. Indeed, even in strife influenced countries such as Afghanistan, Libya, Pakistan, and Yemen, road traffic accidents remain the most common cause of fatal injuries, causing between two and eight times more fatalities than war and lawful mediation. The World Health Organization (WHO) 2013 assessed the traffic casualty rate in the Eastern Mediterranean Region (EMR) to be the second most elevated rate universally after the African Region and extending a few other countries in the region. The aim of this paper was to enrich the global highway safety knowledge by revealing the catastrophic impact of traffic accidents on the economy of the societies and the safety of the common worldwide.
1. INTRODUCTION
On September 11, 2001, the twin towers of the World Trade Center were devastated and history recorded that over 3,000 people were killed. Not many people know that approximately the same number of people die every day on roads worldwide. This fact does not involve at least the 30,000 others injured or disabled. These compile to over 1 million people getting killed and between 20-50 million getting injured or becoming infirm in road accidents each year. It is explicit that road traffic injuries are a major public health issue globally [1]. In Iraq, for example, more years of the possibility of life are lost due to road casualties than other causes. Year by year, Iraqis die on the roads with a medium of around 7000 persons per year or about 2 persons per hour. In other words, every day, approximately 25 Iraqis who leave home for work, school, university, shopping, or social gathering never return home due to being victims of road accidents [2, 3]. According to Bassam 2017 [4], the number of Iraqis who were killed throughout all the wars that Iraq has been through is still far less than the number of people who considerably die of road
accidents. Thus, if the problem of traffic accidents in Iraq has not been properly addressed, there will be catastrophic consequences on the safety of the Iraqi society. A combination of traffic accidents prediction and control strategies with the expert system technology provides a useful environment for engineers, students, and decision-makers to apply traffic accidents prediction and control strategies.
2. TRAFFIC ACCIDENTS AND RELATED PRACTICES WORLDWIDE
Road traffic accidents are the main cause of death for young people and the eighth major cause of all deaths globally with predestined 1.24 million deaths every year [5, 6]. About 85% of deaths are occurring in developing countries. Males, especially those between 15 and 44 years old, are the extremely affected group of people with traffic accidents. Traffic accidents expenditure of countries is 1 to 2% of their total national products [5]. Although only 52% of vehicles in the world are recorded in developing countries, 80% of road traffic deaths occur in these countries (WHO, 2013) [7]. Actually, as expected by the WHO that road traffic Disability-Adjusted Life Years (DALYs) loss will shift from being the ninth essential cause of DALYs in 1999 to the third essential cause by the year 2020 as shown in Table 1. Whilst low- and middle-income countries confront with grave road accidents, the trend in high-income countries is the opposite. There is a severe distinction in casualty rates among high-end low-income countries [3]. Fatality rates in high-income countries have been declining, while in the low- and middle-income countries, they are still on an upward pattern. In spite of the fact that the extent of increment differs by area, tragically the most noteworthy ascent is in Asia.
S.No | 1998 Disease or Injury | 2020 Disease or Injury |
---|---|---|
1 | Lower respiratory contaminations | Ischaemic heart disease |
2 | HIV/AIDS | Unipolar major depression |
3 | Perinatal conditions | Road traffic injuries |
4 | Diarrhoeal diseases | Cerebrovascular disease |
5 | Unipolar major depression | Chronic obstructive pulmonary disease |
6 | Ischaemic heart disease | Lower respiratory infections |
7 | Cerebrovascular disease | Tuberculosis |
8 | Malaria | War |
9 | Road traffic injuries | Diarrheal diseases |
10 | Chronic obstructive pulmonary disease | HIV/AIDS |
Category of Explanatory Variables | Variable Levels | Effect | Causes More Rear-end Accidents |
---|---|---|---|
Psycho-somatic Stress | - | - | - |
Calm | - | + | - |
Nervous, or hot-tempered[8] | - | --- | Angry, aggressive drivers are a significant psychological and health hazard on the road |
Poor Health Condition | - | - | |
Anxiety and insomnia | - | -- | Causing serious accidents |
Social dysfunction [9] | - | -- | - |
Depression | - | -- | Causing serious accidents |
Physical health [8] | relationships existing between personality, emotion, and behavior, and indicating that public self-consciousness interacts with anger to influence aggression while driving | --- | Angry, aggressive drivers are a significant psychological and health hazard on the road |
Road Environment Factors | - | - | - |
Number of lanes | Others vs. 2-lane | / | - |
6-lane vs. 2-lane | / | - | |
4-lane vs. 2-lane | - | 2-lane | |
Divided/undivided highway | Undivided vs. divided | - | Divided |
Accident time | Night vs. daytime | -- | Day time |
Road surface condition [10] | Wet vs. dry | ++ | Wet |
Slippery vs. dry | ++ | Slippery | |
Light condition | ---- | - | |
Urban/rural | urban vs. rural | + | urban |
Highway character | curve upgrade/downgrade vs. straight-level | + | curve upgrade/downgrade |
curve-level vs. straight level | + | curve-level | |
straight up/downgrade vs. straight-level | + | straight up/downgrade | |
Speed limit [9, 10] | 55 mph vs. 25 mph | ++ | 55 mph |
50 mph vs. 25 mph | ++ | 50 mph | |
45 mph vs. 25 mph | ++ | 45 mph | |
40 mph vs. 25 mph | ++ | 40 mph | |
35 mph vs. 25 mph | ++ | 35 mph | |
30 mph vs. 25 mph | ++ | 30 mph | |
Check your speedometer and discover that you are unknowingly traveling faster than the legal limit. | --- | ||
Striking drivers - Driver characteristics | - | - | - |
Alcohol/drug use[11] | Alcohol under influence vs. No | + | Alcohol under influence |
Drug under influence vs. No | + | Drug under influence | |
Alcohol-Drug under influence vs. No | + | Alcohol-Drug under influence | |
Had been drinking vs. No | + | Had been drinking alcohol | |
Drink on the way back from a party, or pub, even though you realize that you may be over the legal blood-alcohol limit. | --- | - | |
Age [10] | 26–35 vs. <26 | - | <26 |
36–45 vs. <26 | - | <26 | |
46–55 vs. <26 | - | <26 | |
56–65 vs <26 | - | <26 | |
66–75 vs. <26 | - | <26 | |
>75 vs. <26 | - | <26 | |
Residence [9] | live in the local county vs. another county | - | another county |
elsewhere in the state of Florida vs. another county | - | another county | |
other states vs. another county | - | another county | |
Gender [10] | Male vs. Female | + | Male drivers |
Vehicle type | - | - | - |
Accident vehicles [10] | passenger van vs. passenger car | + | passenger van |
pickup/light truck vs. passenger car | + | pickup/light truck | |
large size vehicle vs. passenger car | + | large size vehicle | |
AADT | Average daily traffic flow | + | Higher traffic volume |
Measurement of aberrant driving behavior [11, 12] | Slips and lapses: for example, fail to read the signs correctly, and exit from a roundabout on the wrong road. | --- | - |
Errors: Applying brakes too quickly on a slippery road and/or steer the wrong way in a skid. | ---- | - | |
Violations: ➢ Being impatient with a slow driver in the outer lane and overtake. ➢ Stay in a motorway lane that you know will be closed ahead until the last minute before forcing your way into the other lane. |
--- | - | |
Machinery/mechanical problems [9, 12, 13] |
Tire failure: According to the Crash Stats report, “the tire problem is accounted for about 11.4% of the crashes” where vehicle failures were the cause of the crash making the tire failure the most common cause of a vehicle failure crash. Two of the most common types of problems with tires are tired blowouts and worn tire |
--- | - |
- |
Brakes According to the crash stats report, brake related problems as critical reasons accounted for about 15.4% of crashes where vehicle failure was the cited cause of the crash. Poor condition of brakes is a major factor in rear-end collisions, where the inability to stop in time causes a vehicle to ram right into the vehicle in front. |
--- | - |
- |
Steering and Suspension The third most-common critical reason for a car accident cited in the NHTSA report was a combination of steering, suspension, transmission, engine-related problems. This combination category accounted for just 3 % of the crashes cited in the report [9] |
--- | - |
- |
Headlights and Taillights In low visibility conditions, such as nighttime, foggy roads, or during storms, it becomes much harder to see other vehicles on the road[9] |
--- | - |
- |
Windshield Wipers Never underestimate the importance of the windshield wipers. When it’s raining hard, visibility is greatly reduced. Windshield wipers help clear rain and debris from the windshield, helping improve visibility so as to clarify the vision side.[13] |
--- | - |
Year or Period | Number of the Accidents on the 107 Road Segments (Percentage of Accidents all Over the year %) | Summary Statistics | |||
Median | Average | Max. | Std, Dev. | ||
2005 | 350(41) | 2 | 3.27 | 26 | 4.14 |
2006 | 446(50) | 3 | 4.17 | 22 | 4.87 |
2007 | 584(54) | 3 | 5.46 | 30 | 6.16 |
2008 | 482(49) | 2 | 4.50 | 25 | 5.55 |
2009 | 447(44) | 3 | 4.18 | 28 | 5.16 |
2010 | 486(44) | 4 | 4.54 | 22 | 4.84 |
2008-2010 | 1415(46) | 9 | 13.22 | 75 | 14.38 |
2.1. Traffic Accident Trends in Turkey
In Turkey, which is a more sophisticated and developed country, a day by day aggregate of 3452 road traffic accidents cause 10 individuals to die at the scene and leave 734 individuals injured (according to the statistics compiled for 2012) [14]. In 2010, the number of traffic deaths per 100,000 inhabitants in Turkey was 12, though it was 10.1 for the European Region. And in 2013, Turkey ranked 64th among the 183 WHO countries [14]. Additionally, 73% of fatal or serious accidents occur in urban areas, which are more frequent users of motor vehicles quickly. As per the Turkish Statistical Institute, among the total number of deaths and injuries, urban areas account for 4 deaths and 478 injuries, around 36% and 65% respectively in comparison to the rural areas [15]. As a typical developing country, the number of cars in Turkey is increasing, enabling people to travel more [16]. Whilst drivers need appropriate training [17], together with awareness to pedestrians about the rules of traffic in urban areas that are not strictly followed due to poor institutional association [18]. The development of the urban areas has negatively affected the layout and structure of the road. Thus, the transportation safety in Turkey is menaced [18].
In Turkey, high frequency of traffic accidents, in which thousands of people die or are severely injured every year, is a major social and economic problem. In this context, improving physical and legal infrastructure for road safety and its control are amongst the key objectives of transport policy of Turkey explicitly stated in the long-term annual policy documents of the government. Moreover, in accordance with the objective of decreasing fatalities in traffic accidents by 50% in ten years, which is stated in the United Nations (UN) Global Plan for the Decade of Action for Road Safety (2011–2020), Turkey also adopted the Road Safety Strategy and Action Plan in 2012 [19].
In this sense, Kayigisiz et al., 2015 [6] utilized basic and practical models to analyze the traffic accidents, employing comparable variables utilized by studies conducted in developed countries. The data collected at 107 road segments between the years 2008 to 2010 in Eskişehir (Turkey), involved accidents with fatalities and injuries. Table 3 shows the Accidents on the 107 road segments from 2005 to 2010. Two arrangements of models have been produced: the main set utilized paired logit models of traffic safety and the second set used count data regression models to estimate the events of various accident types. Both the sets considered three accident types: pedestrian-vehicle, vehicle-vehicle, and all accidents combined. Informative factors utilized in those models were derived from ambient land use characteristics, road segment properties, and traffic flow characteristics. Based on the results three types of direct policy implications were outlined. First, open travel should be made possible open transportation stations and by taking safety measures around stations. Second, traffic situation and road systems ought to be enhanced by planning urban configuration, road structure, and transportation configu- ration practice. Third, land use deduction should be reviewed focusing on taking precautions in areas associated with accident-increasing variables, rather than changing mixed land uses to monotonous land uses.
2.2. Traffic Accident Trends in India
Fatalities and injuries resulting from road traffic accidents are an essential and developing general medical,social as well as economical issue in India. Consistently almost 2,650 people die and 9,000 per year get injured due to traffic accidents. In 2013, the most recent year for which data is accessible, 137,423 people died and 469,900 people got injured due to road accidents in India. Traffic accidents have now made Indias traffic system a questionable with almost 140,000 deaths annually, the country has overtaken China to top the world in road fatalities. India is the only country in the world which faces an excess of 15 fatalities and 53 injuries each hour as a consequence of road crashes. While in many developed and developing countries inclusively China, the situation is getting better but, India faces a grave situation. If the trend continues, the total number of road traffic deaths in India
Country | Motorization Rate (No. of Vehicles per 1,000 People) | Fatality Rate (No. of Fatalities per 10,000 Vehicles | Fatality Risk (No. of Fatalities per 100,000 People) |
India (2013) | 130 | 8.6 | 11.2 |
Germany (2012) | 657 | 0.67 | 4.4 |
Japan (2012) | 651 | 0.63 | 4.1 |
New Zealand (2012) | 733 | 0.91 | 6.9 |
Sweden (2012) | 599 | 0.50 | 3.0 |
United Kingdom (2012) | 599 | 0.51 | 2.8 |
United States of America (2012) | 846 | 1.26 | 10.7 |
would increase to 100% somewhere in the range of 2013 and 2027.If no policy is adopted by India to curb the increasing number of accident related deaths, the total number of road traffic deaths in India is probably going to cross the mark of 250,000 by 2025 [2, 3].
Singh 2016 [3] analyzed the road traffic accidents in India at the national, state, and metropolitan city level. The focus was to identify the major road safety issues and discuss countermeasures that could have the potential to address the specific road safety problems. The primary source of data for the study was Accidental Deaths and Suicides in India, 1970 to 2013 published by the National Crime Records Bureau, Ministry of Home Affairs, Government of India, New Delhi. The analysis showed that during the last ten years, road accidental fatalities in India have increased at the rate of 5% per year while the population of the country has increased only at the rate of 1.4% per year. Due to this, fatality risk, road accidental deaths per 100,000 people have expanded from 7.9 in 2003 to 11.2 in 2013. Fatality risk in India is not only quadruple than that in some developed countries, for example, the United Kingdom and Sweden, it is extending beyond that quickly. Despite the low level of motorization, India faces a very high level of fatality risk in comparison to developed countries as indicated in Table 4. Fatality risk in India was quadruple than that in the United Kingdom and Sweden, and almost twice than that in Japan and Germany. It is obvious from Table 4 that the fatality rate in many developed countries is less than 1 fatality per 10,000 vehicles. It was found that the distribution of road accidental deaths and injuries varied according to age, gender, month and time. Among people of all age groups, people of the economically active age group of 30-59 years were the most vulnerable. However, by comparing gender-wise fatalities and accidents, we found that the males accounted for 85.2% of all fatalities and 82.1% of all injuries in 2013 [20]. It was concluded that India is facing the worsening situation. Without the necessary efforts and initiatives the total number of deaths caused by traffic accidents would likely cross the mark of 250,000 by the year 2025. There is thus an urgent need to recognize the worsening situation of road deaths and injuries and take an appropriate action.
2.3. Traffic Accident Trends in Iran
Road Traffic Accidents (RTAs) force a global health burden particularly in countries with low and middle-income where over 90% of deaths occur. There is an expected increase of 65% in the road traffic injuries during the next 20 years [21]. RTAs are a serious public health problem in Iran because an assortment of reasons including a young population of the country leads to more exposure to RTAs. RTA and its related injuries contribute to a significant proportion of the burden of diseases in Iran [22]. They do have a significant impact on the social and economic well-being of people. For example, RTAs are the third elevated cause of mortality in Iran. RTAs caused 685 611 injuries and 22 918 deaths (80%males, 20%females) annually. The worldwide road traffic injury/death rate is 3 people per 10 000 vehicles, but in Iran, it is 33 people per 10 000 vehicles [23]. Several associated factors with the risk of being involved in an RTA are classified into three types: the driver-related factor, the environmental factor and the vehicular factor. Some reports analyzed the impact of the driver license, driver’s medical conditions, the use of seat belt and helmet by the drivers as driver-related factors. Notwithstanding, there is an absence of information with respect to the relationship between driver-related components and RTAs in Iran.
Ghasem et al., 2013 [23] explored the association between driver related factors and RTAs in Iran to offer insight into the potential effects of driver-related factors on RTAs and to improve preventive measures. The cross-sectional study was conducted in Iran and all data regarding RTAs from March 20, 2010, to June 10, 2010, were obtained from the Traffic Police Department. It was observed that around 538 588 RTA records were there, which were classified to control for the main confounders: accident type, the final cause of an accident, time of the accident and driver-related factors. Driver-related factors included sex, educational level, license type, type of injury, the duration between the accident and getting the driving license and driver’s error type. A total of 538 588 drivers (91.83%male, sex proportion of relatively13:1) were involved in the RTAs. Among them 423932 (78.71%) were uninjured; 224818(41.74%) had a diploma degree. Class2 driving license represented the elevated ratio of each driving license (290 811, 54.00%). The large number of accidents took place at 12:00-13:59 pm (75 024, 13.93%). The extent to which drivers are associated with RTAs diminished from 15.90%in the principal year of getting a driving permit to 3.13% following 10 years of driving knowledge. Disregard of regulations was the commonest cause of traffic crashes (345 589, 64.17%). Non-recognition of the traffic rules was the most common cause of death (138175, 25.66% and 129352, 24.02%, individually). Ghasem et al., 2013 [23] found a significant association between the type of accident and sex, education, license type, time of the accident, the final cause of an accident, driver’s error as well as the duration between an accident and getting the driving license (all P<0.001).
It is estimated that up to 20% of traffic accidents are due to sleepiness in industrialized countries. A study showed that the risk of traffic accidents due to automobile driving between 2:00 am and 5:00 pm is 6.5 times higher. Another study reported that the peak time of accidents was at 3:00 am. Also, there is a smaller increase between 14:00 pm and 16:00 pm. Sleep and many other physiological activities are regulated by the circadian rhythm in the brain. The circadian rhythm makes two peaks of sleepiness during a period of 24 h [22]. The strongest level of sleepiness occurs during the night (between midnight and 6:00 am). The second peak, which has less severe sleepiness, occurs in the afternoon between the hours of 13:00 pm and 16:00 pm. Also, the time of maximum alertness and little desire to sleep is between 18:00 and 22:00. Another important point is that in traffic crashes caused by sleepiness, usually only one vehicle is involved (single-vehicle crashes). In these cases, the accidents often occur while overturning [21].
Khosro et al., 2015 [24]studied the relationship between road traffic accidents due to sleepiness in Iran. All the road traffic accidents relevant to sleepiness, which were reported by police, were studied in Tehran province in 2009. The risk of road traffic accidents due to sleepiness was increased by more than sevenfold (odds ratio= 7.33) in low alertness hours (0:00-6:00) compared to other times of the day. The risk of road traffic accidents due to sleepiness was decreased by 0.15-fold (odds ratio = 0.15) in hours with the maximum of alertness (18:00-22:00) of circadian rhythm compared to other times of the day. The occurrence of road traffic accidents due to the sleepiness has significant statistical relations with driving during the lowest point of alertness of circadian rhythm.
2.4. Traffic Accident Trends in Indonesia
Research and statistical figures often conclude that traffic accidents are an iceberg for comprehending the discrepancies of traffic management and entire transportation systems. Figures specifying traffic accidents in Indonesia, as is the case in many other countries, show essentially high numbers and severity levels; These kinds of totals are also obvious in Jakarta, the most densely populated city in the country [1]. Indonesia presently has a population of more than 230 million people, and the country's economic development has given rise to increments in transportation demand and vehicle ownership. Comparable figures are also obvious in Jakarta, the capital which has a population of nearly10 million people. The figures are significantly higher in the daytime because of support from suburbanites from the distant territories and some supporting urban cities. Jakarta may have a population of around 12 million people.With a road ratio of only lower than 7%, the raised vehicle growth rate and the needed public transportation contribute to raising -density of road utilizeation. This tricky circumstance is difficult to determine as over 90% of the accessible transportation modes are road users [1, 25].
According to Soehodho, 2017 [26, 27] traffic accidents are often caused by three different factor types, namely, human factors, vehicle factors, and external factors (including road conditions). Human factors have the strongest influence, as figures from around the world suggest. Our focus is non-human factors in Indonesia. We focused particularly on the poor growth of road infrastructure (a reduced road proportion), poor public transportation, and the adverse phenomena of motorbike ownership and its reckless use. The road network infrastructure in Indonesia has encountered conservative evolution for a long time, and a few urban cities have, expounded much needed road network rehabilitation. These states showed an exclusive rise in traffic as well as higher numbers of traffic accidents. Fig. (1) shows the numbers of traffic accidents in Indonesia meanwhile, the last ten years (2004–2013). The table also indicates several distributions of accident severities: fatalities, severe injuries, and light injuries. Although fatalities and intense injuries are parallel to light injuries, their numbers and trends are still rising.The most common form of public transportation in extreme Indonesian cities is the bus, whose systems range from small to large.
Traffic accidents in Indonesia, and essentially in large cities such as Jakarta, exhibit an upward trend. Whilst human factors have a strong effect, there is another phenomenon that exacerbates the traffic accident issue is the motorbike model. The quickly growing preference for the motorbike mode underscores a supply-demand imbalance in transportation systems in many Indonesian cities, in addition to other factors like the lack of sufficient road infrastructure [28, 29]. Providing sufficient and affordable public transportation is an effective means of combating motorbike use and, by extension, reducing traffic accidents. Development projects for some methods of public transportation have been ongoing in Jakarta and being followed by other considerable cities in the country with comparable aims and targets. It is further expected that various funding sources include the private sector and not only national and sub-national budget resources that will accelerate the frugality of public transportation and improve overall traffic safety [25].
2.5. Traffic Accident Trends in Thailand
According to a worldwide comparison of traffic accident mortalities, it was found that Thailand ranked 70th among 178 countries in the world in the total number of deaths calculated to be approximately 19.6 deaths/100,000 people. The report of the department of health, Thailand B.E.2554 (2011) illustrated that traffic accident is the second leading cause of death in Thailand, after cancer disease, which is the primary cause of injuries [30]. The total cost of traffic accidents in Thailand for the year 2004 was estimated to be 153,755 million baht. Although the government has issued some policies to prevent and control accidents, the numbers of injuries and deaths have continued to increase. The Expressway Authority of Thailand (EXAT). adivision of the Ministry of Transport, takes responsibility for the management and execution of the expressways. The EXAT has a consolidated vision of expressway safety and provides regular road safety audits and black spot treatment. However, the number of accidents on the expressways is still too high as indicated by Vatanavongs and Sonnarong, 2014 [31] from the record in B.E,2553 (2010) of 1878 crashes with 20 deaths and 573 injuries. Nevertheless, road accident prediction is quite difficult because the causes of accidents are generally brought on by various factors such as road user behaviors, vehicle conditions, physical road charact-
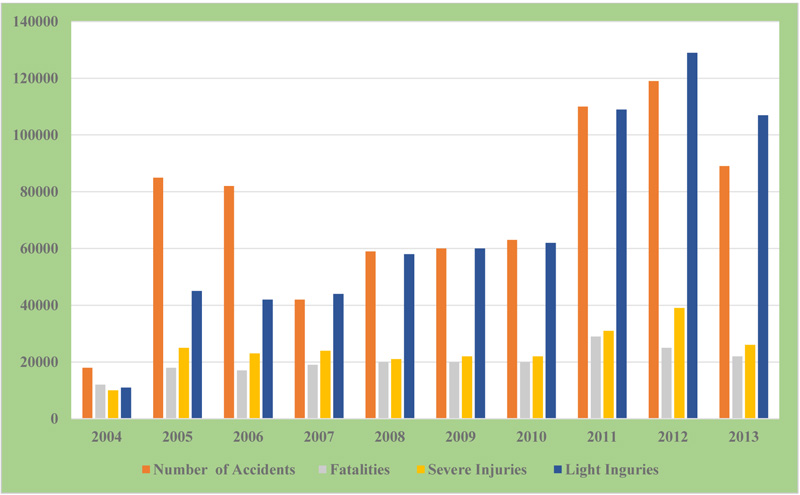
eristics, and the environment, even with the improvement of physical road conditions. Therefore, it is vital to obtain accident data to formulate a statistical model for the purpose of providing an explanation for and forecasting the probability of crashes, as well as finding the factors that might affect the accident severity. This effort will benefit the responsible authorities in well-considered black spot improvement and road safety audit consequence to prevent or reduce any damages caused by accidents [30-32].
Vatanavongs and Sonnarong, 2014 [31] accessed accident data on expressway networks during 2007 to 2010 (updated data were collected), in which Expressway Authority of Thailand (EXAT) as legislatively mandated unit took accountability for the enforcement of nine expressway routes covering distances totalling over 207 km with a record of 2194 crashes. The main objective of the study was to forecast the accident severity through formulating Multiple Logistic Regression Model to analyze the probability of injuries by accident and the fatal accidents in comparison with property damage only. Its measurement comprehensively considered the statistical relationship among variables such as average speed on the road section, average traffic volume per day, a period of time, weather conditions, physical characteristics of accident area, and causes of the accident. Together, the research question was to verify the effect of the variables on the probability of the accidents and to investigate the impacts of the losses in an accident. Their findings verified that speed is the only factor influencing the severity of crashes on an expressway; hence, the speed limit on an expressway should be mandated for drivers by carrying out strict inspection of expressway-speed under the regulations. Also, increasing awareness of accident severity caused by driving at excessive speeds should be promoted by authorities to potentially reduce the risks and levels of accident severity. In addition, the study suggested that further research should focus more on the issue of identification of speed limit design in accordance with the roadway geometric features for drivers safety on expressways to efficiently tackle the accident severity problems.
2.6. Traffic Accidents Trend in Africa
The causes of road traffic crashes (RTCs) are multi-factorial and involve the interaction of a number of pre-crash factors that include humans, vehicles and road environment. Many studies have been conducted to investigate and understand the factors that contribute to RTCs in order to provide countermeasures. In Ethiopia, the fatality of road traffic accidents is considered to be a man-made disaster. The Ethiopian National Road Safety assortment Office cites a road crash fatality rate of 114 deaths per 10, 000 vehicles per year but the factual figure may be higher due to an incorrect reporting system. It has been signified that many road accidents can be prevented and that road safety is ultimately a development problem for considerable number of countries. Eighty % of road traffic deaths occur in middle-income countries, which account about 85% of the mortalities and 90% of the inability adjusted life years (DALY) caused by RTAs worldwide. The hazard of dying as a result of a road traffic injury is the highest in Africa (24.1 per 100,000 population) and 38% of all African road traffic deaths happen of pedestrians [33]. The issues are rising in vehicles in African countries due to rapid motorization and other factors. Overall, in Egypt 64%, in Tunisia 58%, and in Morocco 51% were injury- concerning deaths in 2008. In Libya 43%, in Djibouti 42%, in Namibia 36%, and in Niger, 34% were road traffic accident concerning deaths. The most economically active people (aged 15–59) are at the paramount hazard of dying accordingly a result of RTAs. For this age group, road traffic accidents influenced more than three times as many males as females. Overall, 5% of deaths among males aged 15–59 are attributable to road traffic accidents, but this percentage rises to 6.5% for males in the 15–29 age group in Sub-Saharan Africa [33, 34].
For instance, Ojo, 2014 [35] examined the factors contributing to road traffic crashes in Ekiti State, Nigeria using linear regression analysis and found that over speeding (speed violation), drivers’ distraction and dangerous overtaking contributed significantly to road crashes in the State. Ogunmodede et al. 2012 [36] found that over speeding, wrong overtaking, bad condition of roads, sudden mechanical defects, alcoholic intake, a tire burst, and heavy rainfall contributed to the increasing rate of motorcycle road crashes in Oyo State, Nigeria. Haadi 2014 [37] analyzed the factors that contributed to road crash severity in Ghana’s Northern Region using binary logistic regression. The study found that overloading and obstruction were the two most significant factors contributing to road crash severity in Ghana. Also, brake failure has been found by previous studies to be one of the factors that have contributed to road traffic crashes in developing countries [38].
Chimba et al., 2017 [39] analyzed road traffic crashes in Anambra State, Nigeria with the intention of developing accurate predictive models for forecasting crash frequency in the State using the autoregressive integrated moving average (ARIMA) and autoregressive integrated moving average with explanatory variables (ARIMAX) modelling techniques. The result showed that ARIMAX model outperformed the ARIMA (1,1,1) model generated when their performances were compared using the decrease Bayesian information standard, mean ultimate percentage error, root mean square error; and higher coefficient of determination (R-Squared). The findings of this study revealed that incorporating human, vehicle and environmental factors in time series analysis of the crash dataset produced a more robust predictive model than solely using aggregated crash count. This study provided knowledge on road traffic safety and an approach to forecast accidents using many humans, vehicles and environmental factors. The recommendations made in this study if applied, will help in decreasing the number of road traffic accidents in Nigeria.
2.7. Traffic Accidents in Developed Countries
The road accidents were established as a major cause of worldwide deaths, as well as physical disability. Recent data presented by the World Health Organization showed that evidence clearly. Reduction of road accidents must, therefore, represent a concern for everyone, being perfectly legitimate under the Decade of Action for Road Safety (2011-2020), where European Union has established a goal to reduce 50% of the number of victims in member countries by 2020. Aligned with world-defined guidelines, Portugal took the challenge of being placed among the top ten countries in Europe with the lowest accident rate. In the recent resolution of Ministers Council on 5/2014 and under the Mid-term review 2013-2015, it is defined as a goal for the future of Road Safety in Portugal to attain a result which, in the long term, aims to achieve zero fatalities and zero severe injuries [40].
Beverly et al., 2018 [41] investigated traffic accidents in Scottish Military Veterans. They used data from the Scottish Veterans Health Study to examine the risk of road traffic accidents (RTA) in a large national cohort of veterans, in comparison to people who had never served. It was conducted as a retrospective cohort study of 57,000 veterans and 173,000 non-veterans, followed up for up to 30 years, using survival analysis to compare the risk of RTA injury. Subgroup analysis was utilized to explore trends by birth cohort and length of service. Overall, veterans had a higher risk of RTA Cox proportional risks ratio (HR) 1.17, 95% confidence intervals (CI) 1.14–1.20. The danger was elevated in the veterans with the shortest service (early service leavers), including those who did not complete premier military training (HR 1.31, 95% CI 1.23–1.40). The mean age at first RTA was 34 years. However, the veterans were born in the 1960s contributed to a higher risk compared with those who were born in 1970s. It was, therefore, demonstrated that the increased risk of RTA observed in serving military personnel persists in veterans in the fourth decade of life. The high risk in those who quit the service earlier was attributed to the previous childhood adversity. Recent Ministry of Defense road safety programs may now be reducing the long-term risk of RTA injury.
Chaturabong et al. (2011) [30] estimated the value of statistical life (VSL) and the value of statistical injury (VSI) for motorcyclists in Thailand using the willingness to pay for WTP-CV approach. This approach has been exceedingly used in many sophisticated countries. The WTP method or the value of risk modification is utilized to assess the value that individuals would pay for decreaseing the danger of loss of life. This approach was principally based on survey design to determine the amount individuals would pay. However, efforts to apply the WTP approach in estimating the costs of road accidents in Thailand have been exceptional. One is the contingent valuation (CV) method, which has been, the choice to measure the WTP in this study. The Contingent Valuation (CV) is a commonly used technique for measuring non-market values. Questionnaire surveys were conducted in Bangkok and at surrounding areas in several different locations, such as universities, schools, private companies, and government offices. Participants included 1015 motorcyclists who were randomly selected. The mean WTP values were calculated using simple arithmetic means, and the factors influencing WTP were observed using regression analysis. The estimated VSL ranged from $0.17 million to $0.21 million, and the VSI ranged from $0.08 million to $0.10 million. Individuals with lower incomes, older people, and male motorcyclists were less willing to pay, while government officers and motorcyclists who often wore a helmet were more willing to pay for their fatality risk reduction.
Mon et al. (2018) [42] approximated fatal injury costs due to road accidents in Malaysia using the WTP with the conjoint analysis (CA) design technique. 4,000 respondents, including car drivers and motorcyclists, were interviewed in 13 states of Malaysia. The factors influencing the WTP were analyzed using linear regression analysis. Income, vehicle ownership, occupation, race, risk perception, gender, and accident experience were statistically significant and influenced the WTP. The estimated VSL ranged from $0.36 million (MYR 1.15 million) to $0.45 million (MYR 1.45 million) using the CA, and that value was comparable with the VSL of the previous study using the CV approach.
2.8. Traffic Accident Trends in Jordan
Jordan is considered as one of the dominant world countries in terms of traffic accidents, which was accountable for about 4.5% of fatalities during the year 2007. Furthermore, it was found that the costs of road accidents in Jordan were estimated to be about U.S.A$ 146.3 millions in 1996 which increased to the U.S.A$ 440 millions in 2010 (WHO, 2013) [7]. Thus, this problem was taken seriously by the governmental authorities that are concerned with traffic and road safety. In 2007, the government of Jordan applied a new traffic law with massive activities of police and applied very high fines on the most dangerous traffic violations, and shortly replaced it by another law with reduced, but still a comparatively rise in traffic violation fines in 2008. Furthermore, the Jordanian government implemented a traffic safety strategy starting the year 2008 which included the following measures [43]:
- Implementing the new 2008 traffic law
- Growing execution measures and quality of traffic police
- Coordination with other traffic safety agencies
- Investigation of dense traffic consciousness activities through media and educational foundation.
Bashar et al., 2013 [44] utilized the traffic accidents data in Jordan for a period of thirteen years (1998-2010) to mainly investigate their trends and characteristics over that period. Based on the available data, traffic accidents were analyzed considering several variables including accident type, driver age, speed limit, time of the day, the day of the week, month of the year, climate condition, pavement surface condition, and severity level. It was observed that the numbers of traffic accidents increased over the study period (1998-2010) with a total increment of 223% as a result of increased population, auto ownership, and motorization level (registered vehicles/1,000 populations). Analysis of traffic accident types over the study period showed that an average of 88% accidents of collision type with an increasing trend. 6.3% accidents inlvoved pedestrian with a decreasing trend, and 2.7% were due to wrong turnings with a decreasing trend. The severity level for traffic accidents (number of casualties/ number of accidents) showed a decreasing trend over the study time. The number of fatalities/10,000 registered vehicles showed a decreasing trend, while the number of fatalities/100,000 population showed a rising trend till 2007 and consequently started decreasing. The plurality of traffic accidents occurred through the time period 12:00 – 5:00 PM, with the highest severity levels in the time period (3:00 - 6:00 AM). The largest numbers of traffic accidents occurred on Sundays and Thursdays (the first and last working days in Jordan), while accidents on Friday (the national holiday) had the highest severity level.
2.9. Traffic Accident Trends in Iraq
Iraq is one of the main Arabic countries with an overall area of 438,000 Sq. km and the total population of about 37 millions; around 70 percent of the population live in urban areas. Geographically, Iraq is with latitude and longitude of 33° 00' N and 44° 00' E respectively and regionally is located within the Middle East in Southwest Asia. Major cities include the capital Baghdad, Al-Mosul, Al-Basra and Al-Sulaymaniyah. With over 59,600 km total paved roadways and 4.5 million total motor vehicles, road transport is the essential means of mobility for people and goods (Ali et al., 2018). Road accidents and severity have been the issues for many years in Iraq on the highway. This challenge has become a life-threatening issue after the continuous steep rise in vehicle ownership and their use after 2003 onwards.When the economy started to recover after the gradual removal of economic sanctions imposed by the UN Security Council against Iraq. Furthermore, this rise can be an essential contributing factor to vehicle collisions especially when no effective management programs are adopted [45] Fig. (2) shows the month-wise distribution of road accidents in Iraq. Although the monthly variation in road accidents was not massive, road accidents were relatively high in August -September, and October-November, December. This is related to the extreme weather since the temperature is fairly hot in August-September in Iraq, hence it might affect traffic accidents. High temperatures have both psychological and physiological effect on drivers. Frustration increases with the high temperature, as people get more irritable to others, they get tired, lose their concentration, and as a result, they cannot smartly deal with any haphazard situation on the roads (Bijleveld and Churchill, 2009; Baratian et al., 2014) [3, 46]. This is the reason for growth in traffic accidents in summer, especially in July. In spite of the occurrence of road traffic accidents in August-September, accidents were not as high as in July-September. Generally, the rate was higher than in other months. It may be attributed to the fact that a certain part of the country particularly North Iraq suffered from poor visibility of the roads during December due to the hazy weather condition. Generally, people drive somewhat slower and it becomes difficult to main a safe distance from the vehicle in front of them in hazy weather. The diminished field of vision during the hazy weather increases the danger of accidents (Baratian et al., 2015.) [47]. Traffic accidents massively took place in July due to the a high ratio of students in schools, and universities in the main and branch roads causing more traffic accidents. Fig. (3) shows the cumulative percentages of time-wise distribution of road traffic accidents in Iraq. It clearly reveals that there is a substantial variation in road accidents during the different time interval in the day. Accidents were relatively constant and peaked from 6:00 am to 6:00 pm but decreased during mid-night and early hours of the day. However, it does not indicate that daytime driving is riskier than nighttime driving. Fig. (4) illustrates the number of accidents for the fifteen governorates in Iraq during the study period 2005 and 2011. There is a distinct gap in accident risk ratio among the fifteen governorates in Iraq. Governarates varied from 71 accidents in Anbar to 987 accidents in Baghdad, 1441 in Babil, 1173 in Al-Najaf, and 1154 in Basrah in 2010. In 2011, fatality risk for Baghdad, Babil, Najaf, Qadisyah, Thi Qar, Wasit, and Basrah was higher. From 2005 to 2011, the number of accidents in fifteen governates covered in this study dramatically increased. However, Baghdad city showed a higher result for traffic accidents and fatality rate followed by Babil, Najaf, and Basrah [45].
The traffic accidents related mortality rate in Iraq was 20.2 in 2013. According to the WHO report Iraq ranks 113th in the globe this rate is moderately higher than the worldwide rate of 17.4 [33]. The traffic accidents in Iraq had negatively affected public health and the security of the Iraqi society. Fig. (3) and Fig. (4) show the severity of traffic accidents in Iraq [45].
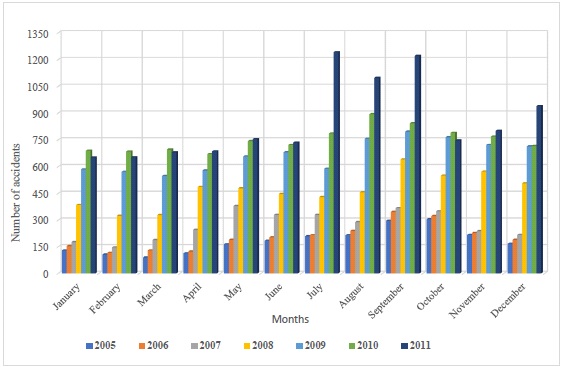
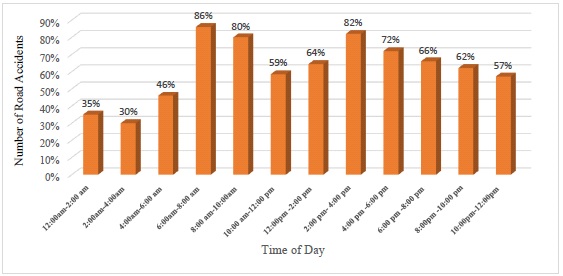
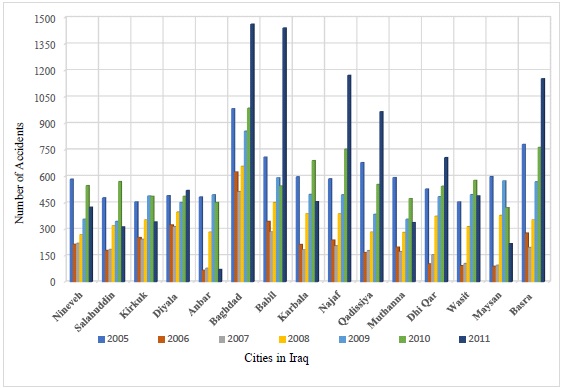
Secondary factors may include adverse weather conditions, such as frost, fog, rain sandstorm, high temperature during the summer season, and other related factors. Human error is the major cause of auto accidents in Iraq. Ismail 2012 [48] indicated that more than 80% of the accidents are due to human error. Roads are full of irresponsible drivers who break traffic rules deliberately or ignorantly. Major traffic violations in Iraq include over speeding, improper overtaking, wrong turning, and stopping. Pedestrians are also at fault because many of them do not abide by traffic rules and regulations and do not follow the instructions necessary for their own safety. Poor roadway conditions are to be blamed for about 10% of traffic accidents in Iraq. Some accidents were attributed to mechanical failure caused by poorly maintained vehicles and oversize loads carried by vehicles. The percentage of accidents due to vehicle failure is more than 10% of traffic accidents [45].
The author of this paper has made frequent visits and conducted with the traffic officers in General Traffic Directory in Baghdad so as to diagnose the fundamental deficiencies in traffic system in Iraq highlighting the safety problems and making the mechanism of accident prevention in Iraq more efficient. The weak points in the Iraqi traffic system are addressed below:
- The procedure of reporting traffic accidents: It includes recording and reporting the accidents, followed by the treatment and analysis process. Without complete and detailed information about traffic accidents, the studies will not be detailed and accurate. At the present time, the value of information that is recorded during the time of accidents depends on the experience of the person who makes this report. The reports that officers make are inadequate. Besides, they do not give the actual causes of accidents. Therefore, a new procedure must be followed in recording the accidents so as to follow a proper way to discover the actual causes of accidents.
- Referencing Systems: The accident location is not identified accurately in the accident reports because of the absence of effective information that locates it properly.
- Lower Safety Standards: These include, such as poor night visibility, absence of signs, road markings, signals, and other traffic controls. Fig. (5) indicates the poor planning for the traffic system in a typical street where neither traffic signals nor traffic signs exist. Besides, less awareness of the public about the importance of traffic rules plays a role in increasing traffic accidents. Furthermore, the erection of concrete barriers on the vital streets in Iraq decreases the width of the streets.This,with the increasing number of vehicles will definitely increase the traffic crashes as it is illuminated in Fig. (5) and Fig. (6).
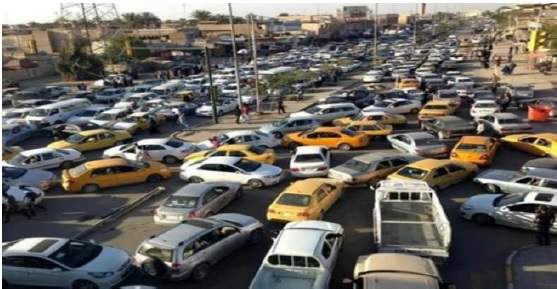
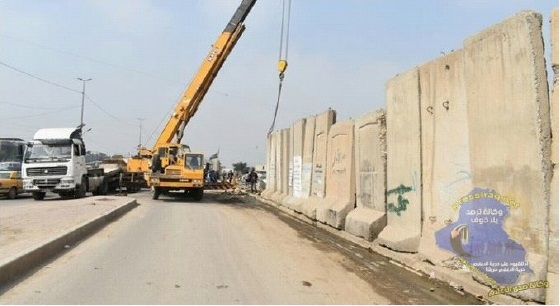
Ali et al., 2018 [45] analysed the accidents in Iraq through the period (2005-2011) for the fifteen governorates in Iraq. Data on a number of traffic accidents per governorate were obtained from the General Traffic Directorate with cooperation by the Ministry of Planning/the Central Statistical Organization (CSO) in coordination with the Ministry of Interior in Iraq. Traffic accidents analysis was performed based on a number of factors like gender, age of the driver, light condition, type of accident and status of the road. Reasons for traffic accident were also illuminated based on the driver, the road, the passenger, and the pedestrians to verify the cause and effect of traffic accidents in Iraq. Results showed that the reasons of traffic accidents deaths and injuries in Iraq varied according to age, gender, month and time. Overall, traffic accidents dramatically increased from 2005 to 2011. Moreover, road accidents were observed to be relatively higher in extreme weather during working hours. Analysis of road traffic accidents based on light condition showed that the accidents rate significantly decreased in the night. Collision accidents were the highest in Baghdad and Babil city. However, the results were slightly approximated for the rest of the cities. Ratios for the car coup accidents were higher in Al- Qadissiya, Baghdad, Babil, and Basrah city for the wet road. It indicates the absence of security precautions especially during rainy days hence vehicles will be slipped accordingly. It was observed that the highest accidents ratio was attributed to the driver and it was 36% for Baghdad city and the number of accidents was 2379. Whilst Nineveh city registered fewer accidents being 22% and totaling 586 in number. Results for traffic accidents based on the type of the vehicle indicated that saloon vehicle resulted in a higher rate of traffic accidents up to 26.3%. Baghdad city had a higher ratio than the other cities, the number of accidents in Baghdad was 1234 while the number of accidents was significantly less (425) in Al-Anbar city.
Bassam et al., 2015 [49] analyzed and evaluated the causes of traffic accidents by using the statistical analysis technique, questionnaire survey, and self-observation study so as propose a management countermeasure for common accident locations on principle urban streets in Arbil City as a guide for traffic safety management program. Traffic accident data, geometric condition data, and traffic condition data, related to Arbil streets network were collected, prepared and analyzed by using the Statistical Package for Social Sciences (SPSS) program and Minitab program. Different predictive statistical models were developed for the streets in Arbil urban area. The Accident prediction models could be used to predict the probability of an accident at a certain location based on different variables. It was concluded that most of the traffic accidents were attributed to some of the geometric and traffic conditions.The questionnaire survey analysis showed that five elements for traffic accidents were: road element, road user element, vehicle element,environmental factors elements, and other factors element. It was concluded from this study that the increase in the number of segments in principle urban streets would increase the number of total accidents and the number of property damages. Besides, it was found that pedestrian crossing areas were ignored by the pedestrians and the drivers did not consider giving time to pedestrians for cross the road, due to lack of traffic awareness by drivers and pedestrians. The study recommended that roads should be designed and constructed according to international standards. Also, it was recommended to improve traffic awareness of road users: drivers, pedestrians, and passengers through organized educational awareness programs.
3. TYPES OF TRAFFIC ACCIDENTS
Traffic accidents occur when a vehicle collides with another object. They are caused by road obstruction, pedestrians, crossing or lingering animal or a stable impediment such as a tree or a utility pole. The common kinds of traffic accidents are vehicle rear-end collisions, side impact collisions, vehicle rollover, sideswipe collisions, head-on collisions, single car accidents, and multiple vehicle pile-ups [50-51].
- Rear-end collision: A rear-end collision occurs when one car hits the rear of the car in front of it. A rear end collision generally happens when the vehicle ahead unexpectedly decelerates or when the vehicle behind unexpectedly accelerates. Injuries to the passengers of the impacted vehicle are generally higher, than those of the passengers of the impacting vehicle.
- Side-impact collision: Side impact collisions occur when the side of a vehicle is hit by the front or rear of another vehicle. Side impact collisions result in more severe injuries and vehicle damage. However, injuries and damage may be dangerous depending on how credible the safety features of vehicles are such as airbags, crumple zones, vehicle construction, and materials. However,sideswipe collisions are collisions involving the adjacent sides of two vehicles. Sideswipe contacts usually result only in cosmetic damage of the car.
- Rollover a vehicle rollover occurs when a vehicle flips over its side or roof and it usually happens when the vehicle makes a high-speed sharp turn.Vehicles with height SUVs are usually more susceptible to a rollover accident since they have a high center of gravity.
- Head on collisions Head on collisions are considered fatal especially when these collisions involve a vehicle traveling at a very high speed.
- Single car accident A single-car accident is also one of the many kinds of vehicle accidents but only involves one vehicle. These accidents occur when the driver loses control over the vehicle due to drowsiness and faling a sleep, or when brake malfunction occurs.
- Multiple vehicle pile-up A multiple vehicle pile-up is an accident involving many vehicles usually happening in freeways or highways. Damage to many vehicles and lives makes this type of accident one of the deadliest traffic accidents since several vehicles are not only hit once but multiple times and from various directions. Cars even spin during a pile-up accident increasing the risk of serious injuries and loss of lives of passengers. Those who decide to run away at once from their vehicles are still at the hazard of being hit by other upcoming vehicles. The cause of multi-vehicle collisions is often hard to determine.
4. CRASH PREDICTION MODELS
To enable efficient allocation of tight resources, new knowledge and instruments are needed for the detection of unsafe road sections and for the estimation of the effects of safety (design) measures. Crash prediction models (CPMs) can be used to estimate the crash frequency on-road section related to the traffic flow, road length and risk factors such as cross-sectional design elements (further referred to as road characteristics). These models can help in investigating the quantitative relations between road characteristics and crashes; they can be used for identifying relative unsafe road section in a network and for estimating the effects of the implementation of safety measures on a road section [52]. The development of CPMs is, however, not a straightforward exercise. It is known that the road length and traffic flow (the exposition to danger) are major components in explaining the crash frequency on a road. A generally accepted theory of how the crash frequencies relate to traffic flow, however, does not seem to exist in the field of crash analysis as.stated in study [38]. Furthermore, problems can be expected with regard to the research database. High-quality data in large quantities for road characteristics, traffic flow, and crashes are rarely readily available and compatibility issues arise when combining different datasets of road characteristics and traffic flow [53].
4.1. Crash Prediction Models Based on Geometric and Traffic Features
Yannis et al. 2010 [54] improved multilevel negative binomial models to inquire into the effects of the strength of the police enforcement on the number of road accidents during 1998-2002 at the regional level in Greece.Nikiforos et al. 2010 [55] aimed at evolving crash prediction models and Accident Modification Factors (AMF) for multilane roads particularly lane widths, type, median width, and shoulder width. They noticed that rising shoulder width had a positive effect on crashes. He opined that shoulder width caused a reduction in crashes for divided highways. Quddus et al., 2010 [56] examined by searching the relationship between the severities of road crashes and the level of traffic difficulties using ordered response models. They collected data between 2003-2006 related to traffic characteristics, crash data and road geometry for M25 London orbital motorway. They opined that the traffic crowding does not influence the severity of road crashes on the M25 motorway.
Wagamama and Dissanayake 2010 [57] verified the influence of accident-related factors on road fatalities taking into account Bali province in Indonesia as a case study. Logistic regression models for fatal accidents were improved. They employed seven predicting variables in the generated models. Through their study, they observed the eventuality of accidents pertaining to female motorcyclists and motorized scooters as 79% and 72% than males. It was revealed that all the vehicle fatalities were related to the age of the drivers being 50%. Hauque et al., 2010 carried out a detailed study on sever crashes involving motorcycles as vehicles. The main focus of their study was to assess how behavioral factors influence the severity crashes and to recognize the utmost vulnerable group of motorcyclists. A questionnaire for 61 items of risk-taking behavior was generated.
is useful for predicting a binary dependent variable as a function of predictor variables. The goal of logistic regression is to identify the best fitting model that describes the relationship between a binary dependent variable and a set of independent or explanatory variables. The dependent variable is the population proportion or probability (P) that the resulting outcome is equal to 1. Parameters obtained for the independent variables can be used to estimate odds ratios for each of the independent variables in the model
Logistic regression models are useful for predicting a binary dependent variable as a function of predictor variables. The goal of logistic regression is to identify the best fitting model that describes the relationship between a binary dependent variable and a set of independent or explanatory variables. The dependent variable is the population proportion or probability (P) that the resulting outcome is equal to 1. Parameters obtained for the independent variables can be used to estimate odds ratios for each of the independent variables in the model proposed by Ali et al., 2018 [58]
The specific form of the logistic regression model is:
![]() |
(1) |
The transformation of conditional mean π (x) logistic function is known as the logit
transformation. The logit is the LN (to base e) of the odds, or likelihood ratio that the
dependent variable is 1, such that
The transformation of conditional mean π(x) logistic function is known as the logit transformation. The logit is the LN (to base e) of the odds, or likelihood ratio that the dependent variable is 1, such that
![]() |
(2) |
Where: Bo: the model constant Bi: the parameter estimates for the independent variables Xi: set of independent variables (i = 1,2,.........,n) P: probability ranges from 0 to 1
: the natural logarithm ranges from negative infinity to positive infinity
The logistic regression model accounts for a curvilinear relationship between the binary choice Y and the predictor variables Xi, which can be continuous or discrete. The logistic regression curve is approximately linear in the middle range and logarithmic at extreme values. A simple transformation of equation (1) yield
![]() |
(3) |
The fundamental equation for the logistic regression shows that when the value of an independent variable increases by one unit and all other variables are held constant, the new probability ratio [Pi/(1-Pi)] is given as follows:
![]() |
(4) |
When the independent variable X increases by one unit, with all other factors remaining constant, the odds [Pi/(1-Pi)] increase by a factor exp iB. This factor is called the odds ratio (OR) and ranges from 0 to positive infinity. It indicates the relative amount by which the odds of the outcome increase (OR>1) or decrease (OR<1) when the value of the corresponding independent variable increases by 1 unit [59, 60].
There was no true R2 value in logistic regression, as in Ordinary Least Squares (OLS) regression. Alternatively, Pseudo R2 can be a proxy of an R2 including Cox & Snell Pseudo-R2 and Nagelkerke Pseudo-R2
![]() |
(5) |
The null model includes only the constant while the k model contains all explanatory variables in the model. Cox & Snell R2 value could not reach 1.0 so that Nagelkerke was used to revise it, including Cox & Snell Pseudo-R2 and Nagelkerke Pseudo-R2 by Ali et al., 2018 [58, 60].
![]() |
(6) |
A Hosmer-Lemeshow Test is used to carry out the goodness of fit measure. The null hypothesis for this test is that the model fits the data, and the alternative is that the model does not fit. The statistical test was conducted by first dividing the data set into roughly 10 (g) groups. The groups were constructed by ordering the existing data by the level of their predicted probabilities [10, 60]. The data were ordered from least likely to most likely for the event. The equally sized groups were formed. From each group, the observed and expected number of events were computed. The test statistics are,
![]() |
(7) |
Where: Cˆ = The Hosmer-Lemeshow test (H-L test) Ok = an Observed number of events in the kth group Ek = Expected number of events in the kth group vk = Variance correction factor for the kth group.
If the observed number of events differs from what is expected by the model, the H-L test will be large and there will be evidence against the null hypothesis.
Chio et al., 2010 [61] studied the contributing factors to crash severity in freeways of Taiwan which were considered as rural roads. They took many variables such as occupation and age of the driver, travel period, travel purpose, location, vehicle type, action of the driver, surface condition, signal control, driver gender, weather, stumbling on road, lighting conditions, collision type, severity, speed limit, road status, marking, authorization, etc. The developed model collision type, the purpose of the journey, major cause, and travel period are 4 significant factors determining the severity of accidents and these 4 factors are necessary for best traffic safety evaluation. Fujita and Mustakim et al., 2011 [62] produced an accident forecast model for the rural roadway depending on the data collected in Malaysia on a rural roadway. They conducted a black spot study to develop accident prediction models by using multiple nonlinear regression methods. The outcome has shown that major access points such as the rise in speed, absence of traffic light, and the rising number of Annual Average Daily Traffic (AADT) are the substantial contributors to accident rates on multiple rural roads. It was concluded that the vehicle speed (AS), Annual Average daily traffic (AADT), Motorcycle (MC), motor car(C), the Gap (GP) and the total length of the Accident section (TL) were significantly contributed to the accidents at four lanes and two-lane undivided rural road.
4.2. Crash Prediction Models Based on Access Road and Segment Length
Kay et al. 2010 [63] evolved a horizontal curve Accident Modification Factor (AMF) for undivided and four-lane divided highways. The elements they took into consideration were: lane width, shoulder width, median width, median type, driveway density, average daily traffic, segment length and degree of the curve. Five years crash data from 1997 to 2001 was collected and models were developed for the curve and tangent. They opined that the driveway density, median width and outside shoulder width were observed to be important factors when both the driveway and non-intersection crashes were considered. Lovegrove et al. 2010 [64] prepared a prediction system where the data was taken from different individuals’ groups. The observation analysis showed that factors such as traffic flow characteristics, geometry and weather had a statistically significant association with traffic accidents.
The single vehicle model includes traffic flow, length of driver’s path on the geometric element, 85th percentile speed on a previous geometric element and radius of the geometric element as explanatory variables. The following equation was fitted proposed by Ali et al., 2018 [58-60].
![]() |
(8) |
Eμsingle = expected annual single-vehicle accident frequency
Q = Annual Average Daily Traffic (AADT) in the direction considered
L= length of vehicle path on the geometric element (m)
S = 85th percentile speed on the geometric element (km/h)
ΔS = decrease in S at the start of the geometric element (km/h)
R = radius of the geometric element
Salvatore; et al. 2010 [48] modelled accident models for two-lane rural highways using exposure geometry, consistency and context variables. In Italy, in over 5 years duration, they collected data of 279 accidents with a total of 640 injured individuals and 16 fatalities. They evolved 19 models and recommended 3 models. Model-1 included only length and traffic volume Model-2 Included driveway density, curvature ratio, the standard deviation of the operating speed profile, length, traffic volume (AADT),while Model-3 included driveway density, roadside hazard rating, length, traffic volume (ADT), speed differential higher than 10Kmph and curvature ratio. They noticed that –AADT accidents were nonlinear and that decreased with increasing curvature ratio and increased with the increasing standard deviation of speed.
Naqvi et al. 2018 [65] studied motorcycle accidents taking into consideration personal and environmental factors. They accepted variables such as traffic movement, the road character, junction type, day, age, lighting conditions, surfaces conditions, and driving behavior. They used logistic regression to predict the occurrence of an accident from the variables taken into consideration and evolved a logit model [66]. According to the study, three elements were identified to be more important predictions of motorcycle accidents. They were, the junction type age of driver and driver behavior. They used walds and Hosmer-Lemeshow test as logistic regression. They opined that the younger drivers were more involved in accidents.
Obaid et al., 2012 [50] conducted a study on the traffic accident at 28 hazardous at Amman –Gordon urban roads. They noticed that the logarithmic and linear models were the most important and realistic models that can be used to predict the relationship between the accident characteristics and dependent variables. The following variables were considered to be the most significant contributors to a traffic accident in serious places such as: the number of vertical curves, median width, type of road surface, average running speed, posted speed, lighting, number of vehicles per hour, number of crossing facilities, maximum and average degree of horizontal curves and proportion of trucks. The following factors are accountable for causing traffic accidents namely: geometric actors: kinds of pedestrian crossings, median width, number of lanes, number of vertical and horizontal curves. Kiran et al. 2017 [67] reviewed the accident systems at selected crossings of an urban arterial in Dhaka. The study revealed around 40 percent of total accidents in the Metro Politian city of Dhaka with intersection accidents. They studied the major causes and types of accidents to identify the problem. They advised suitable countermeasures to decrease such accidents. Major studies concentrated on using Poisson and negative binomial regression models which include traffic flow, segment length, and access roads, road environment and segment distance [67].
4.3. Crash Prediction Models Based on Speed
Kiran et al., 2017 [67] studied the association between the impact speed and risk of pedestrian casualities in a passenger vehicle collision in China based on real-world accident data. They improved a multiple logistic regression model accepting impact speed and age and observed that the risk of pedestrian fatality is 26% at 50Kmph, 50% at 58Kmph and 82% at 70Kmph. The pedestrians seldom survived at an impact speed of 80Kmph. Survenchen and Fengchen 2010 [68] introducing new variables on ascertaining the accident risk under more dangerous driving conditions and set up more realistic accident criteria. Kiran et al. 2017 [67] reviewed the relevance between speed and accidents on single carriageway roads. Accident data for a defined 5 years period like vehicle speed data, traffic flow data, road characteristics and geometric and layout data were obtained . Generalized linear modeling procedure was used for the study of the data and their correlation. The results of their review revealed that the accident frequency in all categories increased rapidly with mean speed. Therefore, showing that a 10% rise in mean speed results in a 26% rise in the frequency of all injury accidents. A 10% enhancement in means speed would be predicted to result in a 30% rise in the frequency of fatal/dangerous accidents.
4.4. Crash Prediction Models Based on Heavy Vehicles
Guest et al., 2014 [69] made an effort to review age-related safety and other factors which contributed to accidents experienced by heavy vehicle drivers. The author used the Canadian Center for Occupational Health and safety. The author found that heavy vehicle drivers below 27 years of age promoted higher rates of accidents. They opined that both the age groups are at high risk of traffic accidents. Sravani et al. 2011 [70] recognized high-risk sites for large truck crashes in Arizona and tested potential risk factors belonging to the design and high-risk site. They identified high-risk sites using negative binomial regression and property damage only equivalents methods. Kiran et al. 2017 [67] reviewed a study that assessed the number of fatalities and serious injuries for heavy vehicle drivers in Western Australia involved in a crash from hospitals police records. The capture and recapture method was used to estimate the similarities and differences in the characteristics of heavy vehicle drivers from the sources of police and hospitals. According to the police reports, each heavy vehicle driver involved in a crash was tallied against heavy vehicle driver’s hospital records with data of the crash, age, name and vehicle type. The calculated number of fatalities and serious injuries to heavy vehicle drivers from 1st July 1999 to 31st December 2000 was 5 and 59, respectively.Drivers aging between 37 and 40 years were found significant in number (P>0.05) in the heavy vehicle crashes. However, female heavy vehicle drivers were over reflected in hospital records when compared to the records of the police.
4.5. Crash Prediction Models Based on Econometric and Social Variables
Lovegrove et al. 2010 [64] built up a community-based, micro level collision prediction model (CPM) used with a regional transportation plan. They extracted data from Columbia, Canada, British and greater Vancouver involving output from the regional transportation model. They observed that volume capacity was the dominant variable. Yannis et al. 2010 [54] conducted a statistical investigation on Greek drivers’ attitude with data taken within the scope of an extensive survey from 23 European countries.
Clarke 2010 [71] studied a sample of 2000 crashes involving drivers aged 60 years considered from the UK midland police forces in the years 1994-2007. Every case was summarized as a narrative with a sketch plan and a list of explanatory factors. It was fundamentally noticed that elder drivers had problems with intersection collisions. Derogates et al., 2010 [72] used 2003 to 2007 crash data of Ohio, the U.S.A to inquire about the odds of a motorcyclist being fatally injured in a crash and the risk factors involved. The fatality rate was found to be the highest for those who were drug impaired being 15.7%, and 13.8% for alcohol addicts. Night time crashes were susceptible to result in a higher than average fatality rate of 4.8%. Other factors that showed higher than average fatality rate were: bad weather conditions 4.1% and summer season 3.6%. Xiugang Li et al., 2011 [73] assessed accident modification factor for rural frontage road segments in Texas, using Generalized Additive Models (GAM). They analyzed in Texas 123 segments on rural frontage roads and noticed that GAM showed a nonlinear relationship between crash risk and changes in lane and shoulder widths for frontage roads. They extracted crash data for the years 1997-2001 from Texas public safety department [58, 74].
CONCLUSION
Based on the above review conducted on traffic accidents and related practices, the following conclusions can be drawn accordingly:
- Traffic accidents have massively grown recently and are classified as the third cause of predicted deaths in 2020. Traffic accidents are threating the life of the public and the economy of the countries. Thus, researches have made lots of attempts to study and address the traffic accidents to reduce the increasing number of accidents and to alleviate their hazardous consequences on society.
- The countries investigated in this article were developing countries classified as the most dangerous in terms of the number of traffic accidents. Traffic accidents are related to a number of attributes like poor planning system, low standards of safety, lack of public consciousness. Thus, future studies should cautiously address and control traffic accidents.
- Crash prediction models (CPMs) were used to estimate the crash frequency on-road sections related to the traffic flow, road length, and risk factors like cross-sectional design elements. These models can be used for identifying relatively unsafe road sections in a network and for estimating the effects of the implementation of safety measures on a road section.
- Prospectus traffic policy should enhance the fundamental standards for good road design and traffic management, improve vehicle standards, control speed, and promote the use of seat belts and helmets, and the enforcement of alcohol limits. Current endeavours to address the problems of road safety were compared with what has been achieved. While there are many interventions that can save lives, political will and commitment at the central state and local government levels are essential. Road users in worldwide deserve better and safer road to travel.
- Current studies have used old softwares to address traffic crashes. However, in future, traffic accidents should be controlled using advanced artificial intelligence software because of the efficiency of such programmes to control and decrease traffic accidents.
- Safety standards should be adopted in Iraq such as strategies focusing on four key factors: exposure, behavioral factors, road environment, and vehicle factors.
CONSENT FOR PUBLICATION
Not Applicable
FUNDING
None.
CONFLICT OF INTEREST
The author declares no conflict of interest, financial or otherwise.
ACKNOWLEDGEMENTS
Declared none.